Focal Plane Array Folding For Efficient Information Extraction And Tracking
AIPR(2012)
摘要
We develop a novel compressive sensing based approach for detecting point sources in images and tracking of moving point sources across temporal images. One application is the muzzle flash detection and tracking problem. We pursue the concept of lower-dimension signal representation from structured sparse matrices, which is in contrast to the use of random sparse matrices described in common compressive sensing algorithms. The primary motivation is that an approach using structured sparse matrices can lead to efficient hardware implementations and a scheme that we term folding in the focal plane array. This method "bins" pixels modulo a pair of specified numbers across the pixel plane in both the horizontal and vertical directions. Under this paradigm, a significant reduction in the amount of pixel samples is required, which enable high speed target acquisition and tracking while reducing the number of A/D's. Folding is used to acquire a pair of significantly smaller images, in which two different folded images provide the necessary redundancy to uniquely extract location information. We detect the centroid of point sources in each of the two folded images and use the Chinese remainder theorem (CRT) to determine the location of the point sources in the original image. In our work, we successfully demonstrated the correctness of this algorithm through simulation and showed the algorithm is capable of detecting and tracking multiple muzzle flashes in multiple temporal frames. We present both initial results and improvements to the algorithm's robustness, based on robust Chinese remainder theorem (rCRT) in the presence of noise.
更多查看译文
关键词
multiple muzzle flash,location information,sparse matrix,focal plane array,focal plane array folding,chinese remainder theorem,point source,structured sparse matrix,multiple temporal frame,common compressive,efficient information extraction,random sparse,feature extraction,object tracking,sparse matrices,compressed sensing
AI 理解论文
溯源树
样例
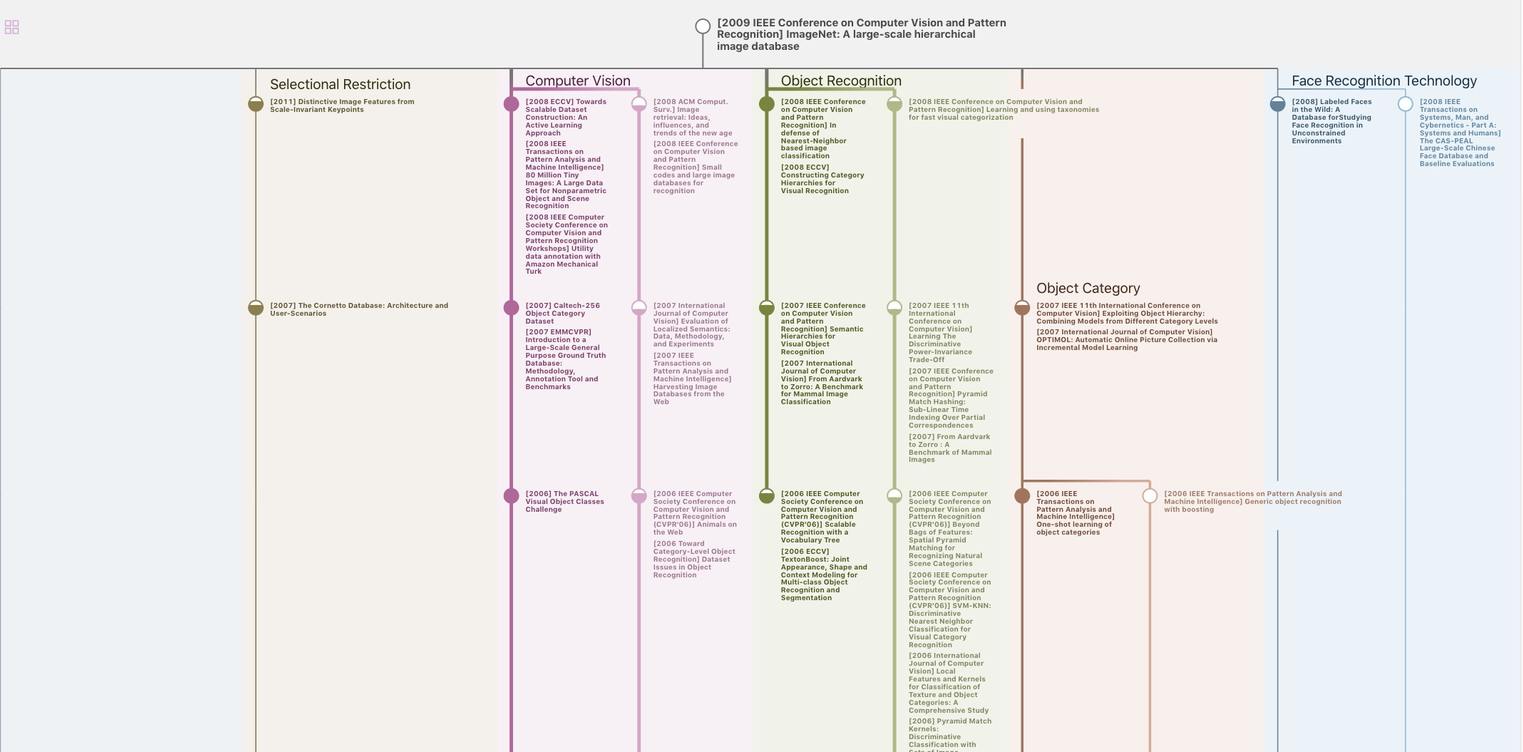
生成溯源树,研究论文发展脉络
Chat Paper
正在生成论文摘要