Anytime Belief Propagation Using Sparse Domains.
CoRR(2013)
摘要
For marginal inference on graphical models, belief propagation (BP) has been the algorithm of choice due to impressive empirical results on many models. These models often contain many variables and factors, however the domain of each variable (the set of values that the variable can take) and the neighborhood of the factors is usually small. When faced with models that contain variables with large domains and higher-order factors, BP is often intractable. The primary reason BP is unsuitable for large domains is the cost of message computations and representation, which is in the order of the cross-product of the neighbors’ domains. Existing extensions to BP that address this concern [1, 2, 4, 8, 9, 10, 13] use parameters that define the desired level of approximation, and return the approximate marginals at convergence. This results in poor anytime behavior. Since these algorithms try to directly achieve the desired approximation, the marginals during inference cannot be characterized, and are often inconsistent with each other. Further, the relationship of the parameter that controls the approximation to the quality of the intermediate marginals is often unclear. As a result, these approaches are not suitable for applications that require consistent, anytime marginals but are willing to trade-off error for speed, for example applications that involve real-time tracking or user interactions. There is a need for an anytime algorithm that can be interrupted to obtain consistent marginals corresponding to fixed points of a well-defined objective, and can improve the quality of the marginals over the execution period, eventually obtaining the BP marginals.
更多查看译文
AI 理解论文
溯源树
样例
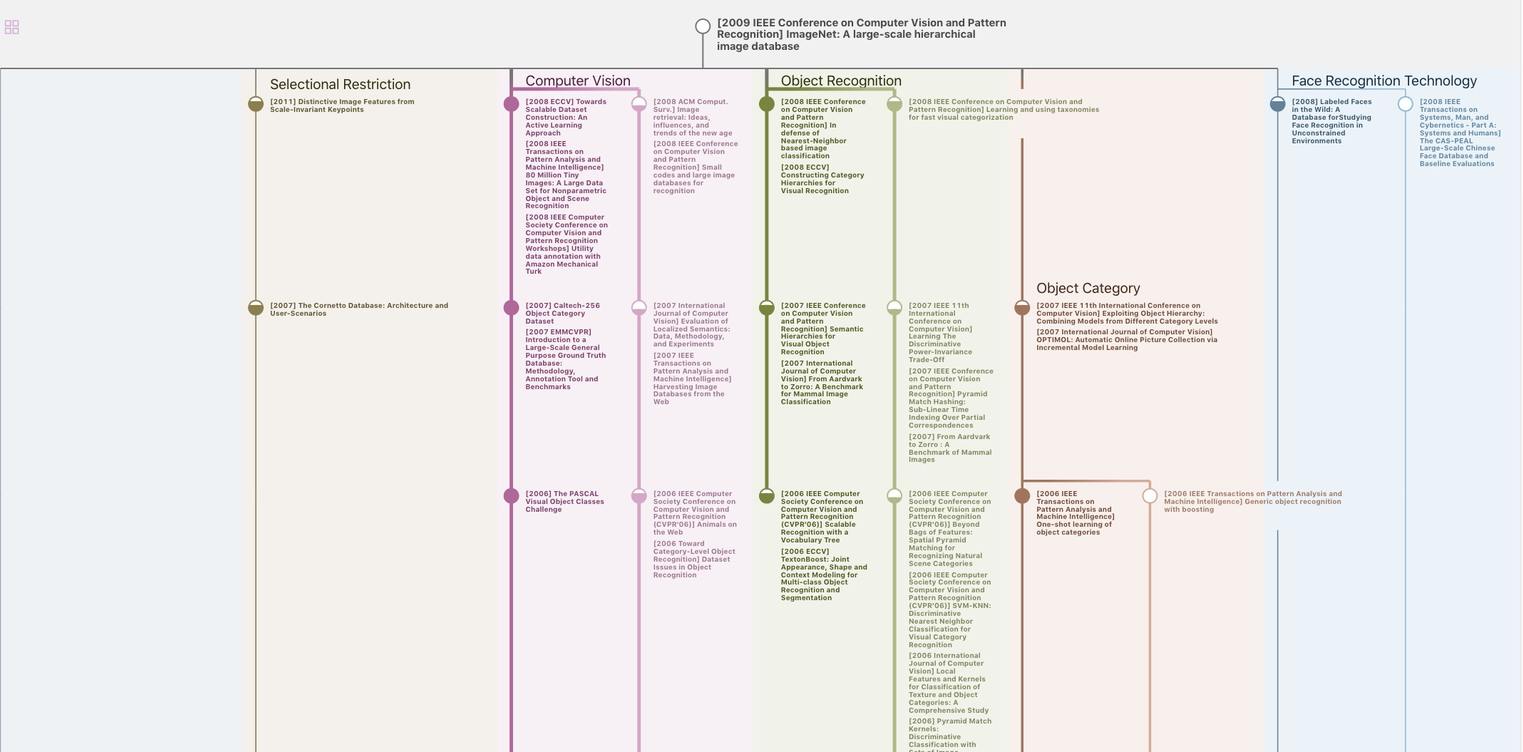
生成溯源树,研究论文发展脉络
Chat Paper
正在生成论文摘要