The Role of Semantic History on Online Generative Topic Modeling
msra(2009)
摘要
Online processing of text streams is an essential task of many genuine applications. The objective is to identify the underlying structure of evolving themes in the incoming streams online at the time of their arrival. As many topics tend to reappear consistently in text streams, incorporating semantics that were discovered in previous streams would eventually enhance the identification and description of topics in the future. Latent Dirichlet Allocation (LDA) topic model is a probabilistic technique that has been successfully used to automatically extract the topical or semantic content of documents. In this paper, we investigate the role of past semantics in estimating future topics under the framework of LDA topic modeling, based on the online version implemented in (1). The idea is to construct the current model based on information propagated from topic models that fall within a "sliding history window". Then, this model is incrementally updated according to the information inferred from the new stream of data with no need to access previous data. Since the proposed approach is totally unsupervised and data-driven, we analyze the effect of different factors that are involved in this model, including the window size, history weight, and equal/decaying history contribution. The proposed approach is evaluated using benchmark datasets. Our experiments show that the embedded semantics from the past improved the quality of the document modeling. We also found that the role of history varies according to the domain and nature of text data.
更多查看译文
AI 理解论文
溯源树
样例
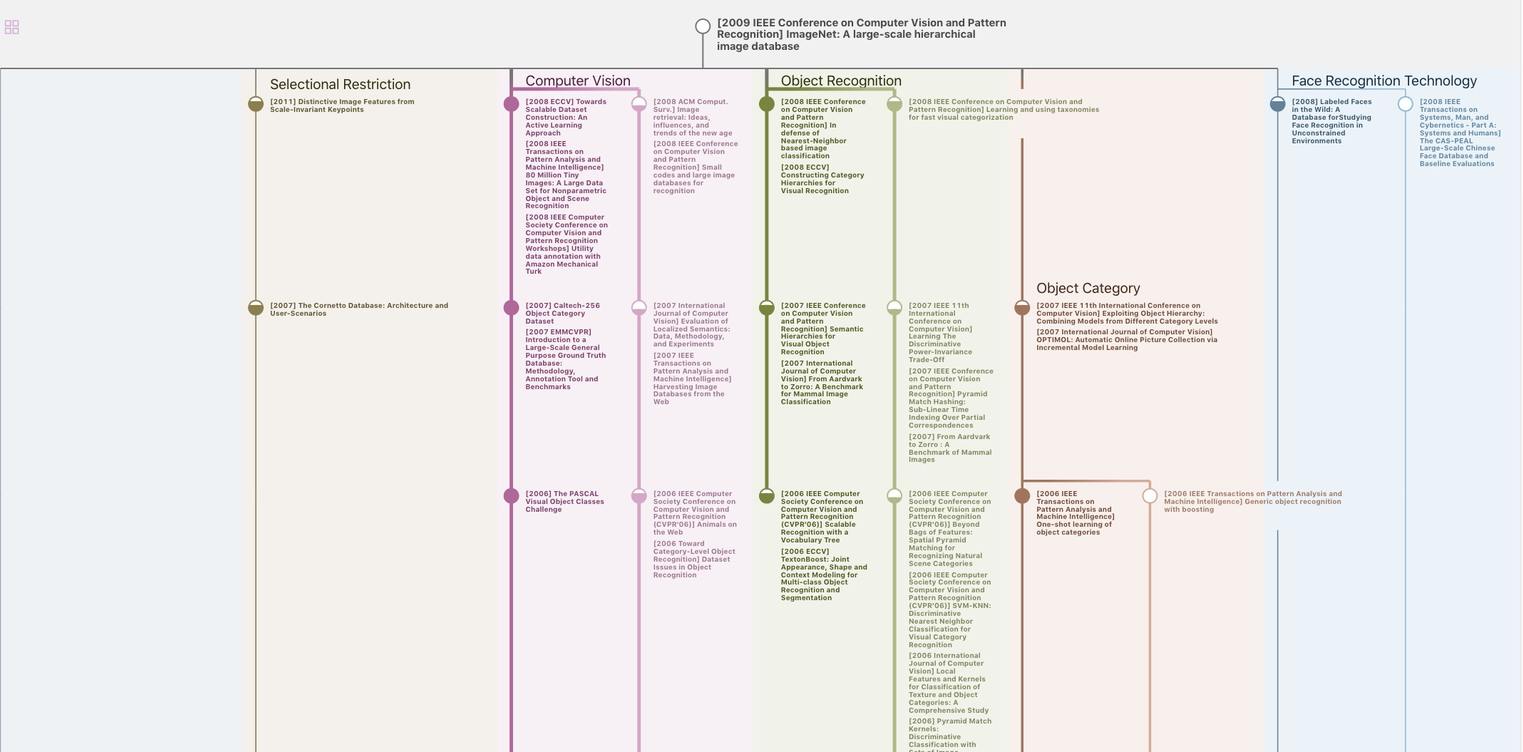
生成溯源树,研究论文发展脉络
Chat Paper
正在生成论文摘要