Voice Conversion For Non-Parallel Datasets Using Dynamic Kernel Partial Least Squares Regression
14TH ANNUAL CONFERENCE OF THE INTERNATIONAL SPEECH COMMUNICATION ASSOCIATION (INTERSPEECH 2013), VOLS 1-5(2013)
摘要
Voice conversion aims at converting speech from one speaker to sound as if it was spoken by another specific speaker. The most popular voice conversion approach based on Gaussian mixture modeling tends to suffer either from model overfitting or over smoothing. To overcome the shortcomings of the traditional approach, we recently proposed to use dynamic kernel partial least squares (DKPLS) regression in the framework of parallel-data voice conversion. However, the availability of parallel training data from both the source and target speaker is not always guaranteed. In this paper, we extend the DKPLS-based conversion approach for non-parallel data by combining it with a well-known INCA alignment algorithm. The listening test results indicate that high-quality conversion can be achieved with the proposed combination. Furthermore, the performance of two variations of INCA are evaluated with both intra-lingual and cross-lingual data.
更多查看译文
关键词
voice conversion,non-parallel data,kernel partial least squares regression,INCA alignment
AI 理解论文
溯源树
样例
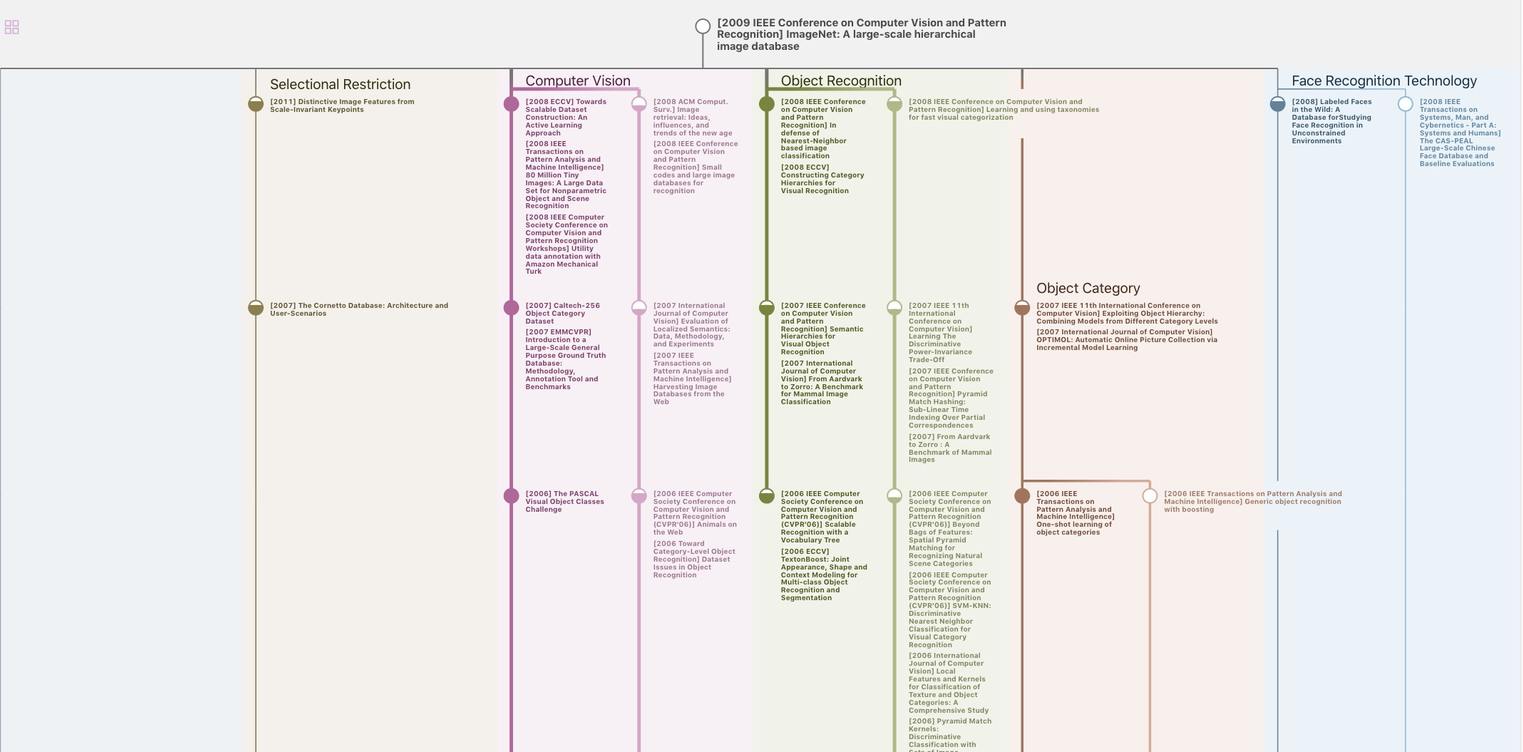
生成溯源树,研究论文发展脉络
Chat Paper
正在生成论文摘要