Influence Maximization: Near-Optimal Time Complexity Meets Practical Efficiency
MOD(2014)
摘要
Given a social network G and a constant k, the influence maximization problem asks for k nodes in G that (directly and indirectly) influence the largest number of nodes under a pre-defined diffusion model. This problem finds important applications in viral marketing, and has been extensively studied in the literature. Existing algorithms for influence maximization, however, either trade approximation guarantees for practical efficiency, or vice versa. In particular, among the algorithms that achieve constant factor approximations under the prominent independent cascade (IC) model or linear threshold (LT) model, none can handle a million-node graph without incurring prohibitive overheads. This paper presents TIM, an algorithm that aims to bridge the theory and practice in influence maximization. On the theory side, we show that TIM runs in O((k+\ell) (n+m) \log n / \epsilon^2) expected time and returns a (1-1/e-\epsilon)-approximate solution with at least 1 - n^{-\ell} probability. The time complexity of TIM is near-optimal under the IC model, as it is only a \log n factor larger than the \Omega(m + n) lower-bound established in previous work (for fixed k, \ell, and \epsilon). Moreover, TIM supports the triggering model, which is a general diffusion model that includes both IC and LT as special cases. On the practice side, TIM incorporates novel heuristics that significantly improve its empirical efficiency without compromising its asymptotic performance. We experimentally evaluate TIM with the largest datasets ever tested in the literature, and show that it outperforms the state-of-the-art solutions (with approximation guarantees) by up to four orders of magnitude in terms of running time. In particular, when k = 50, \epsilon = 0.2, and \ell = 1, TIM requires less than one hour on a commodity machine to process a network with 41.6 million nodes and 1.4 billion edges.
更多查看译文
关键词
Algorithms,Theory,Experimentation
AI 理解论文
溯源树
样例
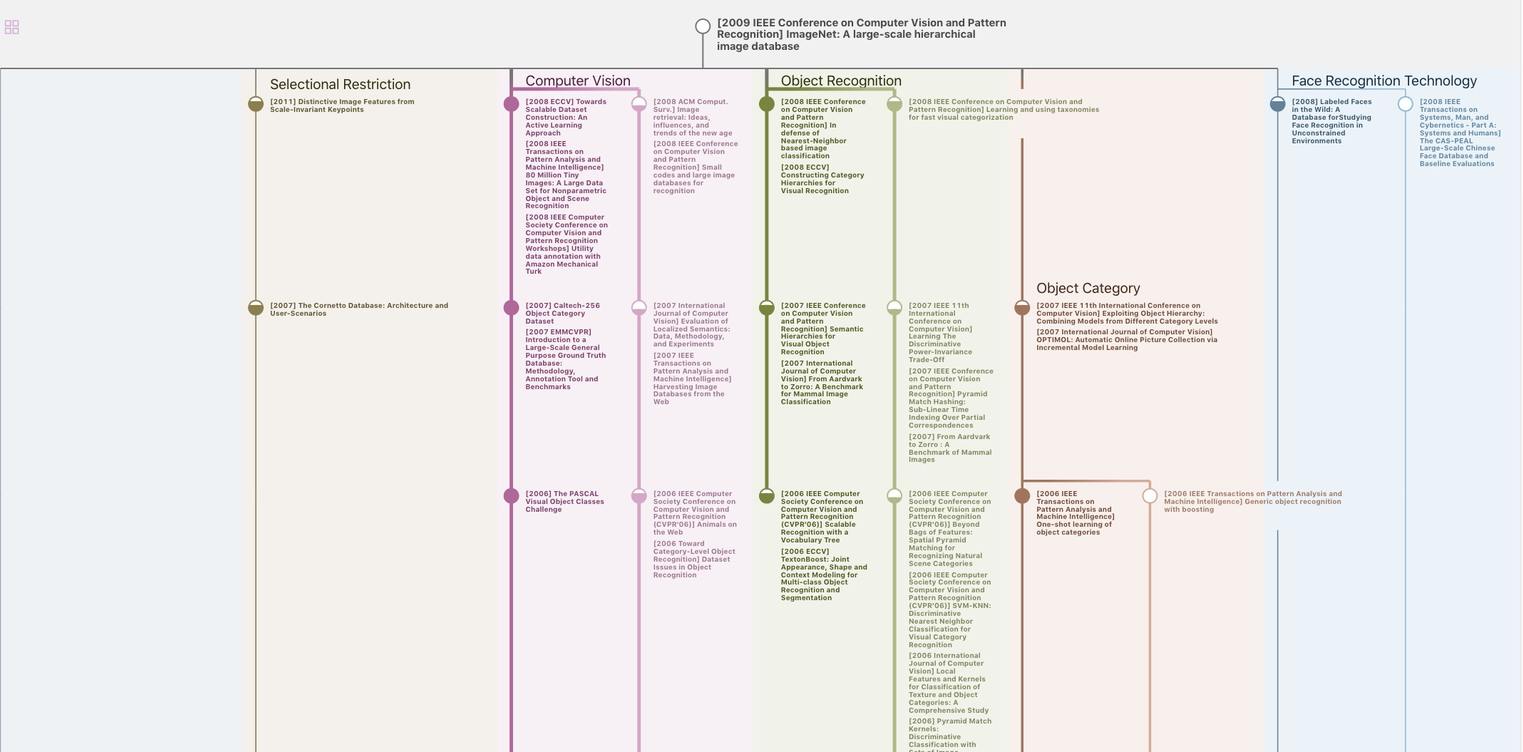
生成溯源树,研究论文发展脉络
Chat Paper
正在生成论文摘要