Supervised vs. Unsupervised Learning for Intentional Process Model Discovery.
Lecture notes in business information processing(2014)
摘要
Learning humans’ behavior from activity logs requires choosing an adequate machine learning technique regarding the situation at hand. This choice impacts significantly results reliability. In this paper, Hidden Markov Models (HMMs) are used to build intentional process models (Maps) from activity logs. Since HMMs parameters require to be learned, the main contribution of this paper is to compare supervised and unsupervised learning approaches of HMMs. After a theoretical comparison of both approaches, they are applied on two controlled experiments to compare the Maps thereby obtained. The results demonstrate using supervised learning leads to a poor performance because it imposes binding conditions in terms of data labeling, introduces inherent humans’ biases, provides unreliable results in the absence of ground truth, etc. Instead, unsupervised learning obtains efficient Maps with a higher performance and lower humans’ effort.
更多查看译文
关键词
process model,unsupervised learning,discovery
AI 理解论文
溯源树
样例
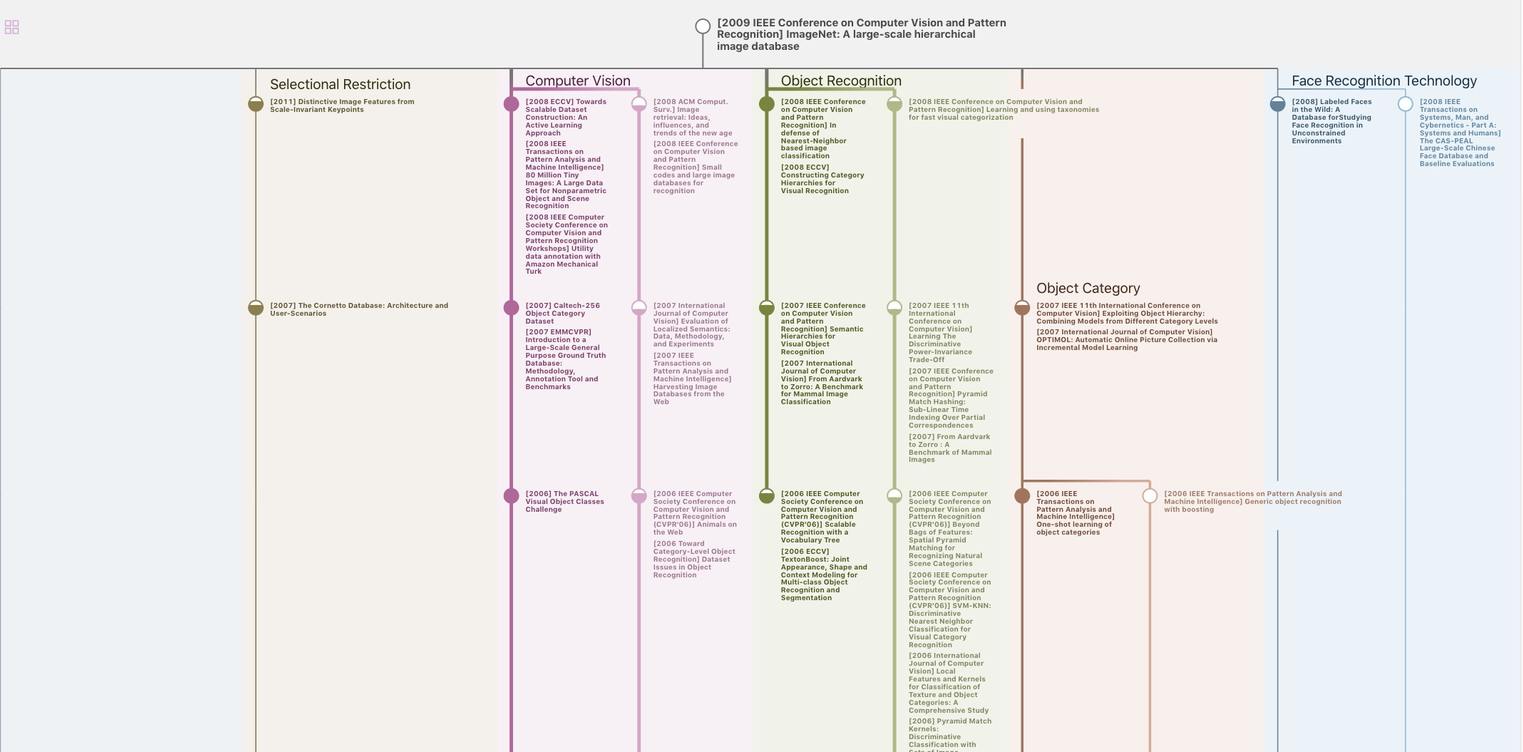
生成溯源树,研究论文发展脉络
Chat Paper
正在生成论文摘要