Weakly Supervised Multi-Graph Learning for Robust Image Reranking
IEEE Transactions on Multimedia(2014)
摘要
Visual reranking has been widely deployed to refine the traditional text-based image retrieval. Its current trend is to combine the retrieval results from various visual features to boost reranking precision and scalability. And its prominent challenge is how to effectively exploit the complementary property of different features. Another significant issue raises from the noisy instances, from manual or automatic labels, which makes the exploration of such complementary property difficult. This paper proposes a novel image reranking by introducing a new Co-Regularized Multi- Graph Learning (Co-RMGL) framework, in which intra-graph and inter-graph constraints are integrated to simultaneously encode the similarity in a single graph and the consistency across multiple graphs. To deal with the noisy instances, weakly supervised learning via co-occurred visual attribute is utilized to select a set of graph anchors to guide multiple graphs alignment and fusion, and to filter out those pseudo labeling instances to highlight the strength of individual features. After that, a learned edge weighting matrix from a fused graph is used to reorder the retrieval results. We evaluate our approach on four popular image retrieval datasets and demonstrate a significant improvement over state-of-the-art methods.
更多查看译文
关键词
Visualization,Labeling,Noise measurement,Robustness,Educational institutions,Semantics,Fuses
AI 理解论文
溯源树
样例
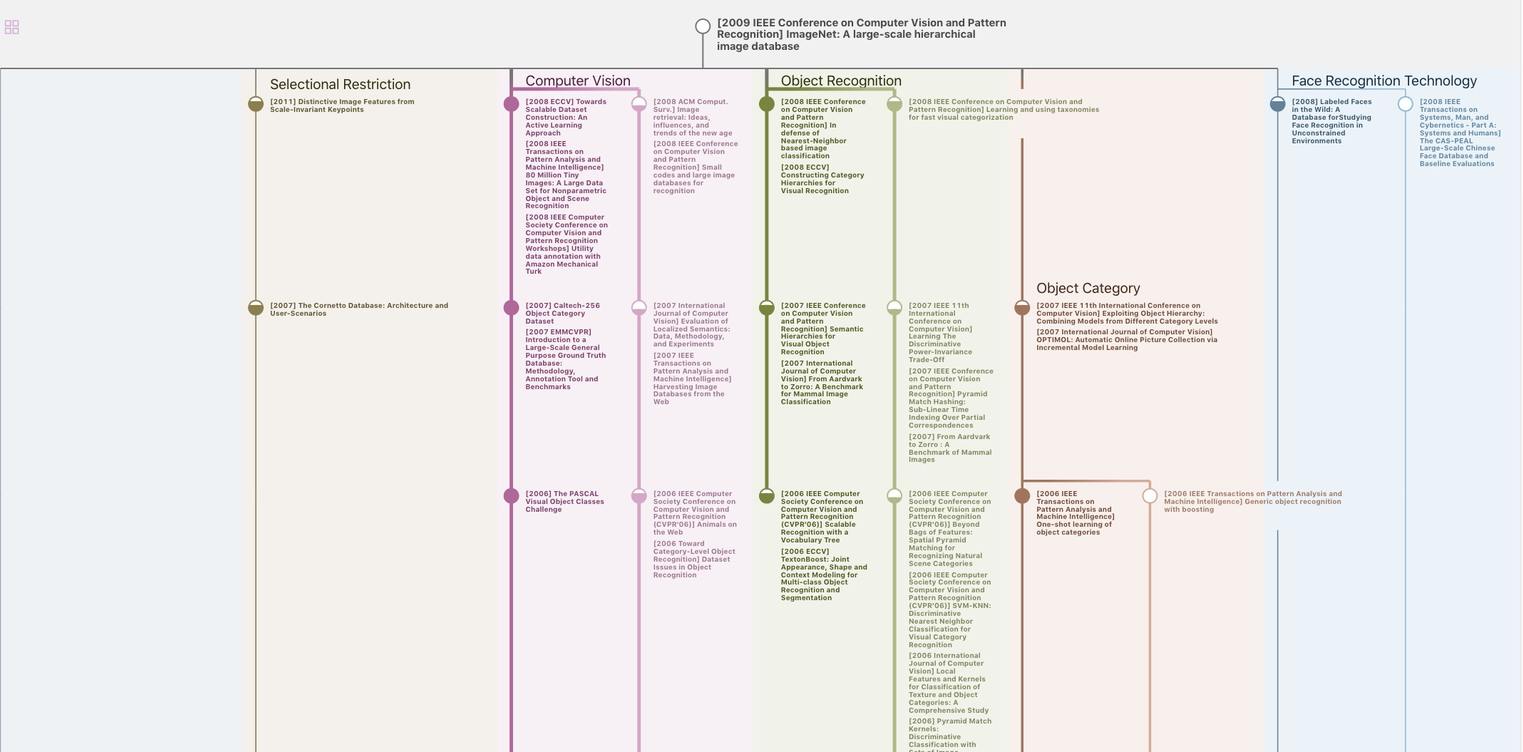
生成溯源树,研究论文发展脉络
Chat Paper
正在生成论文摘要