Graph Matching with Anchor Nodes: A Learning Approach
Computer Vision and Pattern Recognition(2018)
摘要
In this paper, we consider the weighted graph matching problem with partially disclosed correspondences between a number of anchor nodes. Our construction exploits recently introduced node signatures based on graph Laplacians, namely the Laplacian family signature (LFS) on the nodes, and the pair wise heat kernel map on the edges. In this paper, without assuming an explicit form of parametric dependence nor a distance metric between node signatures, we formulate an optimization problem which incorporates the knowledge of anchor nodes. Solving this problem gives us an optimized proximity measure specific to the graphs under consideration. Using this as a first order compatibility term, we then set up an integer quadratic program (IQP) to solve for a near optimal graph matching. Our experiments demonstrate the superior performance of our approach on randomly generated graphs and on two widely-used image sequences, when compared with other existing signature and adjacency matrix based graph matching methods.
更多查看译文
关键词
graph laplacians,optimized proximity measure,quadratic programming,first order compatibility term,weighted graph matching problem,adjacency matrix,integer programming,pattern matching,near optimal graph matching,existing signature,laplacian family signature,anchor nodes,node signatures,lfs,optimization problem,image sequences,explicit form,weighted graph,randomly generated graphs,graph theory,integer quadratic program,learning approach,pairwise heat kernel map,node signature,iqp,graph matching,anchor node,niobium,heating,vectors,kernel,approximation algorithms
AI 理解论文
溯源树
样例
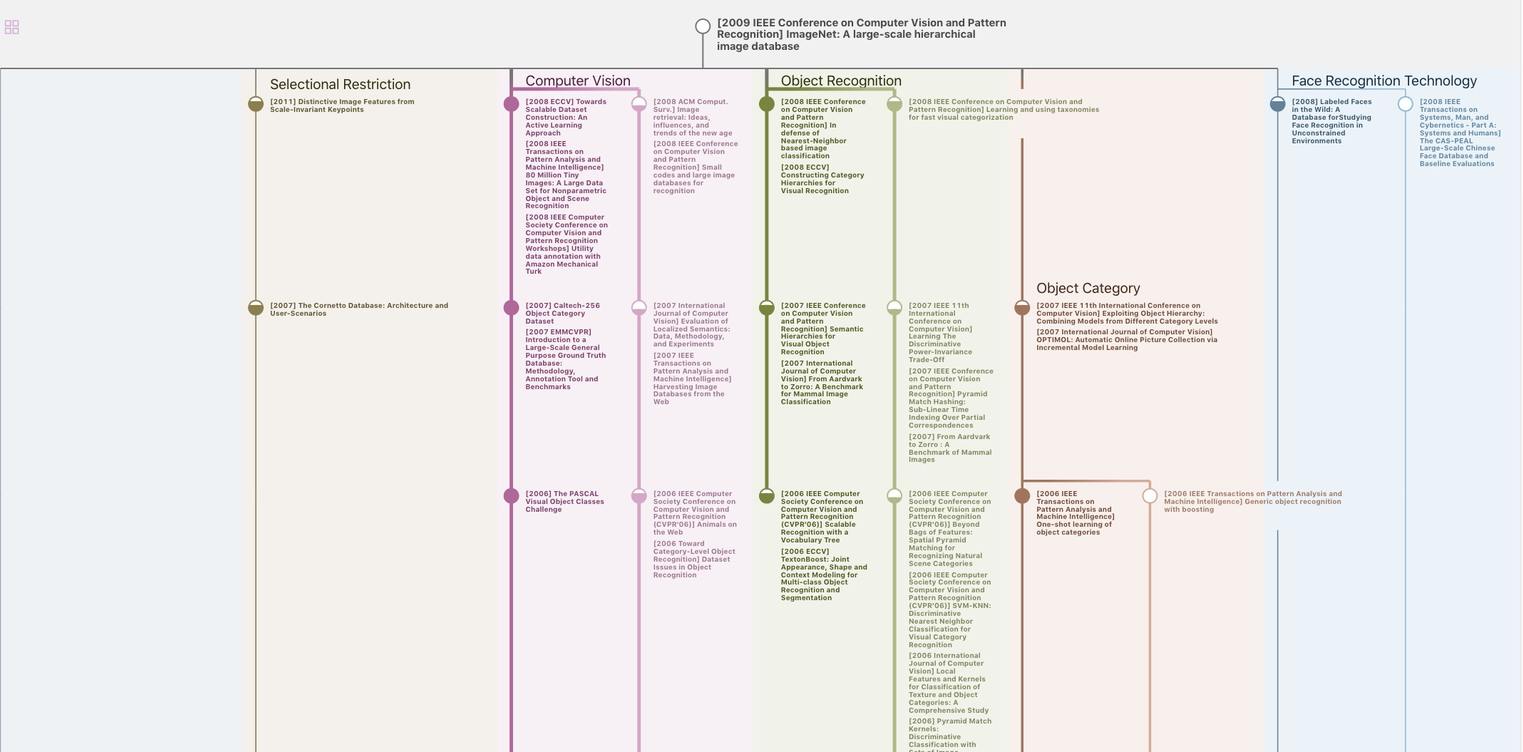
生成溯源树,研究论文发展脉络
Chat Paper
正在生成论文摘要