Toward Optimal Ordering of Prediction Tasks
SDM(2009)
摘要
Many applications involve a set of prediction tasks that must be accomplished sequentially through user interaction. If the tasks are interdependent, the order in which they are performed may have a signicant impact on the overall per- formance of the prediction systems. However, manual speci- cation of an optimal order may be dicult when the inter- dependencies are complex, especially if the number of tasks is large, making exhaustive search intractable. This paper presents the rst attempt at solving the optimal task order- ing problem using an approximate formulation in terms of pairwise task order preferences, reducing the problem to the well-known Linear Ordering Problem. We propose two ap- proaches for inducing the pairwise task order preferences { 1) a classier-agnosti c approach based on conditional entropy that determines the prediction tasks whose correct labels lead to the least uncertainty for the remaining predictions, and 2) a classier-depende nt approach that empirically de- termines which tasks are favored before others for better predictive performance. We apply the proposed solutions to two practical applications that involve computer-assisted trouble report generation and document annotation, respec- tively. In both applications, the user lls up a series of elds and at each step, the system is expected to provide useful suggestions, which comprise the prediction (i.e. classica- tion and ranking) tasks. Our experiments show encouraging improvements in predictive performance, as compared to ap- proaches that do not take task dependencies into account.
更多查看译文
关键词
conditional entropy,exhaustive search
AI 理解论文
溯源树
样例
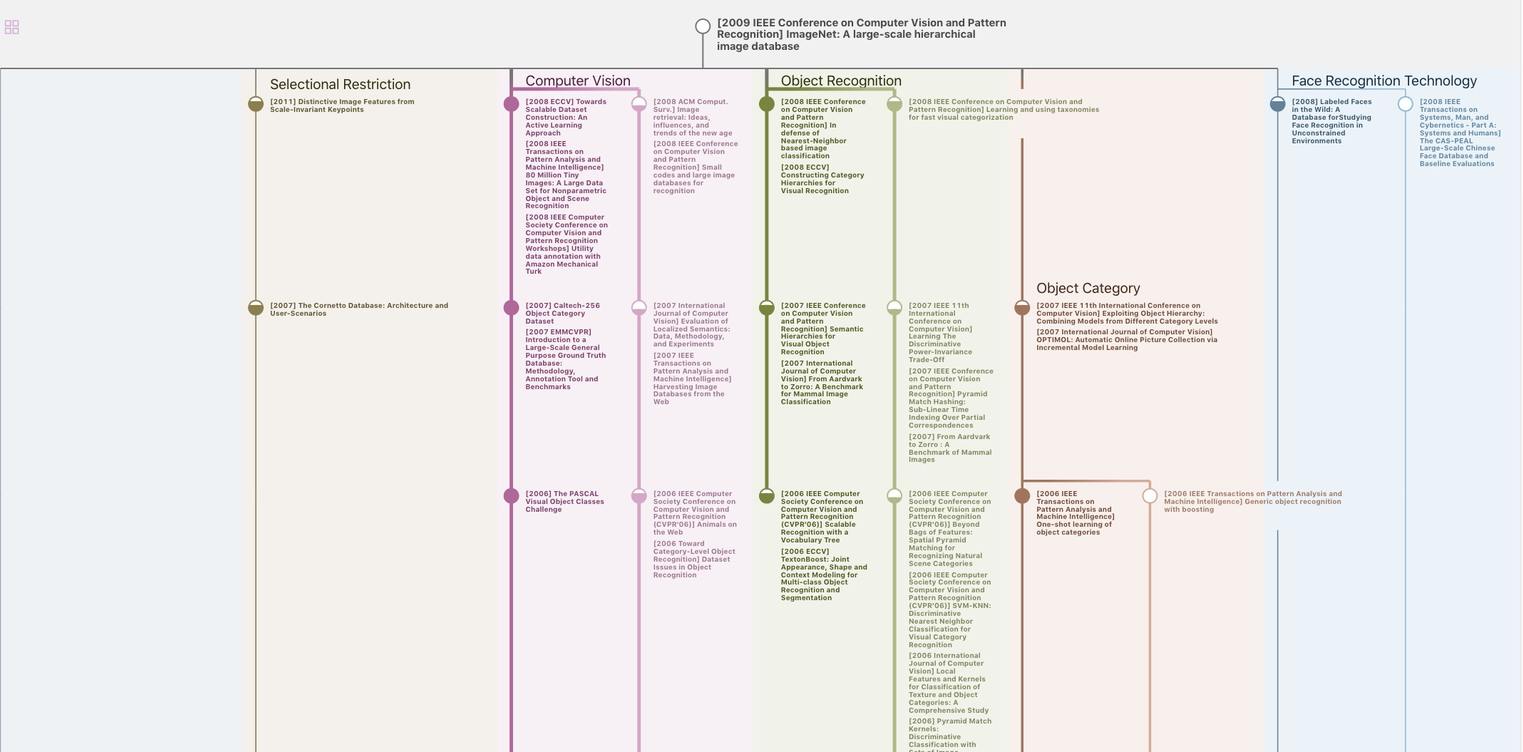
生成溯源树,研究论文发展脉络
Chat Paper
正在生成论文摘要