Regularized bundle methods for convex and non-convex risks
Journal of Machine Learning Research(2012)
摘要
Machine learning is most often cast as an optimization problem. Ideally, one expects a convex objective function to rely on efficient convex optimizers with nice guarantees such as no local optima. Yet, non-convexity is very frequent in practice and it may sometimes be inappropriate to look for convexity at any price. Alternatively one can decide not to limit a priori the modeling expressivity to models whose learning may be solved by convex optimization and rely on non-convex optimization algorithms. The main motivation of this work is to provide efficient and scalable algorithms for non-convex optimization. We focus on regularized unconstrained optimization problems which cover a large number of modern machine learning problems such as logistic regression, conditional random fields, large margin estimation, etc. We propose a novel algorithm for minimizing a regularized objective that is able to handle convex and non-convex, smooth and non-smooth risks. The algorithm is based on the cutting plane technique and on the idea of exploiting the regularization term in the objective function. It may be thought as a limited memory extension of convex regularized bundle methods for dealing with convex and non convex risks. In case the risk is convex the algorithm is proved to converge to a stationary solution with accuracy e with a rate O(1/λε) where λ is the regularization parameter of the objective function under the assumption of a Lipschitz empirical risk. In case the risk is not convex getting such a proof is more difficult and requires a stronger and more disputable assumption. Yet we provide experimental results on artificial test problems, and on five standard and difficult machine learning problems that are cast as convex and non-convex optimization problems that show how our algorithm compares well in practice with state of the art optimization algorithms.
更多查看译文
关键词
efficient convex optimizers,regularized bundle method,convex regularized bundle method,convex optimization,convex objective function,non-convex optimization algorithm,non-convex optimization,objective function,art optimization algorithm,non-convex risk,non-convex optimization problem,non convex risk,optimization,cutting plane
AI 理解论文
溯源树
样例
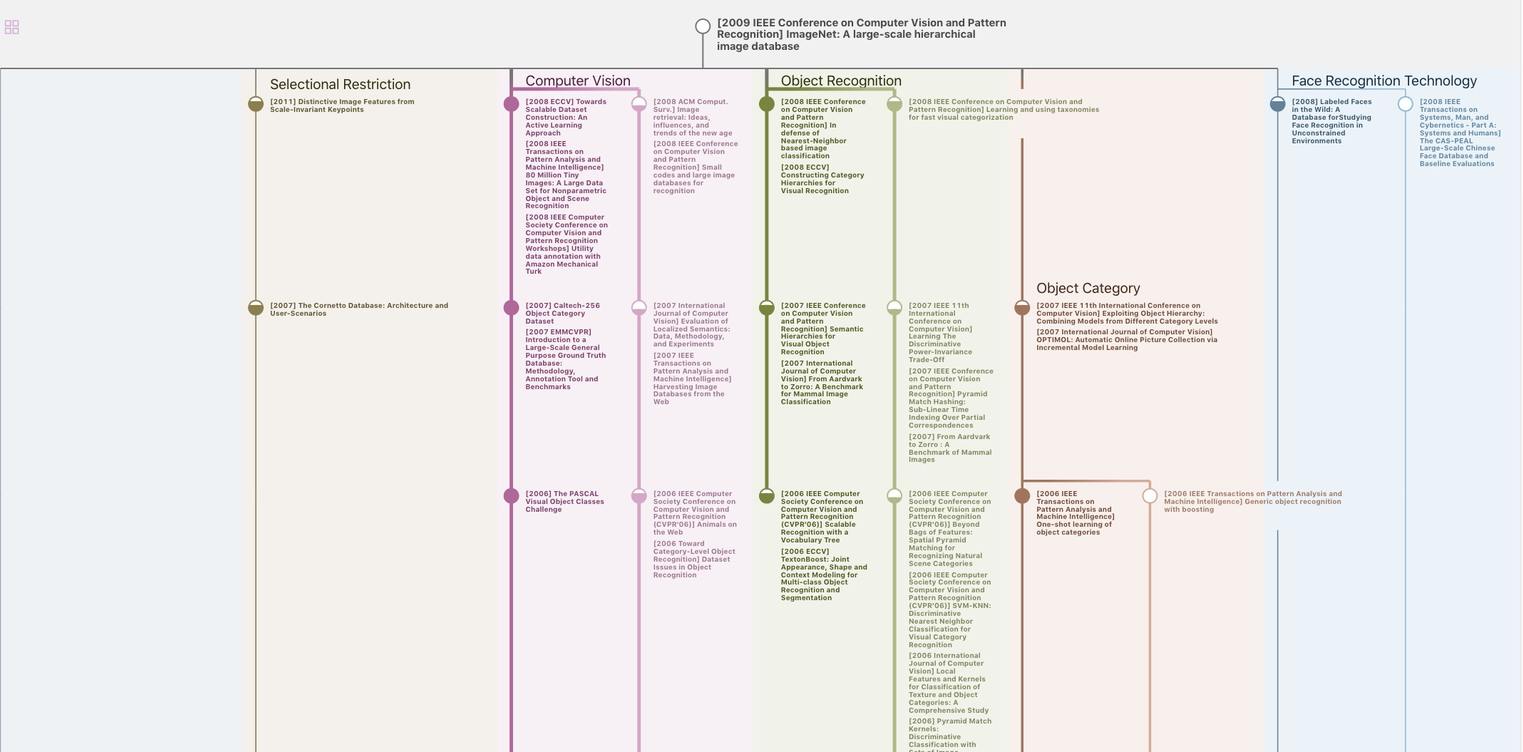
生成溯源树,研究论文发展脉络
Chat Paper
正在生成论文摘要