Variational Bayesian View of Weighted Trace Norm Regularization for Matrix Factorization
IEEE Signal Process. Lett.(2013)
摘要
Matrix factorization with trace norm regularization is a popular approach to matrix completion and collaborative filtering. When entries of the matrix are sampled non-uniformly (which is the case for collaborative prediction), a properly weighted correction to the trace norm regularization is known to improve the performance dramatically. While the weighted trace norm regularization has been rigorously studied, its generative counterpart is not known yet. In this paper we show that the weighted trace norm regularization emerges from variational Bayesian matrix factorization where variational distributions over factor matrices are restricted to be isotropic Gaussians with the common variance. We show that variational variance corresponds to the regularization parameter. Thus, the regularization parameter can be automatically learned by variational inference rather than cross-validation. Experiments on MovieLens and Netflix datasets confirm the variational Bayesian perspective of the weighted trace norm regularization, demonstrating that variational parameter learned by variational inference agrees with the value of the regularization parameter found by cross-validation.
更多查看译文
关键词
variational inference,collaborative prediction,collaborative filtering,bayes methods,trace norm regularization,factor matrices,weighted trace norm regularization,matrix decomposition,matrix factorization,variational distribution,isotropic gaussian,variational bayesian inference,variational bayesian matrix factorization,matrix completion,bayesian methods,materials,computer science,collaboration,covariance matrix
AI 理解论文
溯源树
样例
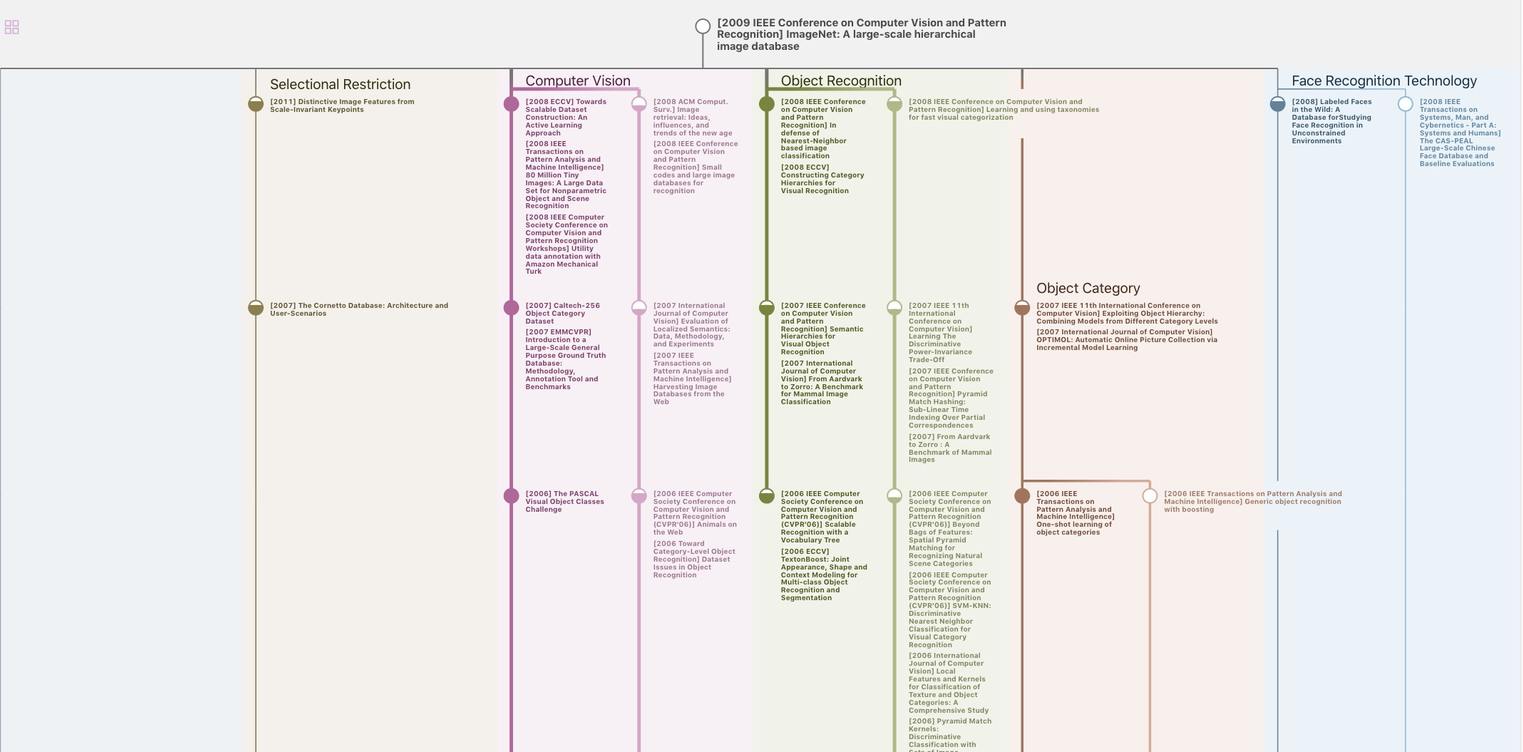
生成溯源树,研究论文发展脉络
Chat Paper
正在生成论文摘要