Exploring Label Dependency in Active Learning for Phenotype Mapping.
BioNLP '12: Proceedings of the 2012 Workshop on Biomedical Natural Language Processing(2012)
摘要
Many genetic epidemiological studies of human diseases have multiple variables related to any given phenotype, resulting from different definitions and multiple measurements or subsets of data. Manually mapping and harmonizing these phenotypes is a time-consuming process that may still miss the most appropriate variables. Previously, a supervised learning algorithm was proposed for this problem. That algorithm learns to determine whether a pair of phenotypes is in the same class. Though that algorithm accomplished satisfying F-scores, the need to manually label training examples becomes a bottleneck to improve its coverage. Herein we present a novel active learning solution to solve this challenging phenotype-mapping problem. Active learning will make phenotype mapping more efficient and improve its accuracy.
更多查看译文
关键词
active learning,novel active learning solution,supervised learning algorithm,challenging phenotype-mapping problem,multiple measurement,multiple variable,phenotype mapping,appropriate variable,different definition,genetic epidemiological study,Exploring label dependency
AI 理解论文
溯源树
样例
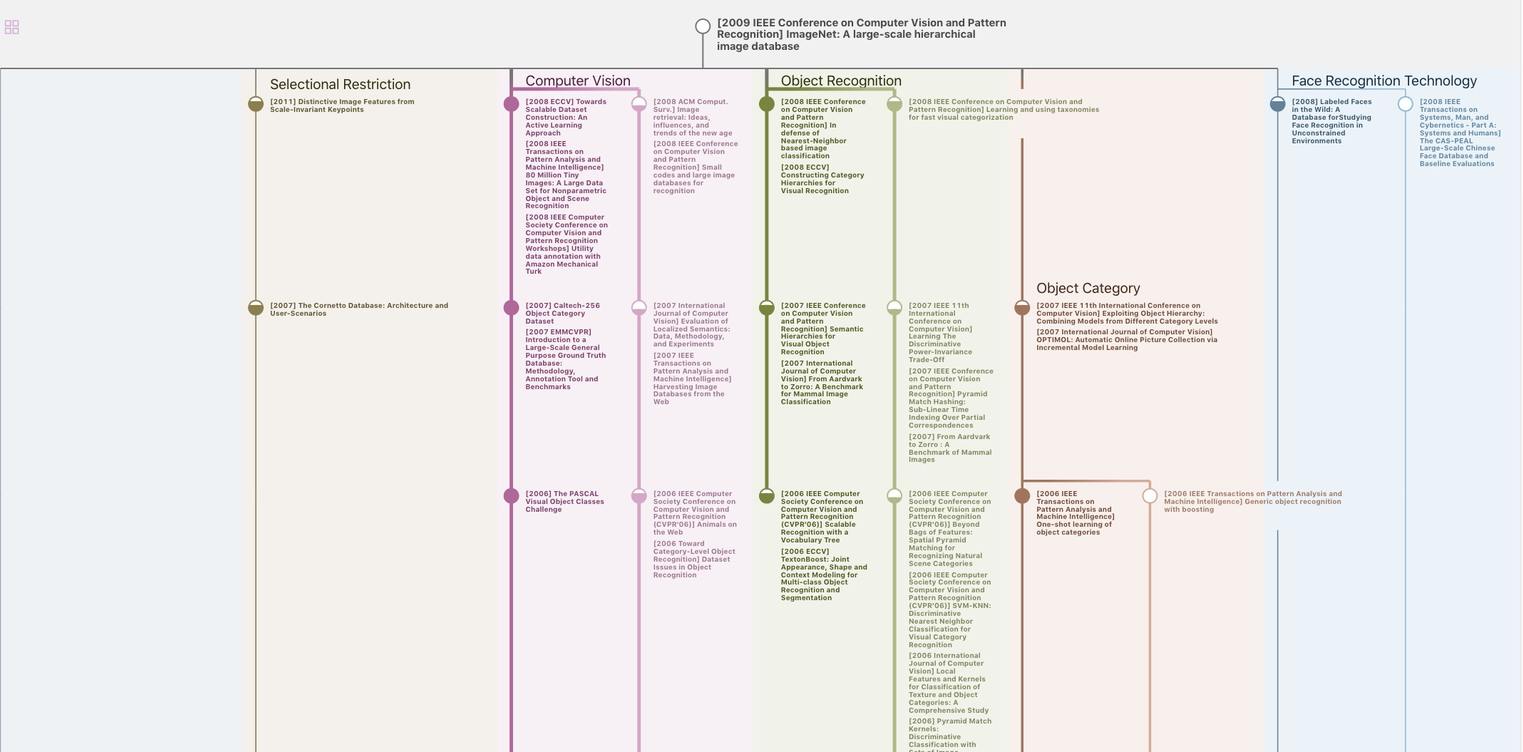
生成溯源树,研究论文发展脉络
Chat Paper
正在生成论文摘要