A Deep Learning Methodology To Proliferate Golden Signoff Timing
DATE '14: Proceedings of the conference on Design, Automation & Test in Europe(2014)
摘要
Signoff timing analysis remains a critical element in the IC design flow. Multiple signoff corners, libraries, design methodologies, and implementation flows make timing closure very complex at advanced technology nodes. Design teams often wish to ensure that one tool's timing reports are neither optimistic nor pessimistic with respect to another tool's reports. The resulting "correlation" problem is highly complex because tools contain millions of lines of black-box and legacy code, licenses prevent any reverse-engineering of algorithms, and the nature of the problem is seemingly "unbounded" across possible designs, timing paths, and electrical parameters.In this work, we apply a "big-data" approach to the timer correlation problem. We develop a machine learning-based tool, Golden Timer eXtension (GTX), to correct divergence in flip-flop setup time, cell arc delay, wire delay, stage delay, and path slack at timing endpoints between timers. We propose a methodology to apply GTX to two arbitrary timers, and we evaluate scalability of GTX across multiple designs and foundry technologies /libraries, both with and without signal integrity analysis. Our experimental results show reduction in divergence between timing tools from 139.3ps to 21.1ps (i.e., 6.6x) in endpoint slack, and from 117ps to 23.8ps (4.9x reduction) in stage delay. We further demonstrate the incremental application of our methods so that models can be adapted to any outlier discrepancies when new designs are taped out in the same technology /library. Last, we demonstrate that GTX can also correlate timing reports between signoff and design implementation tools.
更多查看译文
关键词
Big Data,delays,flip-flops,learning (artificial intelligence),Big-Data approach,GTX,IC design flow,advanced technology nodes,cell arc delay,deep learning methodology,electrical parameters,endpoint slack,flip-flop setup time,foundry technology-libraries,golden signoff timing analysis,golden timer extension,machine learning-based tool,outlier discrepancies,path slack,signal integrity analysis,stage delay,time 139.3 ps to 21.1 ps,timer correlation problem,timing paths,wire delay,
AI 理解论文
溯源树
样例
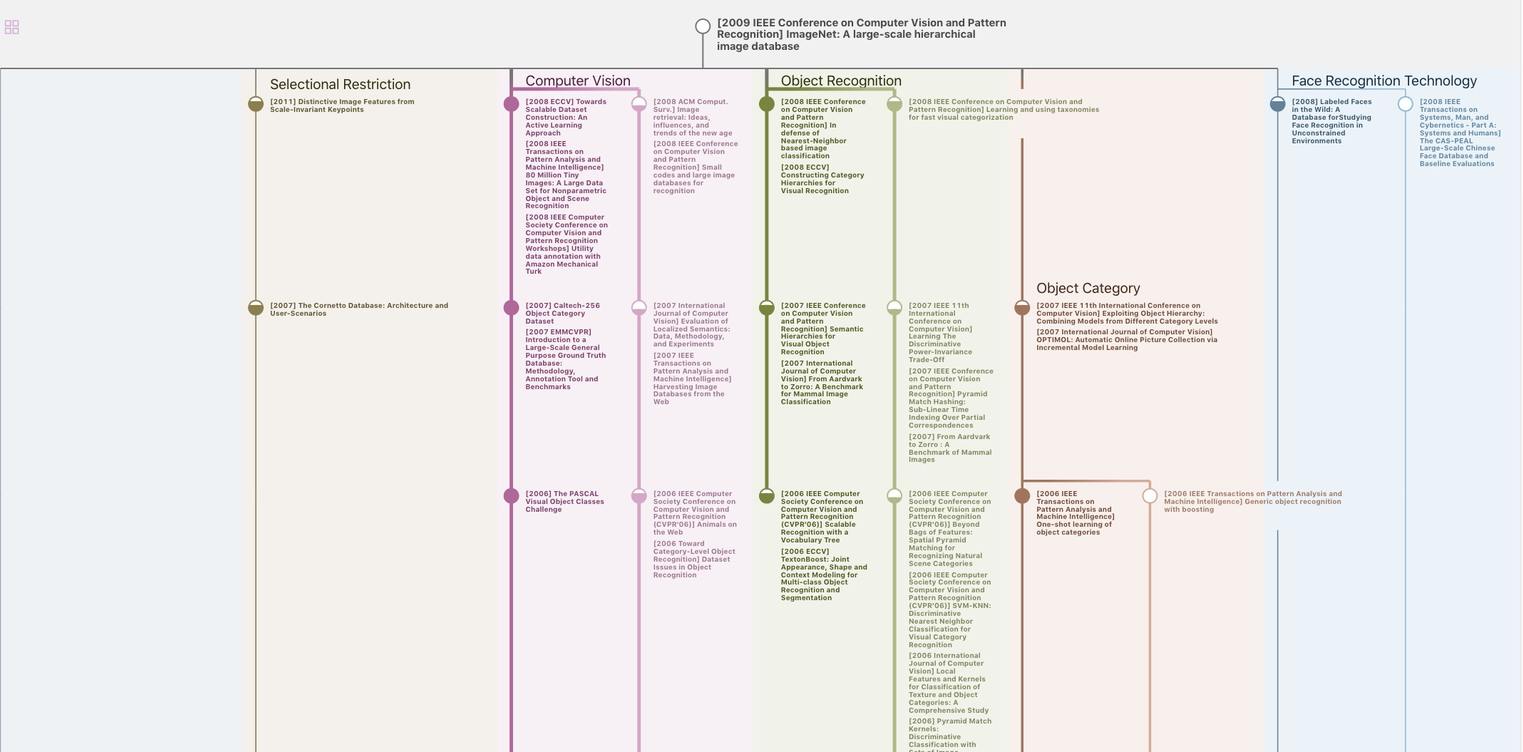
生成溯源树,研究论文发展脉络
Chat Paper
正在生成论文摘要