Applying one-vs-one and one-vs-all classifiers in k-nearest neighbour method and support vector machines to an otoneurological multi-class problem.
PubMed(2011)
摘要
We studied how the splitting of a multi-class classification problem into multiple binary classification tasks, like One-vs-One (OVO) and One-vs-All (OVA), affects the predictive accuracy of disease classes. Classifiers were tested with an otoneurological data using 10-fold cross-validation 10 times with k-Nearest Neighbour (k-NN) method and Support Vector Machines (SVM). The results showed that the use of multiple binary classifiers improves the classification accuracies of disease classes compared to one multi-class classifier. In general, OVO classifiers worked out better with this data than OVA classifiers. Especially, the OVO with k-NN yielded the highest total classification accuracies.
更多查看译文
关键词
multi-class classification,binary classifiers,otoneurology,k-nearest neighbour method,support vector machines
AI 理解论文
溯源树
样例
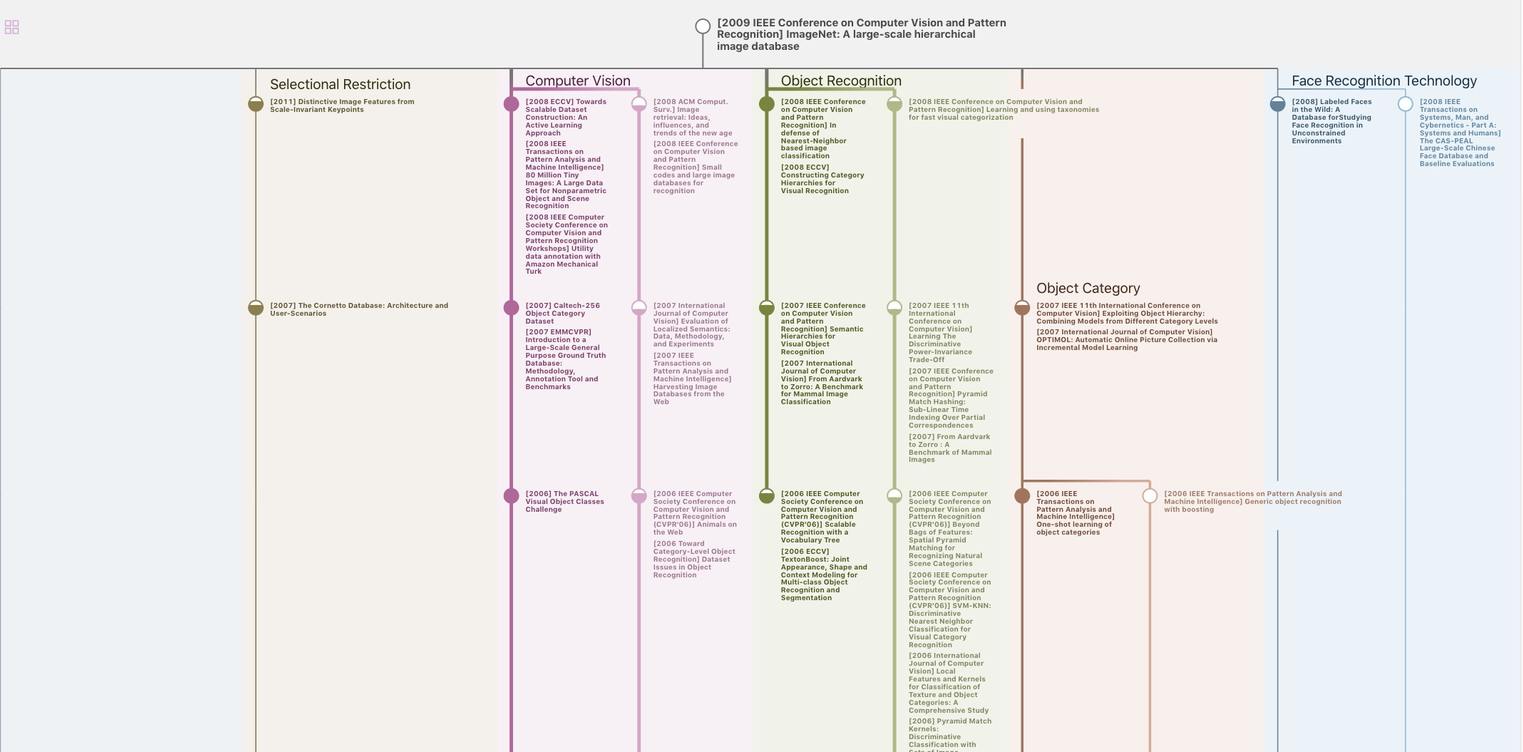
生成溯源树,研究论文发展脉络
Chat Paper
正在生成论文摘要