Cross-domain Text Classification using Wikipedia
IEEE Intelligent Informatics Bulletin(2008)
摘要
Abstract—Traditional approaches,to document,classification requires labeled data in order to construct reliable and accurate classifiers. Unfortunately, labeled data are seldom available, and often too expensive to obtain, especially for large domains and fast evolving scenarios. Given a learning task for which training data are not available, abundant labeled data may exist for a different but related domain. One would,like to use the related labeled data as auxiliary information,to accomplish,the classification task in the target domain. Recently, the paradigm of transfer learning has been introduced,to enable effective learning strategies when auxiliary data obey a different probability distribution. A co-clustering based,classification algorithm,has been previ- ously proposed,to tackle cross-domain,text classification. In this work, we extend the idea underlying this approach by making the latent semantic,relationship between,the two domains,explicit. This goal is achieved with the use of Wikipedia. As a result, the pathway,that allows to propagate,labels between,the two domains not only captures common words, but also semantic concepts based on the content of documents. We empirically demonstrate the efficacy of our,semantic-based,approach,to cross-domain classification using a variety of real data. Index Terms—Text Classification, Wikipedia, Kernel methods,
更多查看译文
关键词
transfer learning,probability distribution,kernel method,indexing terms
AI 理解论文
溯源树
样例
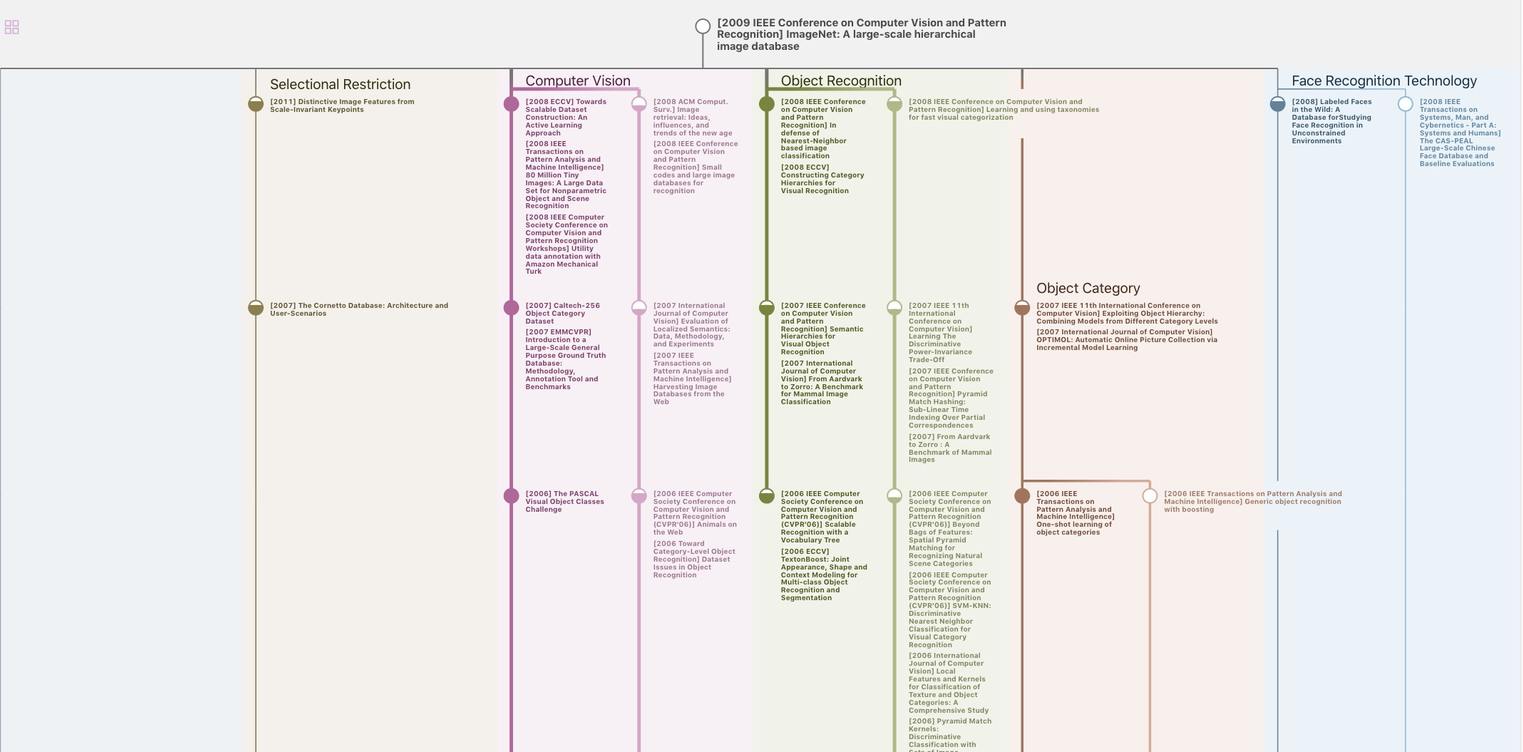
生成溯源树,研究论文发展脉络
Chat Paper
正在生成论文摘要