Adaptive Machinery to Support Natural Conversations
msra(2013)
摘要
Real-time adaptation and ongoing learning promise to provide computational machinery to support fluid conversational interaction. We will focus on representations and inferential strategies that can provide real-time adaptation to multiple characteristics of a conversation. The work approaches conversation from a perspective of inference and decision making under uncertainty. Automated methods for engaging users in conversations must address critical uncertainties about users'attentional status, utterances, and goals. Although uncertainties arising in conversation with a computer may pertain largely to the special context of interacting with a computer, they are not uncommon in conversations among people. Limitations of speech recognition hardware and software may severely limit the abilities of computers to interpret a user's utterances with certainty. Furthermore, automated inference procedures and representations may not be able disambiguate a user's intentions and needs. However, grappling with uncertainties about someone's utterances and about their associated goals is also common in human to human conversations. People frequently employ a wide range of strategies to resolve uncertainty and repair possible misunderstandings as part of a collaborative process known as grounding, or converging on a shared understanding. Although the precise nature and distribution of the uncertainties may shift in moving from human--human to human--computer conversation, we believe that there is great opportunity to develop representations and inference strategies that allow computers to engage users in a natural, expected manner in the course of converging on a shared understanding during conversations. In the Conversational Architectures project, we have been exploring methods for performing inference under uncertainty about the best actions to take to resolve key uncertainties and ambiguities in conversational systems. Our research centers on the development of principles and architectures for engaging in grounding in conversation. Our approach is motivated by the intuition that modeling the way people in conversation employ natural, compensatory strategies for resolving ambiguity and pursuing a shared understanding can provide adaptive machinery for grappling with uncertainties associated with the limited abilities of computers, in a manner akin to the familiar experience of conversing with a person of poor language or hearing skills. In the extended abstract, we will briefly introduce four levels of analysis and describe representations and inference strategies for managing uncertainty within and between levels (Paek & Horvitz, 1999). The operation of the methods will be highlighted by reviewing interactions drawn from conversation between a user and a prototype system named the Bayesian Receptionist (Horvitz & Paek, 1999). The Bayesian Receptionist harnesses Bayesian inference, natural language parsing, and speech recognition (Heidorn, 1999) to control dialog about tasks usually handled by receptionists at the front desks of buildings at the Microsoft corporate campus. Our framework is built upon earlier research that has investigated how people collaboratively contribute to a conversation at successive levels of mutual understanding through grounding (Clark & Schaefer, 1987, 1989). While researchers have examined the relationship of these multiple levels with miscommunication (Brennan & Hulteen, 1995; Dillenbourg et al., 1996; Traum, 1994; Traum & Dillenbourg, 1996, 1998), relatively little work has focused on exploiting uncertainty; for example, by explicitly quantifying uncertainty in terms of probabilities at each level. The framework we present broadens the scope of previous models of grounding and referential expressions (Edmonds, 1993; Heeman, 1991; Heeman & Hirst, 1992; Hirst et al., 1994) by highlighting the efficacy of Bayesian networks and decision theory to reason about uncertainty before and during misunderstanding. Furthermore, the introduction of decision theory allows systems to use expected utility to provide fine-grain, context-sensitive guidance of compensatory measures, rather than relying solely on ad hoc procedures (Brennan, 1998).
更多查看译文
AI 理解论文
溯源树
样例
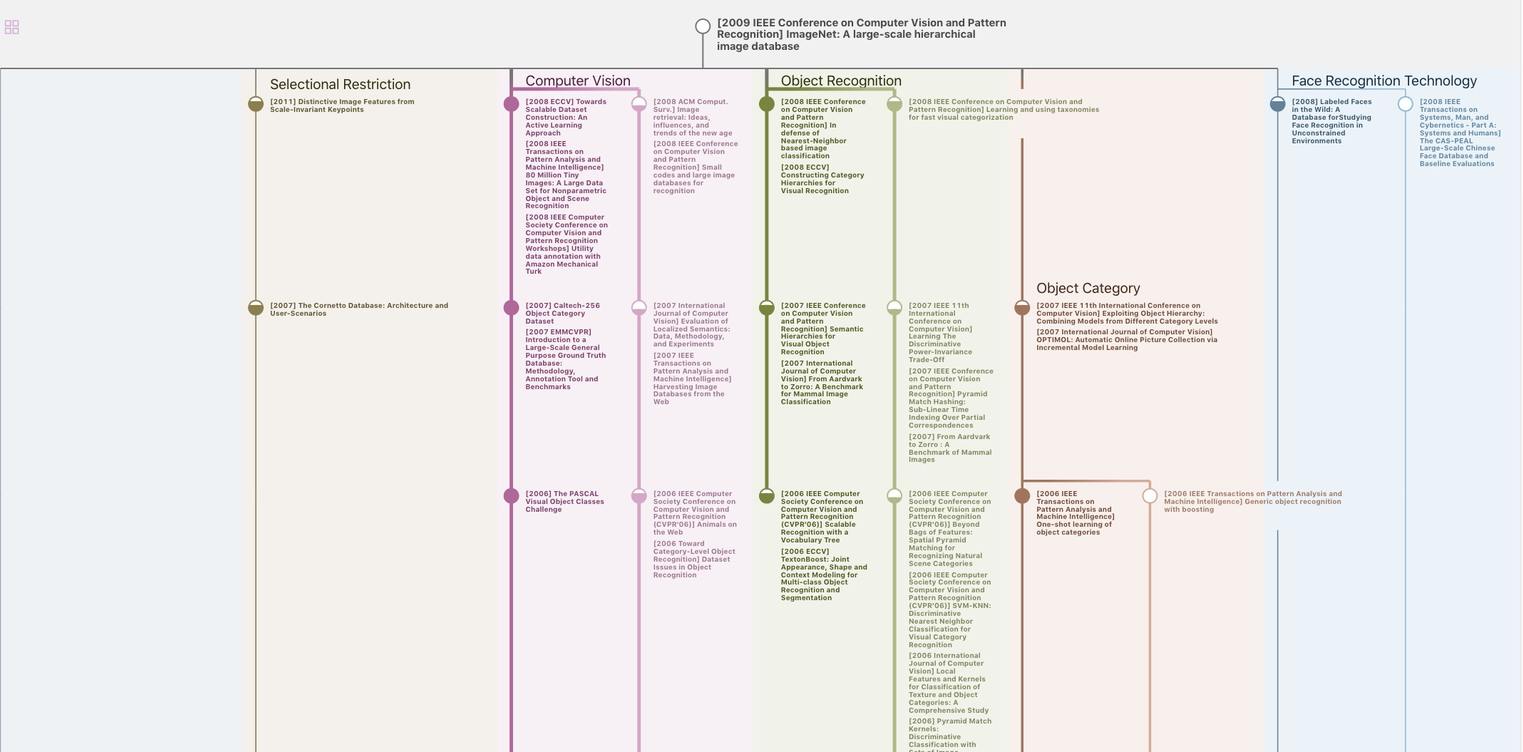
生成溯源树,研究论文发展脉络
Chat Paper
正在生成论文摘要