The entire quantile path of a risk-agnostic SVM classifier
UAI '09 Proceedings of the Twenty-Fifth Conference on Uncertainty in Artificial Intelligence(2012)
摘要
A quantile binary classifier uses the rule: Classify x as + 1 if P(Y = 1|X = x) ≥ τ, and as -1 otherwise, for a fixed quantile parameter τ ∈ [0, 1]. It has been shown that Support Vector Machines (SVMs) in the limit are quantile classifiers with τ = 1/2. In this paper, we show that by using asymmetric cost of misclassification SVMs can be appropriately extended to recover, in the limit, the quantile binary classifier for any τ. We then present a principled algorithm to solve the extended SVM classifier for all values of τ simultaneously. This has two implications: First, one can recover the entire conditional distribution P(Y = 1|X = x) = τ for τ ∈ [
更多查看译文
关键词
support vector machines,entire conditional distribution,asymmetric cost,fixed quantile parameter,entire quantile path,principled algorithm,misclassification svms,quantile binary classifier,extended svm classifier,risk-agnostic svm classifier,quantile classifier
AI 理解论文
溯源树
样例
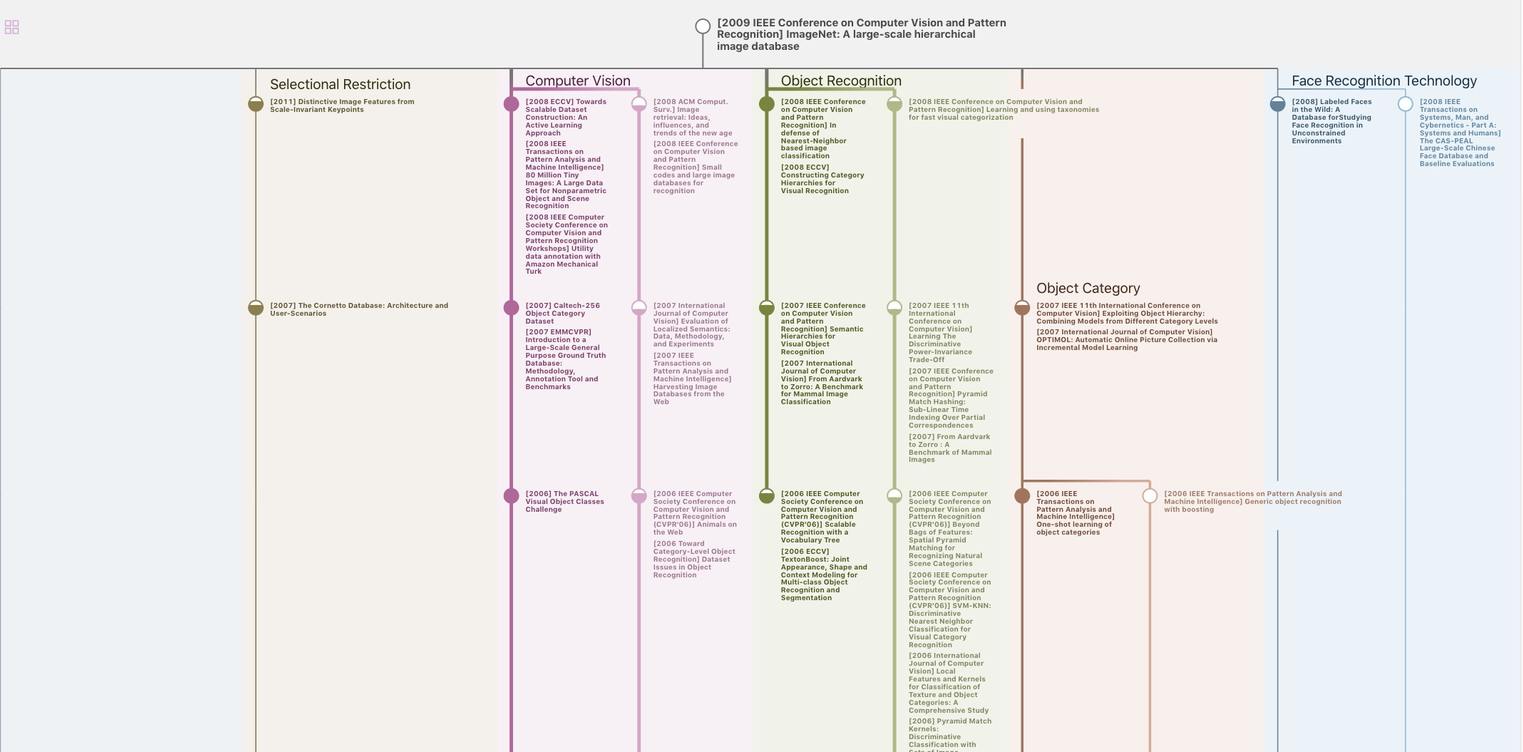
生成溯源树,研究论文发展脉络
Chat Paper
正在生成论文摘要