Attribute Weighted Value Difference Metric
Tools with Artificial Intelligence(2013)
摘要
Classification is an important task in data mining, while accurate class probability estimation is also desirable in real-world applications. Some probability-based classifiers, such as the k-nearest neighbor algorithm (KNN) and its variants, can estimate the class membership probabilities of the test instance. Unfortunately, a good classifier is not always a good class probability estimator. In this paper, we try to improve the class probability estimation performance of KNN and its variants. As we all know, KNN and its variants are all of the distance-related algorithms and their performance is closely related to the used distance metric. Value Difference Metric (VDM) is one of the widely used distance metrics for nominal attributes. Thus, in order to scale up the class probability estimation performance of the distance-related algorithms such as KNN and its variants, we propose an Attribute Weighted Value Difference Metric (AWVDM) in this paper. AWVDM uses the mutual information between the attribute variable and the class variable to weight the difference between two attribute values of each pair of instances. Experimental results on 36 UCI benchmark datasets validate the effectiveness of the proposed AWVDM.
更多查看译文
关键词
value difference metric,accurate class probability estimation,k-nearest neighbor algorithm,class membership probability,awvdm,good class probability estimator,pattern classification,class probability estimation,k-nearest neighbor,distance metrics,knn,class variable,attribute variable,attribute weighted value difference metric,attribute weighted value difference,data mining,classification,probability-based classifier,distance-related algorithm,attribute value,class probability estimation performance,probability,attribute weighting,proposed awvdm
AI 理解论文
溯源树
样例
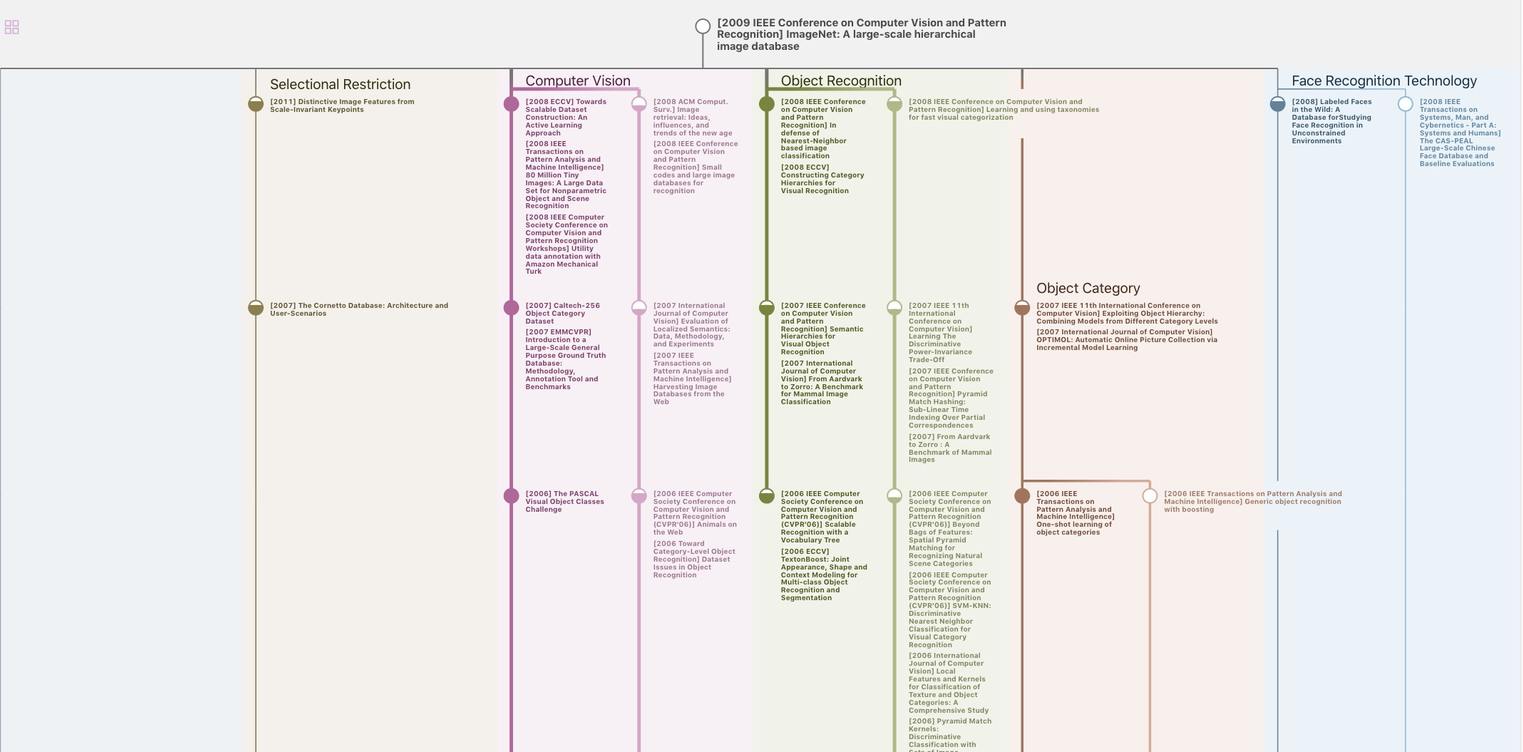
生成溯源树,研究论文发展脉络
Chat Paper
正在生成论文摘要