Information-Theoretic Strategies For Quantifying Variability And Model-Reality Comparison In The Climate System
18TH WORLD IMACS CONGRESS AND MODSIM09 INTERNATIONAL CONGRESS ON MODELLING AND SIMULATION: INTERFACING MODELLING AND SIMULATION WITH MATHEMATICAL AND COMPUTATIONAL SCIENCES(2009)
摘要
Model-reality comparison can be viewed in a communications context, with the observed data the "sent message," the model output "received message," and the model the noisy channel over which the message is transmitted (Figure 1). Information theory offers a way to assess literally the "information content" of any system and offers a means for objective quantification of model-observational data fidelity. The Shannon entropy (SE) H (X) is the measure of the amount of uncertainty, variability, or "surprise" present in a system variable X, while the mutual information (MI) I(X;Y) measures the amount of shared information or redundancy between two variables X and Y. Information theory's roots lie in the analysis of communication of data across a noisy channel (Figure 1) and offer a scheme for quantifying how well a message X coming from a transmitter arrives as Y at the receiver. A more general information-theoretic measure of message degradation is the Kullback-Leibler divergence (KLD), which quantifies insufficiency of agreement in the probatility density functions associated with X and Y. The ratio of MI to SE yields the amount of information shared by two datasets versus the information content of one alone. Unfortunately, these information-theoretic techniques work best for discrete rather than continuous systems. The reason is that evaluation of the SE for continuous systems-the differential entropy-does not constitute the continuum limit of the SE. Relative quantities such as the MI and KLD are always valid in the continuum case and are the continuum limit of their discrete counterparts, but they are just that-relative. This begs the question: Is there some way one can benchmark it against some continuum surrogate for the SE? Thus, one faces a choice when using information theory for model validation and intercomparison: (1) adopt coarse-graining strategies that are physically relevant, always aware that computed SE results are specific to a given discretisation, or (2) treat the data as continuous and use the MI combined with some benchmark quantity. In this paper, I adopt strategy (1), and restrict the scope to a variable that has well-agreed-upon discretisations-total cloud cover, which by observational convention is frequently coarse-grained by oktas, tenths, or percent.I first review basic concepts from information theory. I put forward the notion that the SE is an alternative measure of climate variability, and I evaluate it for reanalysis data and climate model output, producing global maps of the SE. I discuss how to structure sampling from two datasets to construct "messages" for use in information-theoretic model validation. I derive from the SE and MI a pair of fidelity ratios for assessing model-reality fidelity, and evaluate them for total cloud amount. I apply a modified KLD to assess model-reality agreement for local, temporally sampled total cloud and explain the relative strictness of the KLD- and MI-based validation standards. I conclude with a roadmap for analysing and validating the informatics of climate.
更多查看译文
关键词
Information Theory, Statistics, Climate Data Analysis
AI 理解论文
溯源树
样例
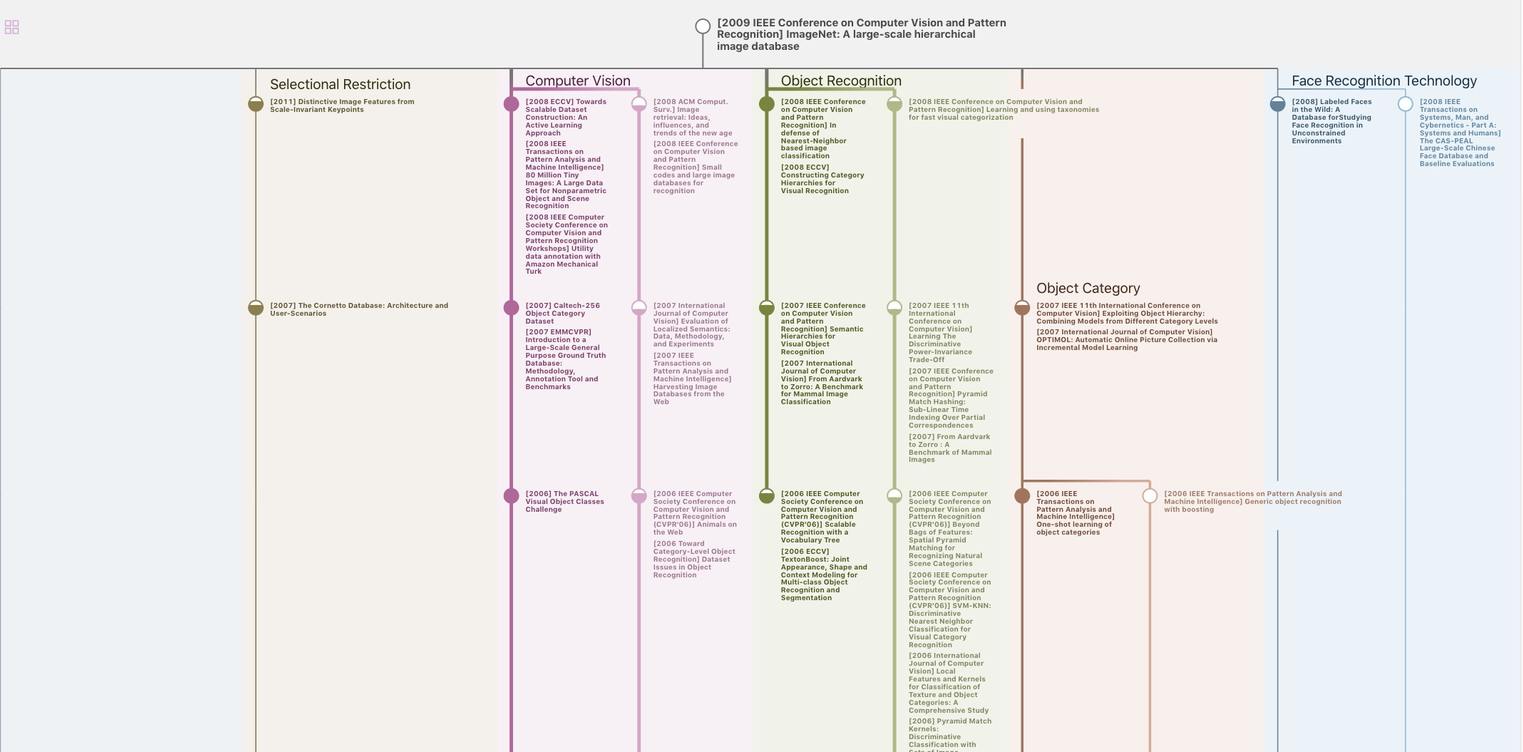
生成溯源树,研究论文发展脉络
Chat Paper
正在生成论文摘要