Text Clustering with Local Semantic Kernels
SURVEY OF TEXT MINING II: CLUSTERING, CLASSIFICATION, AND RETRIEVAL(2008)
摘要
Document clustering is a fundamental task of text mining, by which efficient organization, navigation, summarization, and retrieval of documents can be achieved. The clustering of documents presents difficult challenges due to the sparsity and the high dimensionality of text data, and to the complex semantics of natural language. Subspace clustering is an extension of traditional clustering that is designed to capture local feature relevance, and to group documents with respect to the features (or words) that matter the most. This chapter presents a subspace clustering technique based on a locally adaptive clustering (LAC) algorithm. To improve the subspace clustering of documents and the identification of keywords achieved by LAC, kernel methods and semantic distances are deployed. The basic idea is to define a local kernel for each cluster by which semantic distances between pairs of words are computed to derive the clustering and local term weightings. The proposed approach, called semantic LAC, is evaluated using benchmark datasets. Our experiments show that semantic LAC is capable of improving the clustering quality.
更多查看译文
AI 理解论文
溯源树
样例
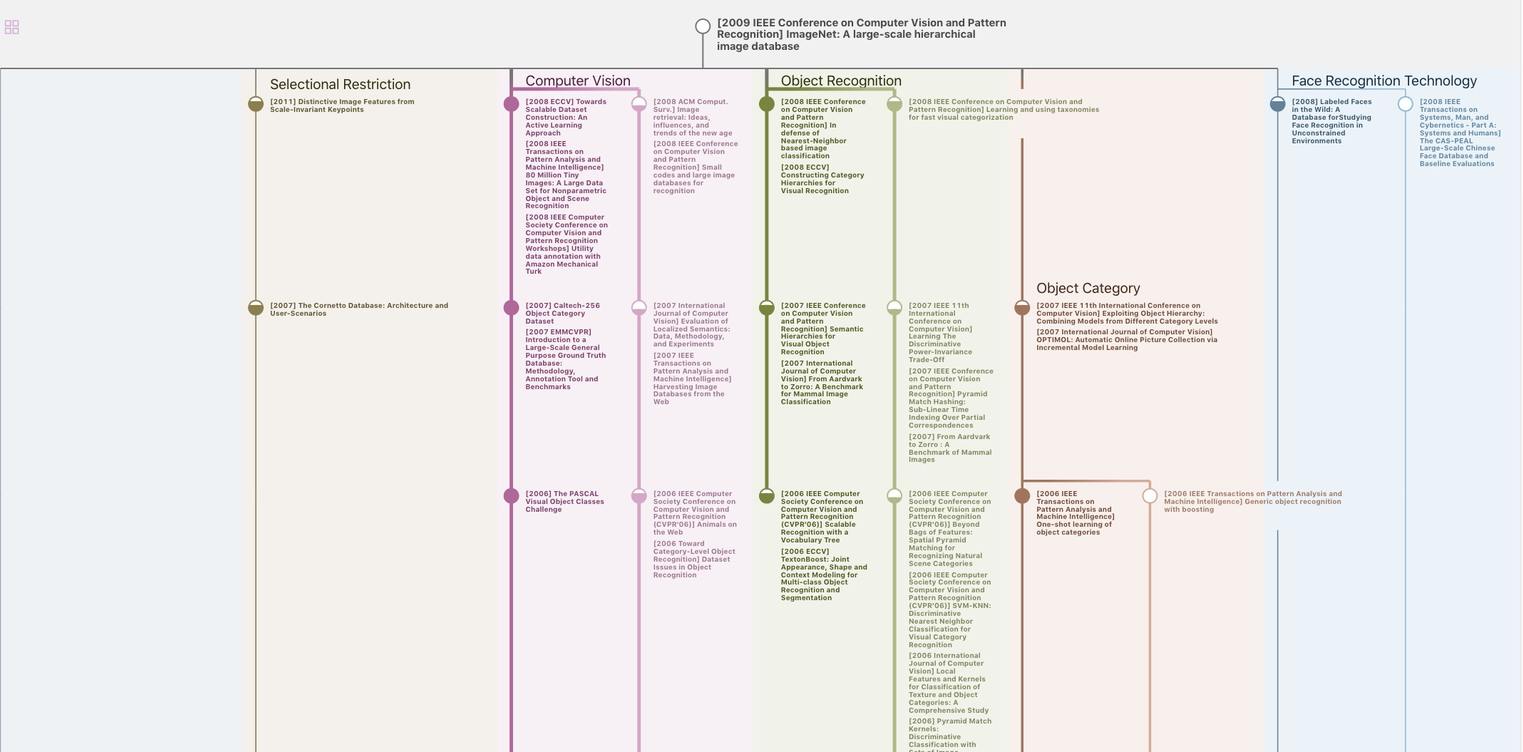
生成溯源树,研究论文发展脉络
Chat Paper
正在生成论文摘要