Sufficient Dimension Reduction For Visual Sequence Classification
2010 IEEE CONFERENCE ON COMPUTER VISION AND PATTERN RECOGNITION (CVPR)(2010)
摘要
When classifying high-dimensional sequence data, traditional methods (e.g., HMMs, CRFs) may require large amounts of training data to avoid overfitting. In such cases dimensionality reduction can be employed to find a low-dimensional representation on which classification can be done more efficiently. Existing methods for supervised dimensionality reduction often presume that the data is densely sampled so that a neighborhood graph structure can be formed, or that the data arises from a known distribution. Sufficient dimension reduction techniques aim to find a low dimensional representation such that the remaining degrees of freedom become conditionally independent of the output values. In this paper we develop a novel sequence kernel dimension reduction approach (S-KDR). Our approach does not make strong assumptions on the distribution of the input data. Spatial, temporal and periodic information is combined in a principled manner, and an optimal manifold is learned for the end-task. We demonstrate the effectiveness of our approach on several tasks involving the discrimination of human gesture and motion categories, as well as on a database of dynamic textures.
更多查看译文
关键词
spatial information,databases,degree of freedom,computer vision,feature extraction,graph theory,dynamics,image classification,dimension reduction,training data,principal component analysis,gaussian processes,unsupervised learning,kernel,support vector machines,linear discriminant analysis,conditional independence,artificial neural networks,application software
AI 理解论文
溯源树
样例
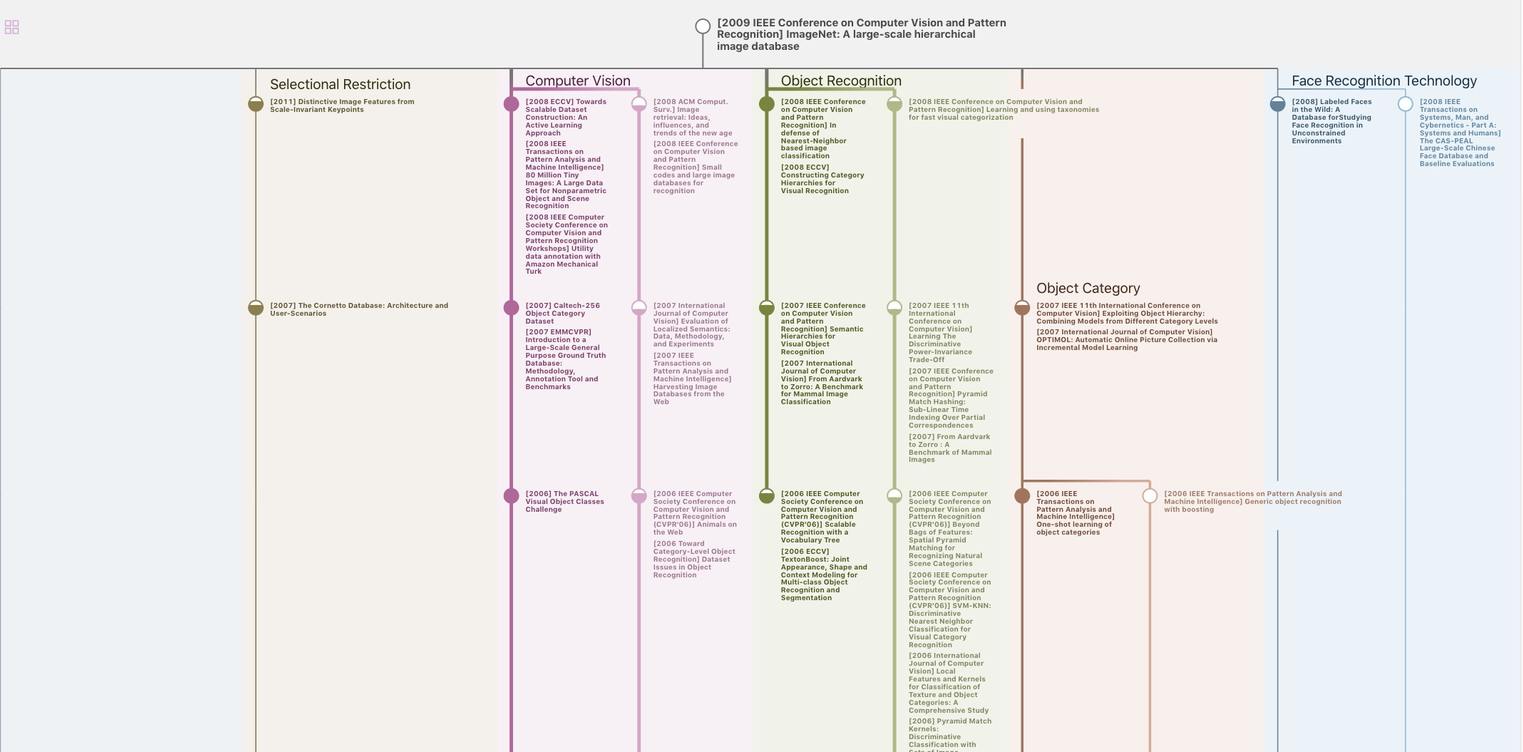
生成溯源树,研究论文发展脉络
Chat Paper
正在生成论文摘要