Learnability of Relatively Quantified Generalized Formulas
ALGORITHMIC LEARNING THEORY, PROCEEDINGS(2004)
摘要
In this paper we study the learning complexity of a vast class of quantifed formulas called Relatively Quantified Generalized Formulas. This class of formulas is parameterized by a set of predicates, called a basis. We give a complete classification theorem, showing that every basis gives rise to quantified formulas that are either polynomially learnable with equivalence queries, or not polynomially predictable with membership queries under some cryptographic assumption. We also provide a simple criteria distinguishing the learnable cases from the non-learnable cases.
更多查看译文
关键词
Generalize Formula, Constraint Satisfaction Problem, Concept Class, Target Concept, Unary Relation
AI 理解论文
溯源树
样例
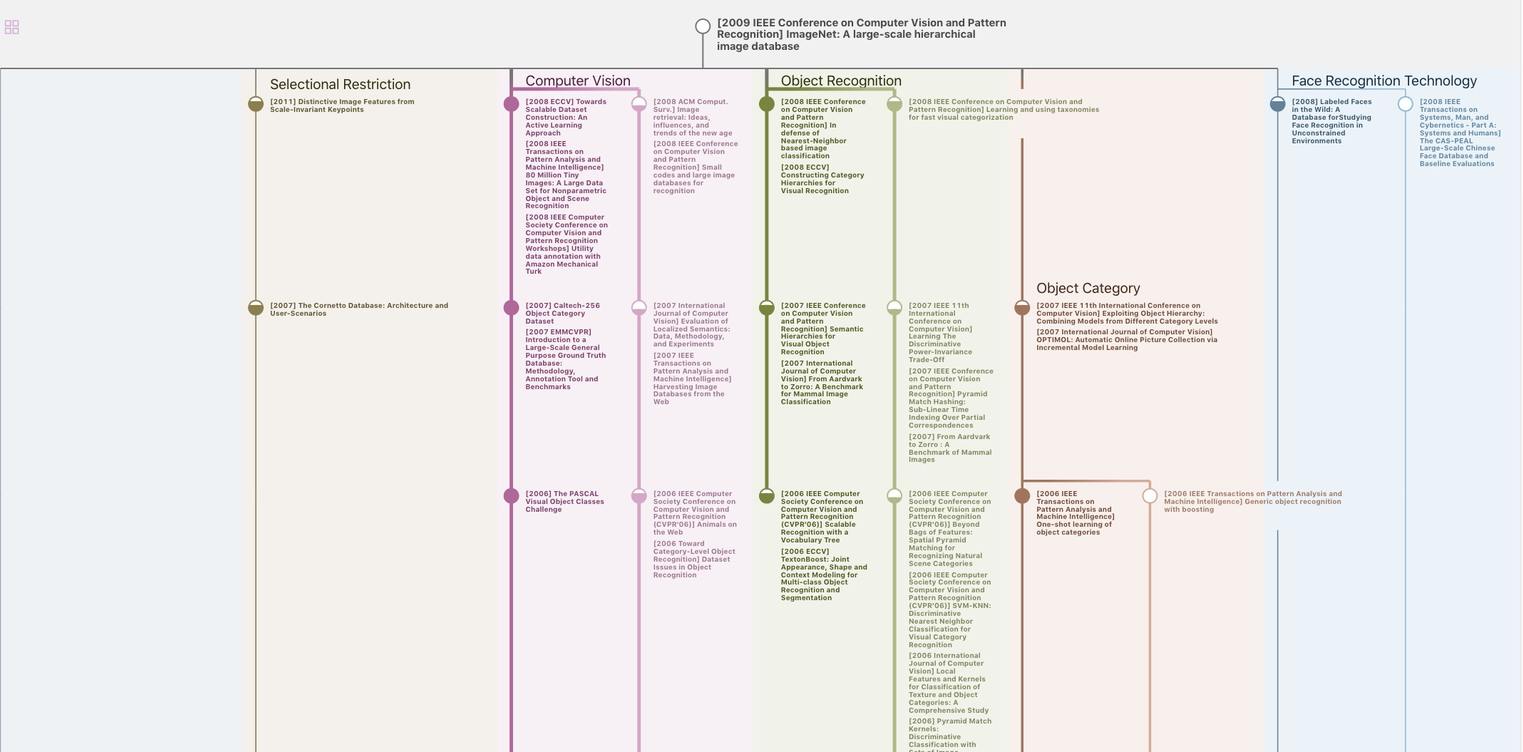
生成溯源树,研究论文发展脉络
Chat Paper
正在生成论文摘要