Fast Kernel Classifiers with Online and Active Learning
Journal of Machine Learning Research(2005)
摘要
Very high dimensional learning systems become theoretically possible when training examples are abundant. The computing cost then becomes the limiting factor. Any efficient learning algorithm should at least take a brief look at each example. But should all examples be given equal attention?This contribution proposes an empirical answer. We first present an online SVM algorithm based on this premise. LASVM yields competitive misclassification rates after a single pass over the training examples, outspeeding state-of-the-art SVM solvers. Then we show how active example selection can yield faster training, higher accuracies, and simpler models, using only a fraction of the training example labels.
更多查看译文
关键词
state-of-the-art svm solvers,training example,competitive misclassification rate,lasvm yield,fast kernel classifiers,active example selection,faster training,brief look,online svm algorithm,active learning,training example label,efficient learning algorithm,limiting factor
AI 理解论文
溯源树
样例
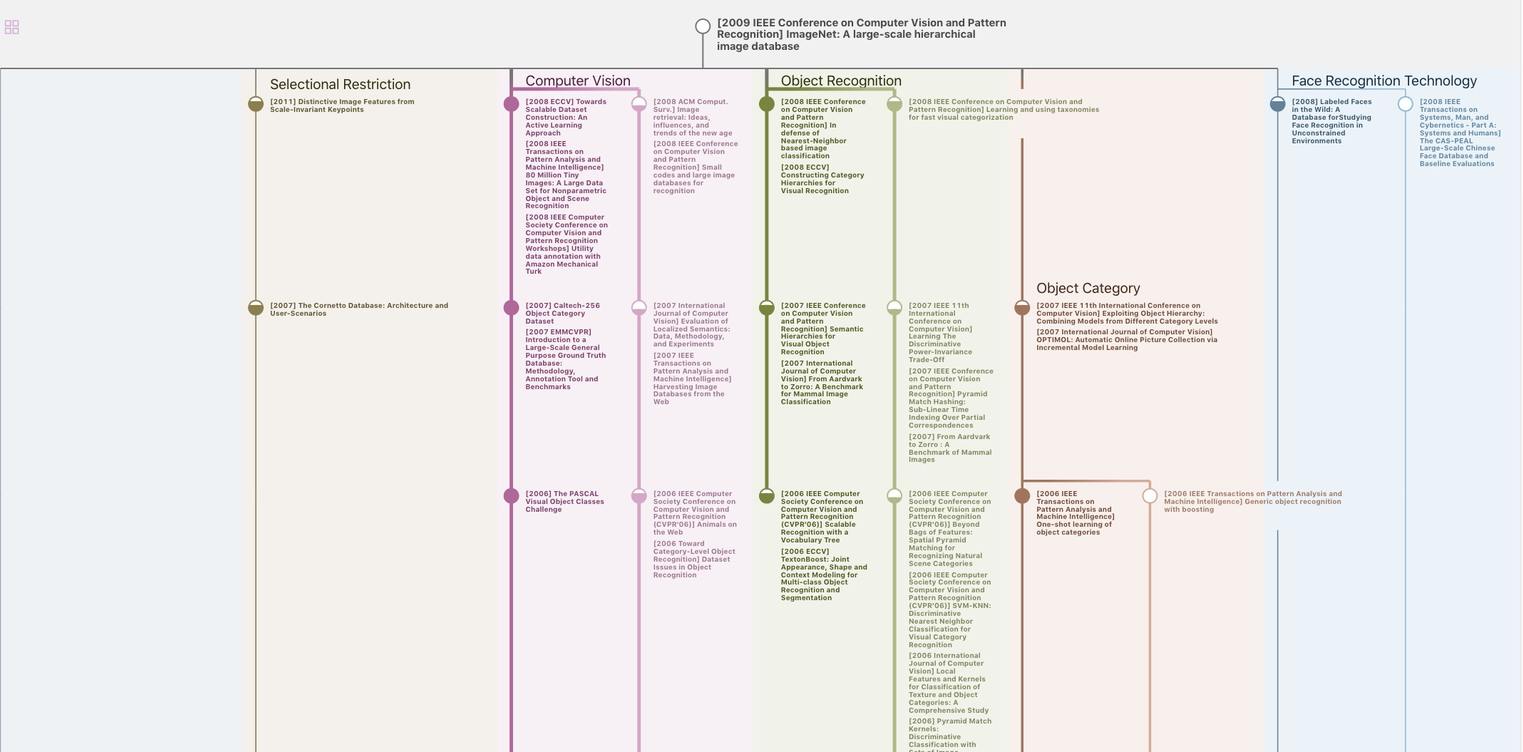
生成溯源树,研究论文发展脉络
Chat Paper
正在生成论文摘要