Density-Based Clustering over an Evolving Data Stream with Noise
SIAM Proceedings Series(2006)
摘要
Clustering is an important task in mining evolving data streams. Beside the limited memory and one-pass constraints, the nature of evolving data streams implies the following requirements for stream clustering: no assumption on the number of clusters, discovery of clusters with arbitrary shape and ability to handle outliers. While a lot of clustering algorithms for data streams have been proposed, they offer no solution to the combination of these requirements. In this paper, we present Den Stream, a new approach for discovering clusters in an evolving data stream. The "dense" micro-cluster (named core-micro-cluster) is introduced to summarize the clusters with arbitrary shape, while the potential core-micro-cluster and outlier micro-cluster structures are proposed to maintain and distinguish the potential clusters and outliers. A novel pruning strategy is designed based on these concepts, which guarantees the precision of the weights of the micro-clusters with limited memory. Our performance study over a number of real and synthetic data sets demonstrates the effectiveness and efficiency of our method.
更多查看译文
关键词
Data mining algorithms,Density based clustering,Evolving data streams
AI 理解论文
溯源树
样例
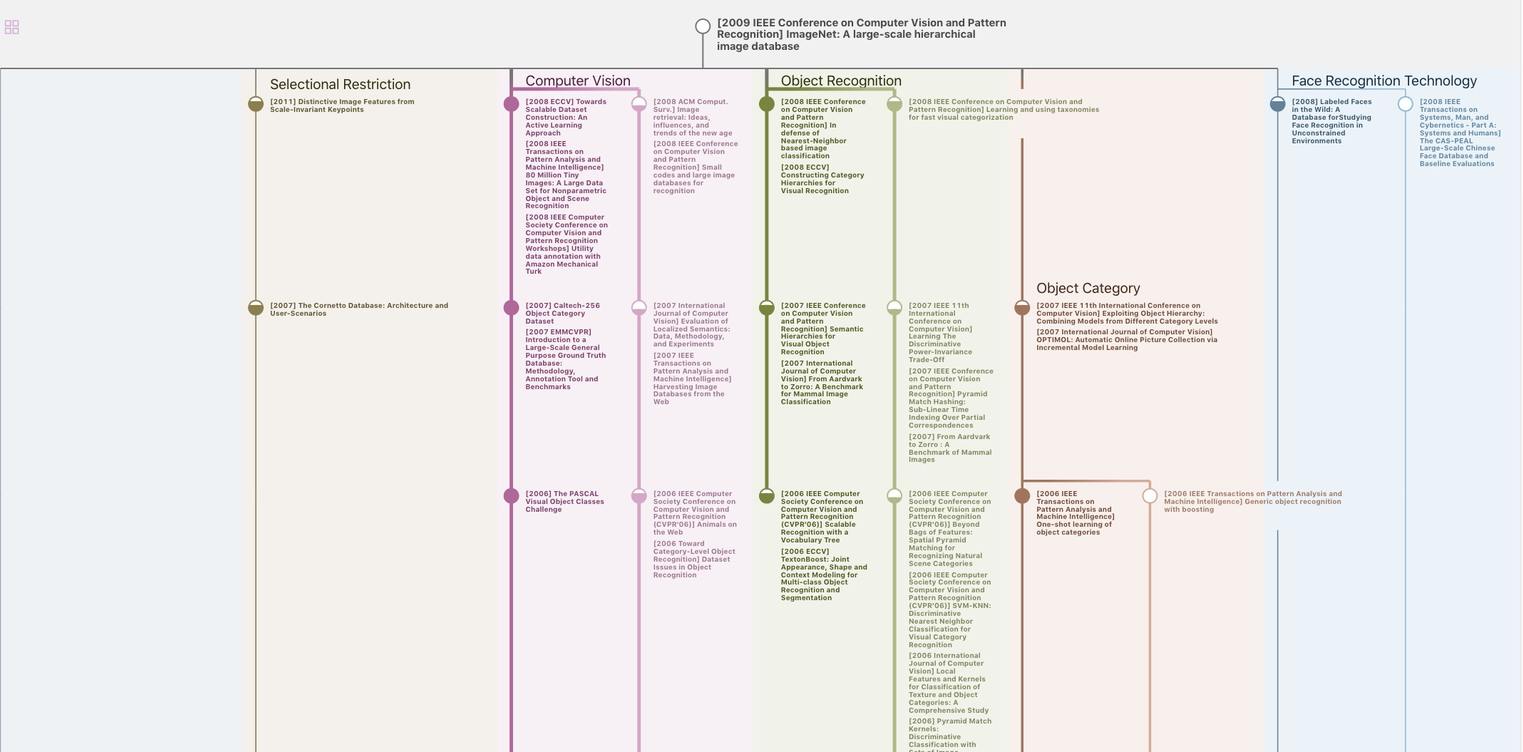
生成溯源树,研究论文发展脉络
Chat Paper
正在生成论文摘要