Segmenting human knee cartilage automatically from multi-contrast MR images using support vector machines and discriminative random fields
ICIP(2011)
摘要
This paper presents a novel solution toward the accurate and automatic cartilage segmentation with multi-contrast MR images based on pixel classification. The previous pixel classification based works for cartilage segmentation only rely on the labeling by a trained classifier, such as support vector machines (SVM) or k-nearest neighbors. However, these frameworks do not consider the spatial information. To incorporate spatial dependencies in pixel classification, we explore a principled framework of pixel classification based on the convex optimization of an SVM-based association potential and a discriminative random fields (DRF) based interaction potential for our task of cartilage segmentation. The local image structure based features as well as the features based on geometrical information are adopted as the features. We finally perform the loopy belief propagation inference algorithm to find the optimal label configuration. Our framework is validated on a dataset of multi-contrast MR images. Experimental results show that the combined features compare favorably to the two types of separate features and our pixel classification framework outperforms the conventional frameworks based solely on SVM or DRF for cartilage segmentation in subject-specific training scenario.
更多查看译文
关键词
k-nearest neighbors,multi-contrast mr images,automatic segmentation,inference mechanisms,trained classifier,pixel classification,image segmentation,image classification,human knee cartilage,biomedical mri,mri,discriminative random fields,cartilage segmentation,cartilage,loopy belief propagation inference algorithm,support vector machines,medical image processing,image structure,sensitivity,spatial information,convex optimization,spatial dependence,support vector machine,magnetic resonance imaging,k nearest neighbor,loopy belief propagation,feature extraction,magnetic resonance image,random field
AI 理解论文
溯源树
样例
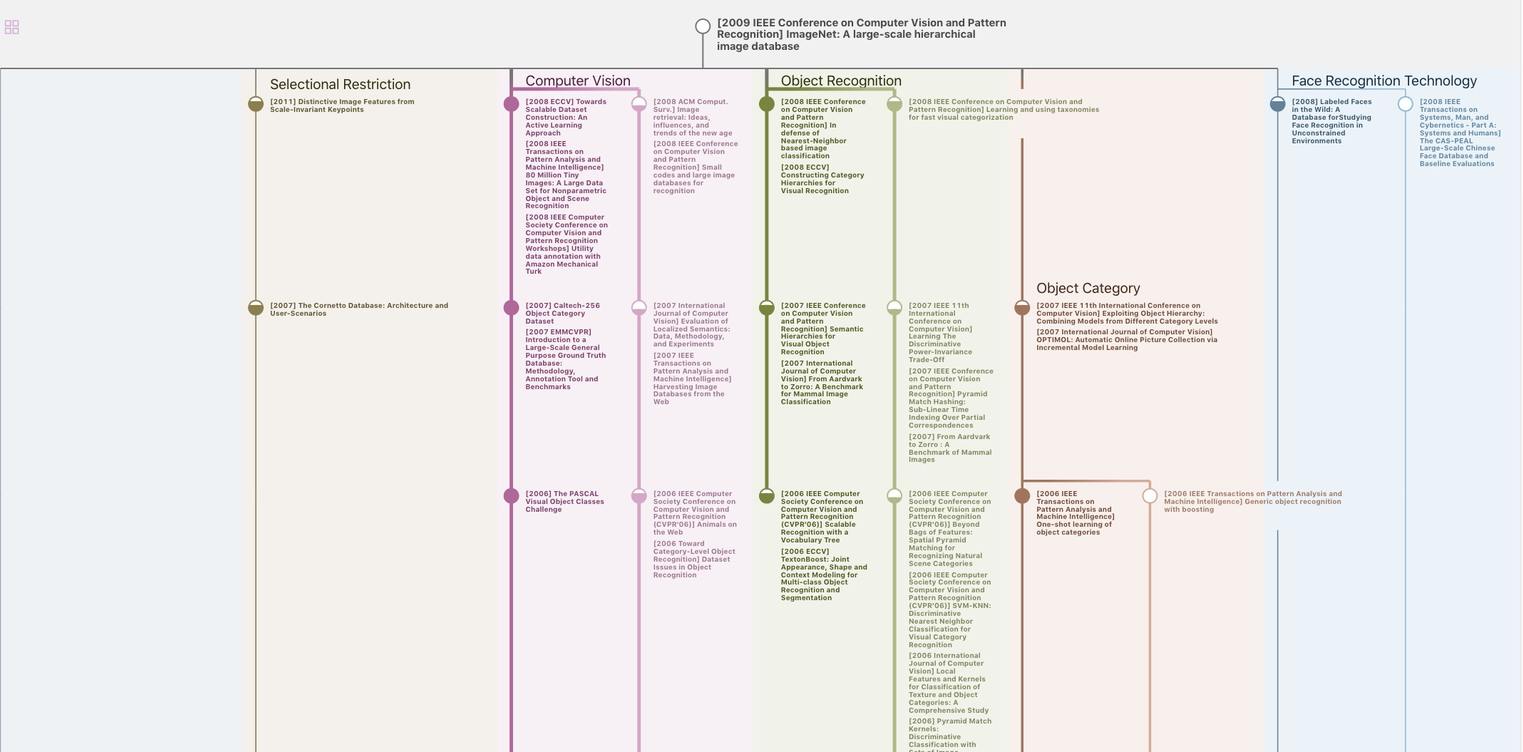
生成溯源树,研究论文发展脉络
Chat Paper
正在生成论文摘要