Efficient Gradient-Based Inference through Transformations between Bayes Nets and Neural Nets
ICML'14: Proceedings of the 31st International Conference on International Conference on Machine Learning - Volume 32(2015)
摘要
Hierarchical Bayesian networks and neural networks with stochastic hidden units are commonly perceived as two separate types of models. We show that either of these types of models can often be transformed into an instance of the other, by switching between centered and differentiable non-centered parameterizations of the latent variables. The choice of parameterization greatly influences the efficiency of gradient-based posterior inference; we show that they are often complementary to eachother, we clarify when each parameterization is preferred and show how inference can be made robust. In the non-centered form, a simple Monte Carlo estimator of the marginal likelihood can be used for learning the parameters. Theoretical results are supported by experiments.
更多查看译文
关键词
neural nets,bayes nets,inference,transformations,gradient-based
AI 理解论文
溯源树
样例
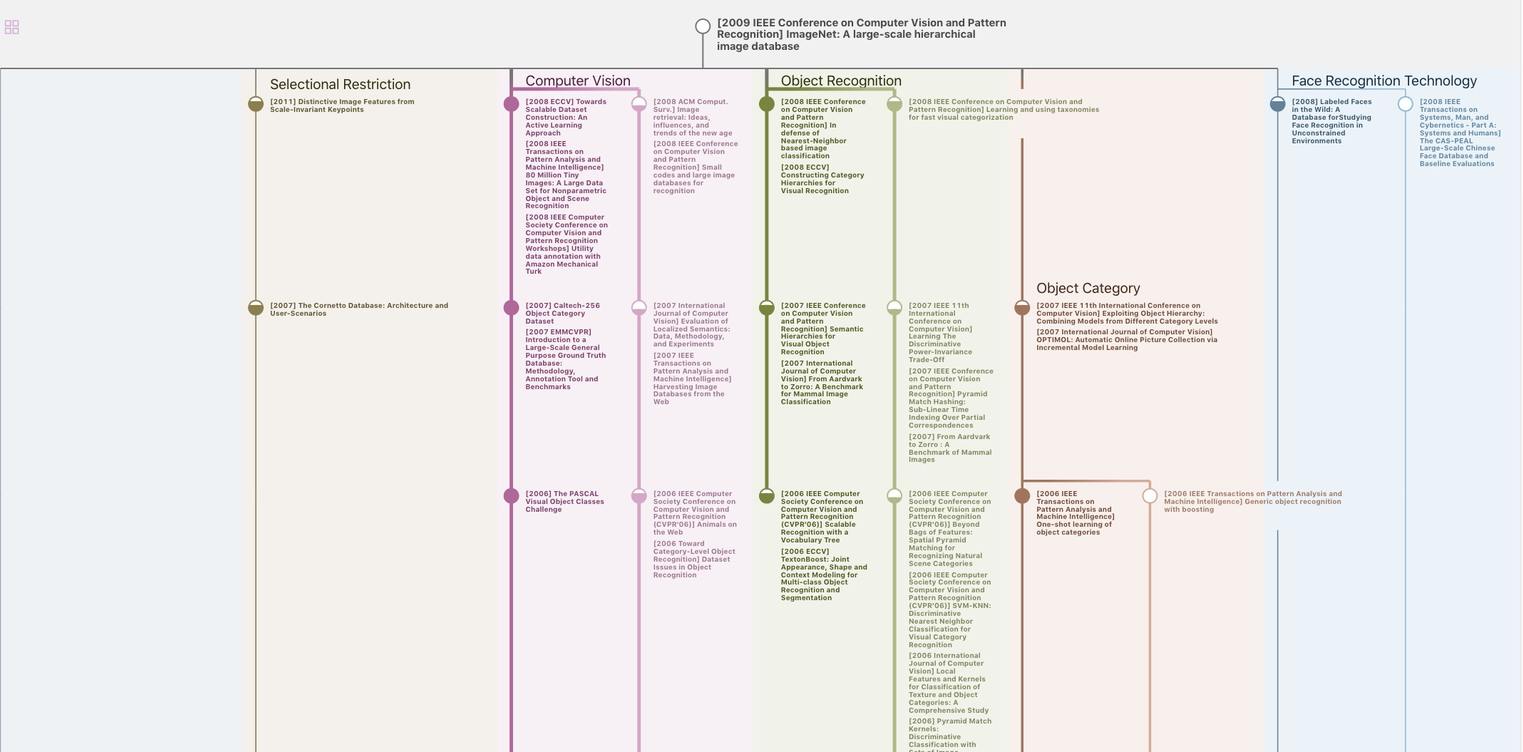
生成溯源树,研究论文发展脉络
Chat Paper
正在生成论文摘要