Discovering joint audio–visual codewords for video event detection
Machine Vision and Applications(2013)
摘要
Detecting complex events in videos is intrinsically a multimodal problem since both audio and visual channels provide important clues. While conventional methods fuse both modalities at a superficial level, in this paper we propose a new representation—called bi-modal words—to explore representative joint audio–visual patterns. We first build a bipartite graph to model relation across the quantized words extracted from the visual and audio modalities. Partitioning over the bipartite graph is then applied to produce the bi-modal words that reveal the joint patterns across modalities. Different pooling strategies are then employed to re-quantize the visual and audio words into the bi-modal words and form bi-modal Bag-of-Words representations. Since it is difficult to predict the suitable number of bi-modal words, we generate bi-modal words at different levels (i.e., codebooks with different sizes), and use multiple kernel learning to combine the resulting multiple representations during event classifier learning. Experimental results on three popular datasets show that the proposed method achieves statistically significant performance gains over methods using individual visual and audio feature alone and existing popular multi-modal fusion methods. We also find that average pooling is particularly suitable for bi-modal representation, and using multiple kernel learning to combine multi-modal representations at various granularities is helpful.
更多查看译文
关键词
Bi-modal words,Multimodal fusion,Multiple kernel learning,Event detection
AI 理解论文
溯源树
样例
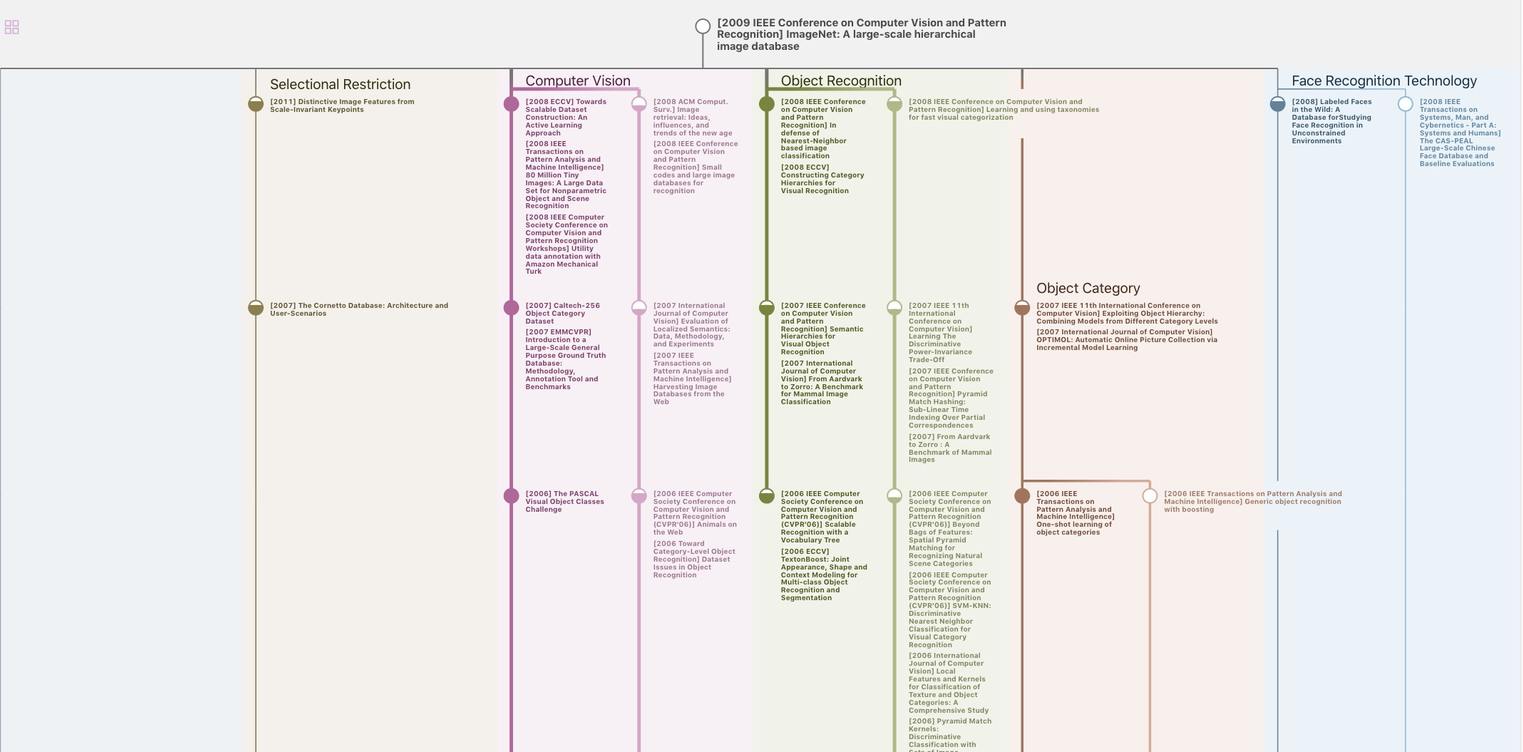
生成溯源树,研究论文发展脉络
Chat Paper
正在生成论文摘要