A survey of large-scale analytical query processing in MapReduce
The VLDB Journal(2013)
摘要
Enterprises today acquire vast volumes of data from different sources and leverage this information by means of data analysis to support effective decision-making and provide new functionality and services. The key requirement of data analytics is scalability, simply due to the immense volume of data that need to be extracted, processed, and analyzed in a timely fashion. Arguably the most popular framework for contemporary large-scale data analytics is MapReduce, mainly due to its salient features that include scalability, fault-tolerance, ease of programming, and flexibility. However, despite its merits, MapReduce has evident performance limitations in miscellaneous analytical tasks, and this has given rise to a significant body of research that aim at improving its efficiency, while maintaining its desirable properties. This survey aims to review the state of the art in improving the performance of parallel query processing using MapReduce. A set of the most significant weaknesses and limitations of MapReduce is discussed at a high level, along with solving techniques. A taxonomy is presented for categorizing existing research on MapReduce improvements according to the specific problem they target. Based on the proposed taxonomy, a classification of existing research is provided focusing on the optimization objective. Concluding, we outline interesting directions for future parallel data processing systems.
更多查看译文
关键词
MapReduce,Survey,Data analysis,Query processing,Large-scale,Big Data
AI 理解论文
溯源树
样例
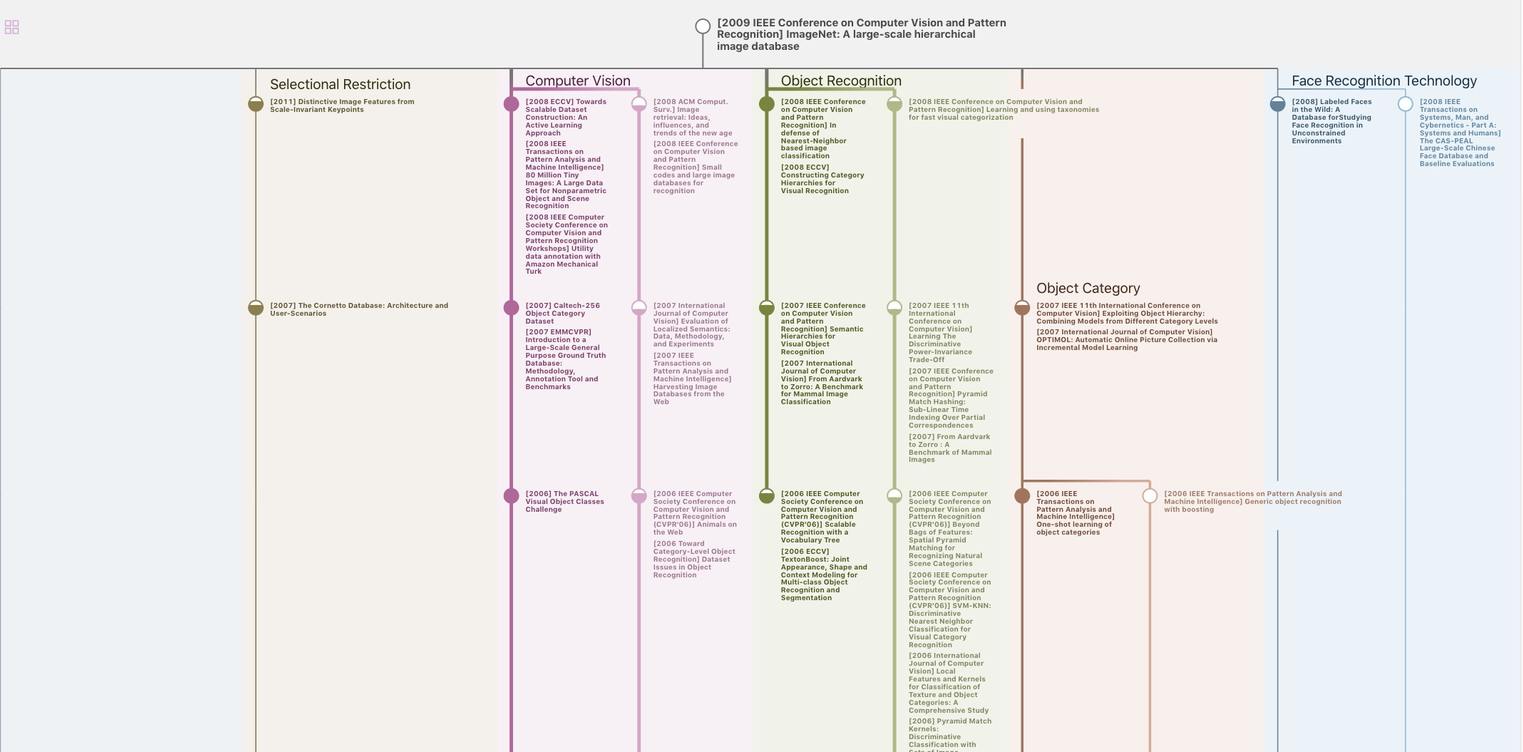
生成溯源树,研究论文发展脉络
Chat Paper
正在生成论文摘要