Bayesian compressed sensing with unknown measurement noise level.
ACSSC(2013)
摘要
In sparse Bayesian learning (SBL) approximate Bayesian inference is applied to find sparse estimates from observations corrupted by additive noise. Current literature only vaguely considers the case where the noise level is unknown a priori. We show that for most state-of-the-art reconstruction algorithms based on the fast inference scheme noise precision estimation results in increased computational complexity and reconstruction error. We propose a three-layer hierarchical prior model which allows for the derivation of a fast inference algorithm that estimates the noise precision with no complexity increase. Numerical results show that it matches or surpasses other algorithms in terms of reconstruction error.
更多查看译文
关键词
computational modeling,noise,vectors
AI 理解论文
溯源树
样例
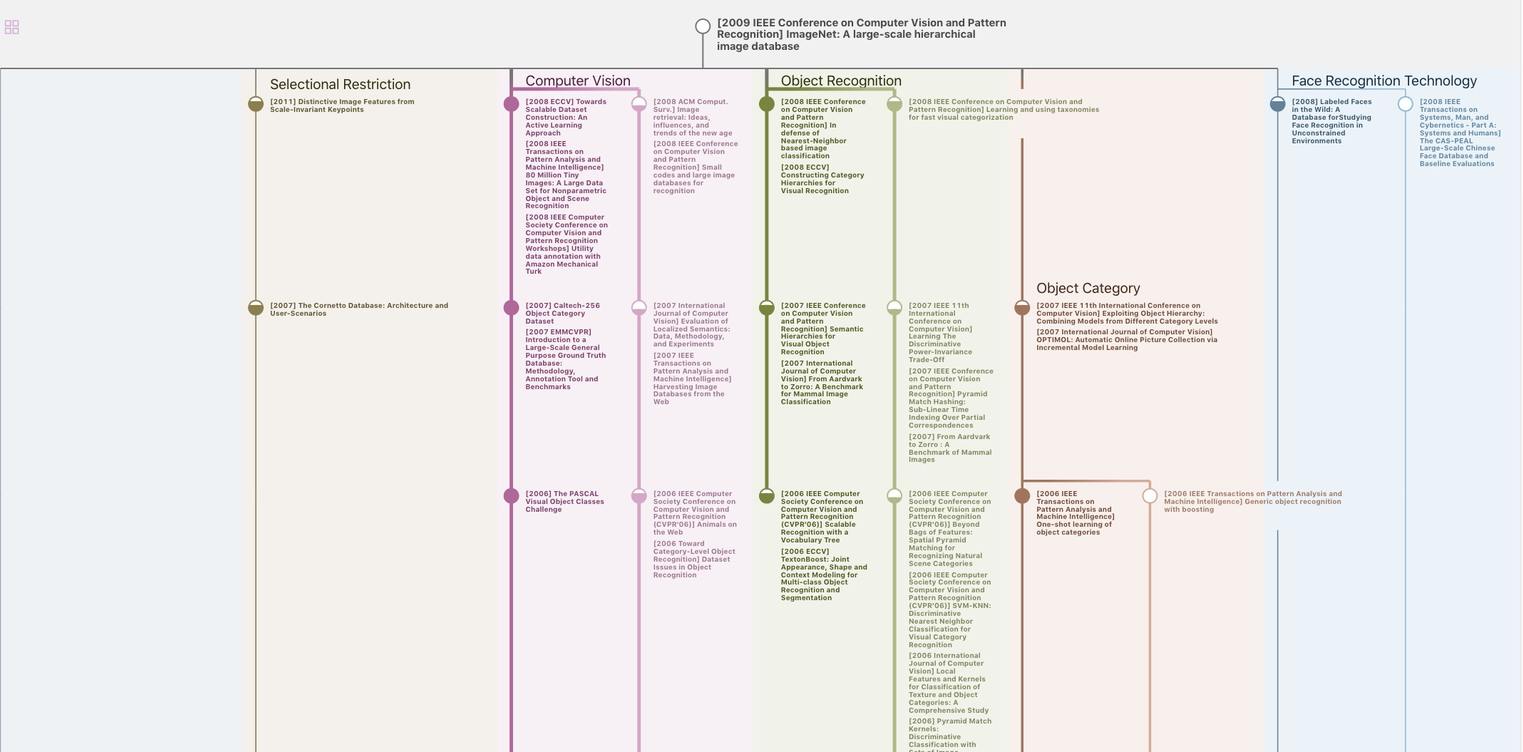
生成溯源树,研究论文发展脉络
Chat Paper
正在生成论文摘要