Influence Blocking Maximization in Social Networks under the Competitive Linear Threshold Model Technical Report
CoRR(2011)
摘要
In many real-world situations, different and often opposite opinions, innovations, or products are competing with one another for their social influence in a networked society. In this paper, we study competitive influence propagation in social networks under the competitive linear threshold (CLT) model, an extension to the classic linear threshold model. Under the CLT model, we focus on the problem that one entity tries to block the influence propagation of its competing entity as much as possible by strategically selecting a number of seed nodes that could initiate its own influence propagation. We call this problem the influence blocking maximization (IBM) problem. We prove that the objective function of IBM in the CLT model is submodular, and thus a greedy algorithm could achieve 1-1/e approximation ratio. However, the greedy algorithm requires Monte-Carlo simulations of competitive influence propagation, which makes the algorithm not efficient. We design an efficient algorithm CLDAG, which utilizes the properties of the CLT model, to address this issue. We conduct extensive simulations of CLDAG, the greedy algorithm, and other baseline algorithms on real-world and synthetic datasets. Our results show that CLDAG is able to provide best accuracy in par with the greedy algorithm and often better than other algorithms, while it is two orders of magnitude faster than the greedy algorithm.
更多查看译文
关键词
objective function,social network,monte carlo simulation,greedy algorithm,threshold model,social influence
AI 理解论文
溯源树
样例
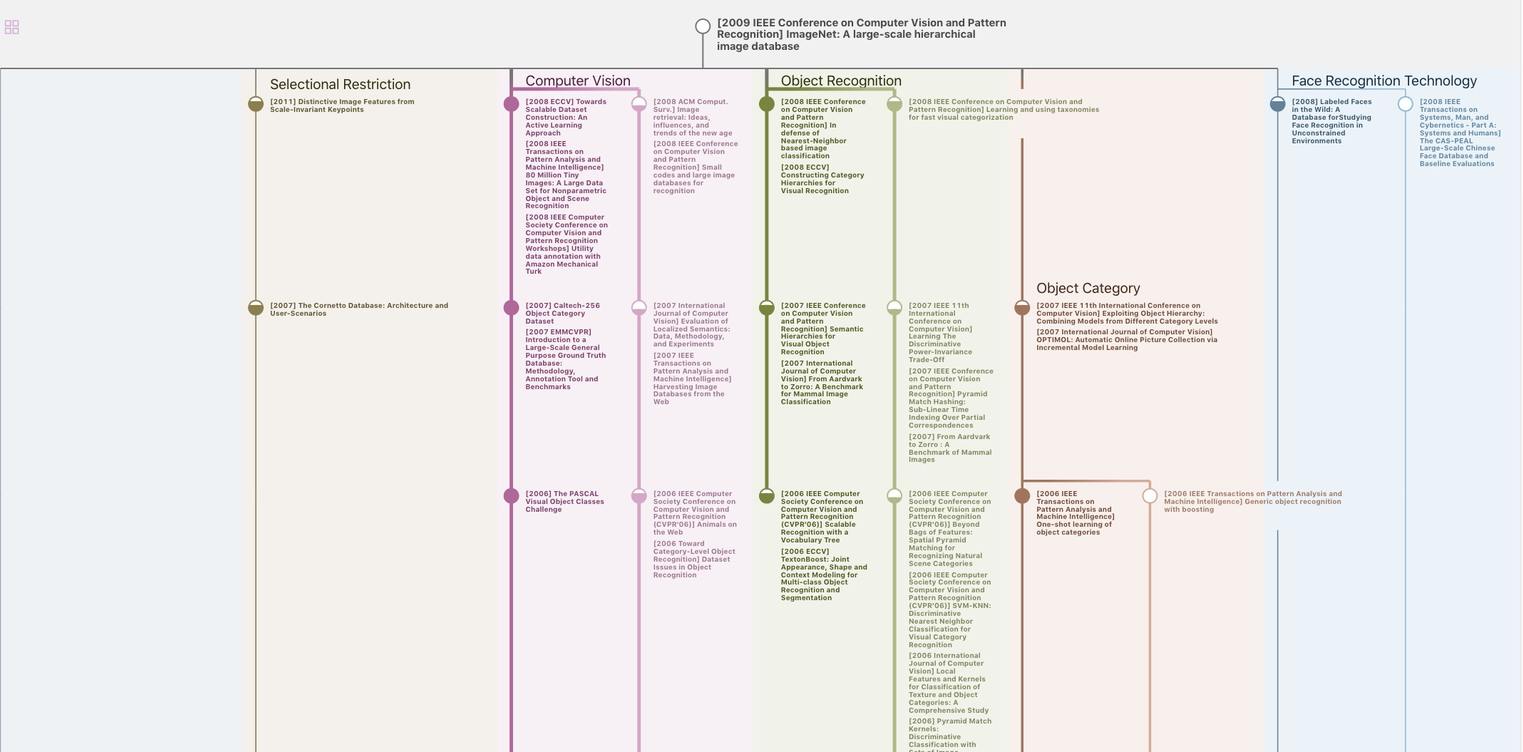
生成溯源树,研究论文发展脉络
Chat Paper
正在生成论文摘要