A Study of Statistical Methods for Function Prediction of Protein Motifs
Applied Bioinformatics(2012)
摘要
Automatic discovery of new protein motifs (i.e. amino acid patterns) is one of the major challenges in bioinformatics. Several algorithms have been proposed that can extract statistically significant motif patterns from any set of protein sequences. With these methods, one can generate a large set of candidate motifs that may be biologically meaningful. This article examines methods to predict the functions of these candidate motifs. We use several statistical methods: a popularity method, a mutual information method and probabilistic translation models. These methods capture, from different perspectives, the correlations between the matched motifs of a protein and its assigned Gene Ontology™ terms that characterise the function of the protein. We evaluate these different methods using the known motifs in the InterPro database. Each method is used to rank candidate terms for each motif. We then use the expected mean reciprocal rank to evaluate the performance. The results show that, in general, all these methods perform well, suggesting that they can all be useful for predicting the function of an unknown motif. Among the methods tested, a probabilistic translation model with a popularity prior performs the best.
更多查看译文
关键词
Gene Ontology,Mutual Information,Popularity Method,Pattern Discovery,Translation Model
AI 理解论文
溯源树
样例
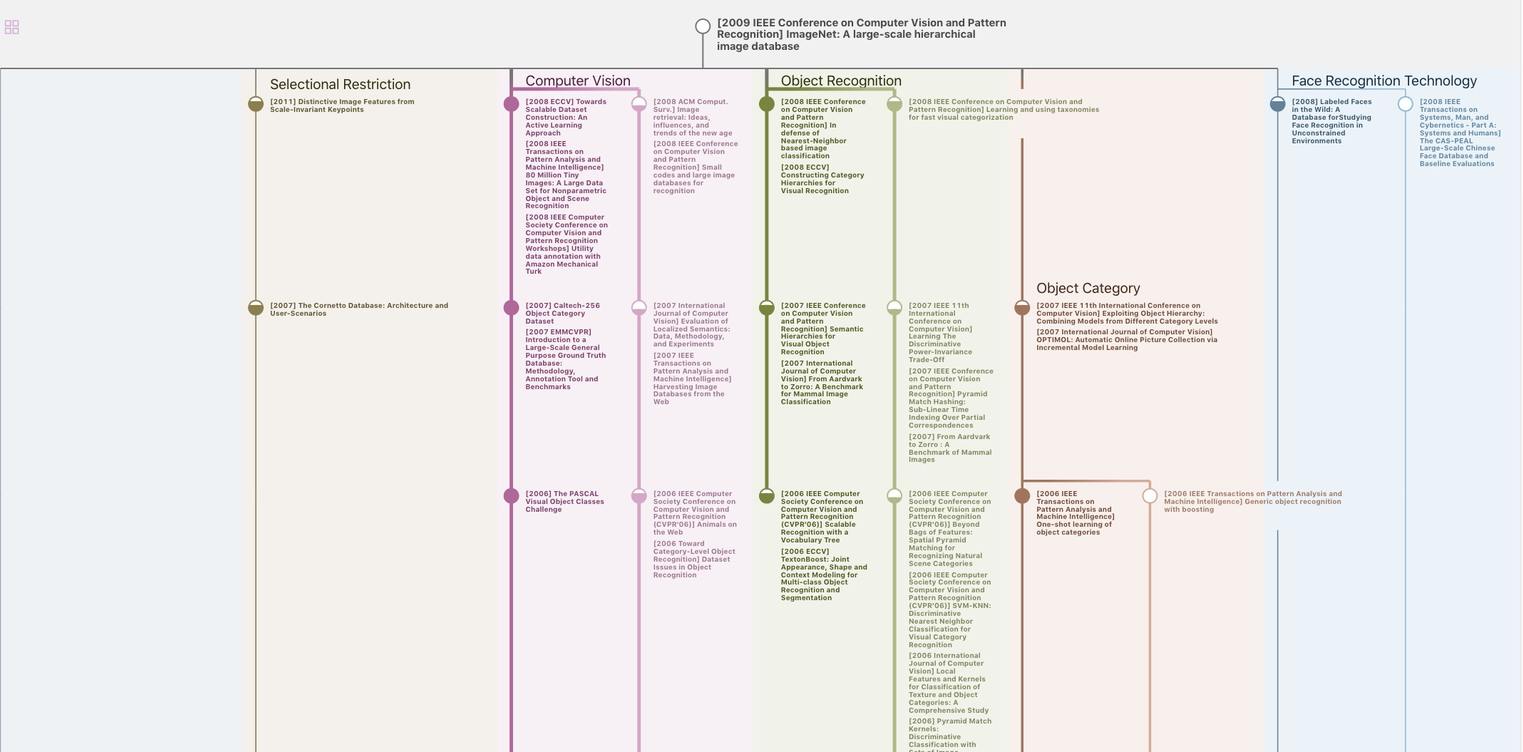
生成溯源树,研究论文发展脉络
Chat Paper
正在生成论文摘要