Improving the Statistical Arbitrage Strategy in Intraday Trading by Combining Extreme Learning Machine and Support Vector Regression with Linear Regression Models
Tools with Artificial Intelligence(2013)
摘要
In this paper we investigate the statistical and economic performance for statistical arbitrage strategy using Extreme Learning Machine (ELM) and Support Vector Regression (SVR) models, and their forecast combination through four linear combination models. The application of the traditional Kalman Filter for the statistical arbitrage strategy improves the statistical performance of ELM and SVR individual forecasts. It is presented evidence that the financial performance for most of cointegrated pairs can be improved by at least one linear combination technique.
更多查看译文
关键词
Kalman filters,electronic trading,learning (artificial intelligence),regression analysis,support vector machines,ELM,Kalman filter,SVR,economic performance,extreme learning machine,financial performance,intraday trading,linear combination model,linear regression model,statistical arbitrage strategy,support vector regression,Extreme Learning Machine,Forecast Combinations,Kalman Filter,Pair Trading,Statistical Arbitrage,Support Vector Regression
AI 理解论文
溯源树
样例
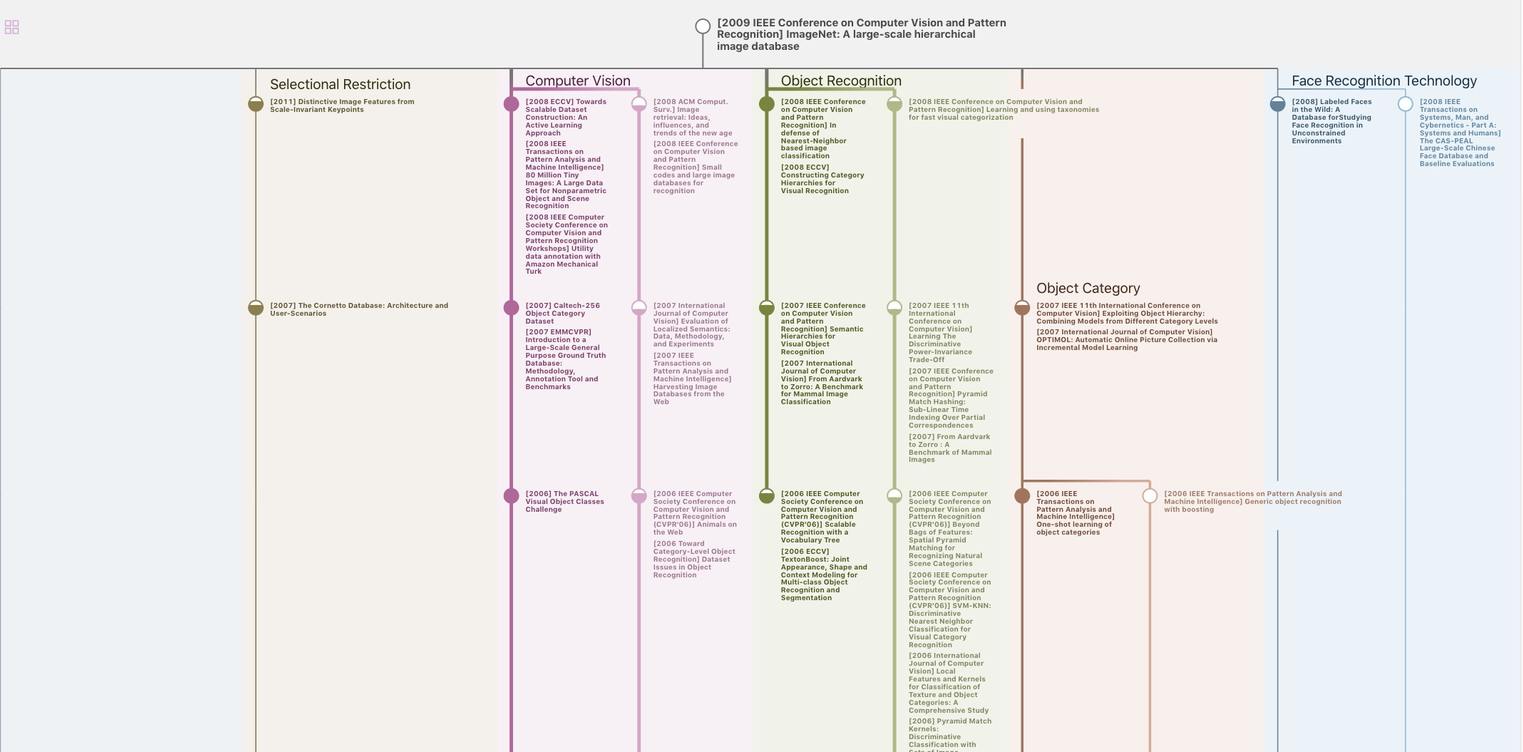
生成溯源树,研究论文发展脉络
Chat Paper
正在生成论文摘要