Music genre classification using multiscale scattering and sparse representations
CISS(2013)
摘要
An effective music genre classication approach is proposed that combines the translation-invariance and deformation-robustness property of scattering coefficients and the discriminative power of sparse representation-based classifiers. We argue that these two approaches to feature selection and classification complement each other in reducing the in-class variability of data, and this should lead to enhanced performance. Our results show clear improvement over a variety of previous approaches. A music genre classication accuracy of approximately 91.2% on the GTZAN database is reported.
更多查看译文
关键词
multiscale scattering representation,gtzan database,music genre classication approach,sparse representation-based classifiers,performance enhancement,in-class data variability reduction,scattering coefficients,sparse matrices,music,pattern classification,translation-invariance property,feature selection approach,feature classification approach,deformation-robustness property,dictionaries,scattering,feature extraction,wavelet transforms,vectors,accuracy
AI 理解论文
溯源树
样例
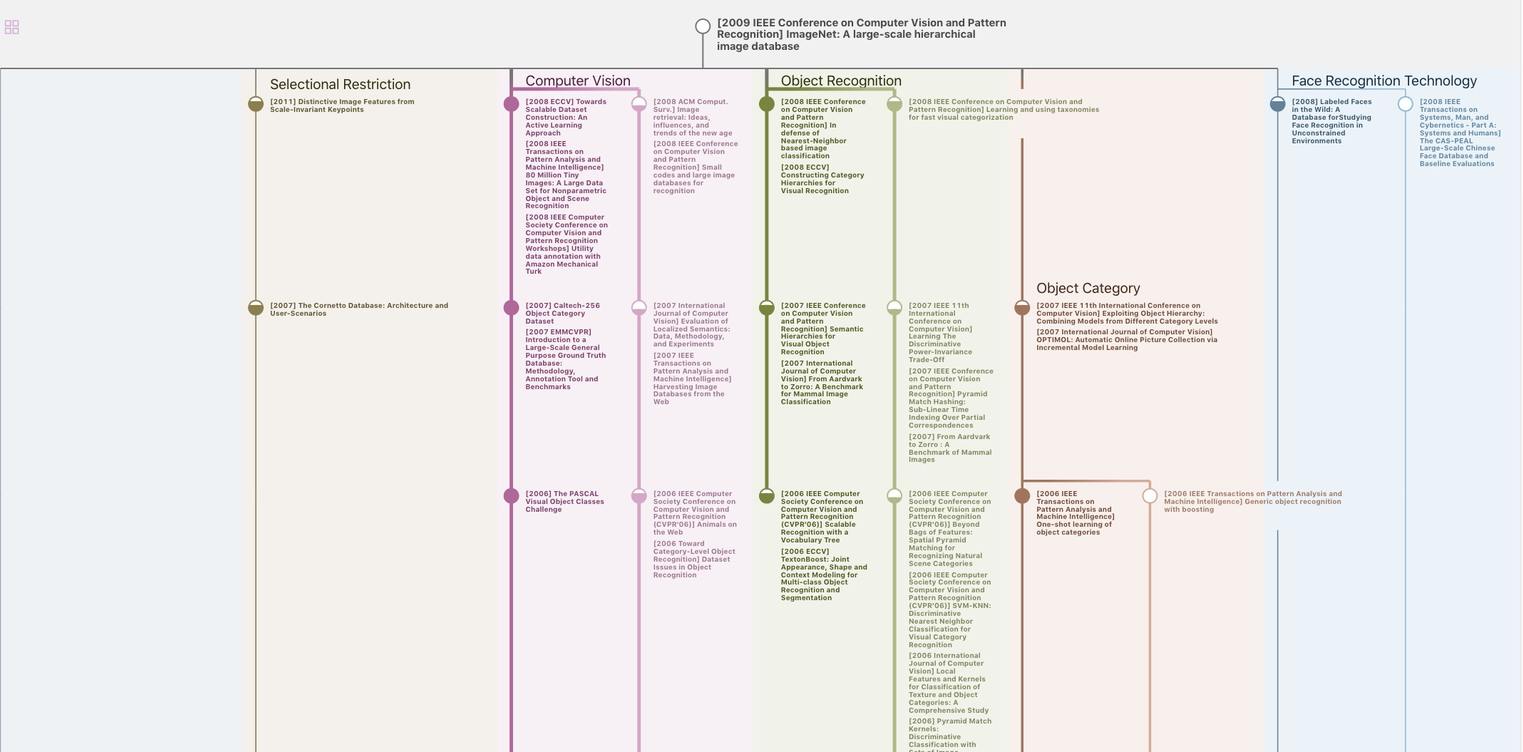
生成溯源树,研究论文发展脉络
Chat Paper
正在生成论文摘要