Accelerating Q-Learning through Kalman Filter Estimations Applied in a RoboCup SSL Simulation
Robotics Symposium and Competition(2013)
摘要
Speed of convergence in reinforcement learning methods represents an important problem, especially when the agent is interacting on adversarial environments like RoboCup Soccer domains. If the agent's learning rate is too small, then the algorithm needs too many iterations in order to successfully learn the task, and this would probably lead to lose the game before the agent has learnt its optimal policy. We attempt to overcome this problem by using partial state estimations when some of the involved dynamics are known or easy to model for accelerating Q-learning convergence, illustrating the results in a RoboCup SSL simulation.
更多查看译文
关键词
partial state estimation,robocup ssl simulation,q-learning convergence,involved dynamic,important problem,accelerating q-learning,robocup soccer domain,kalman filter estimations applied,adversarial environment,optimal policy,learning artificial intelligence,kalman filters,mobile robots
AI 理解论文
溯源树
样例
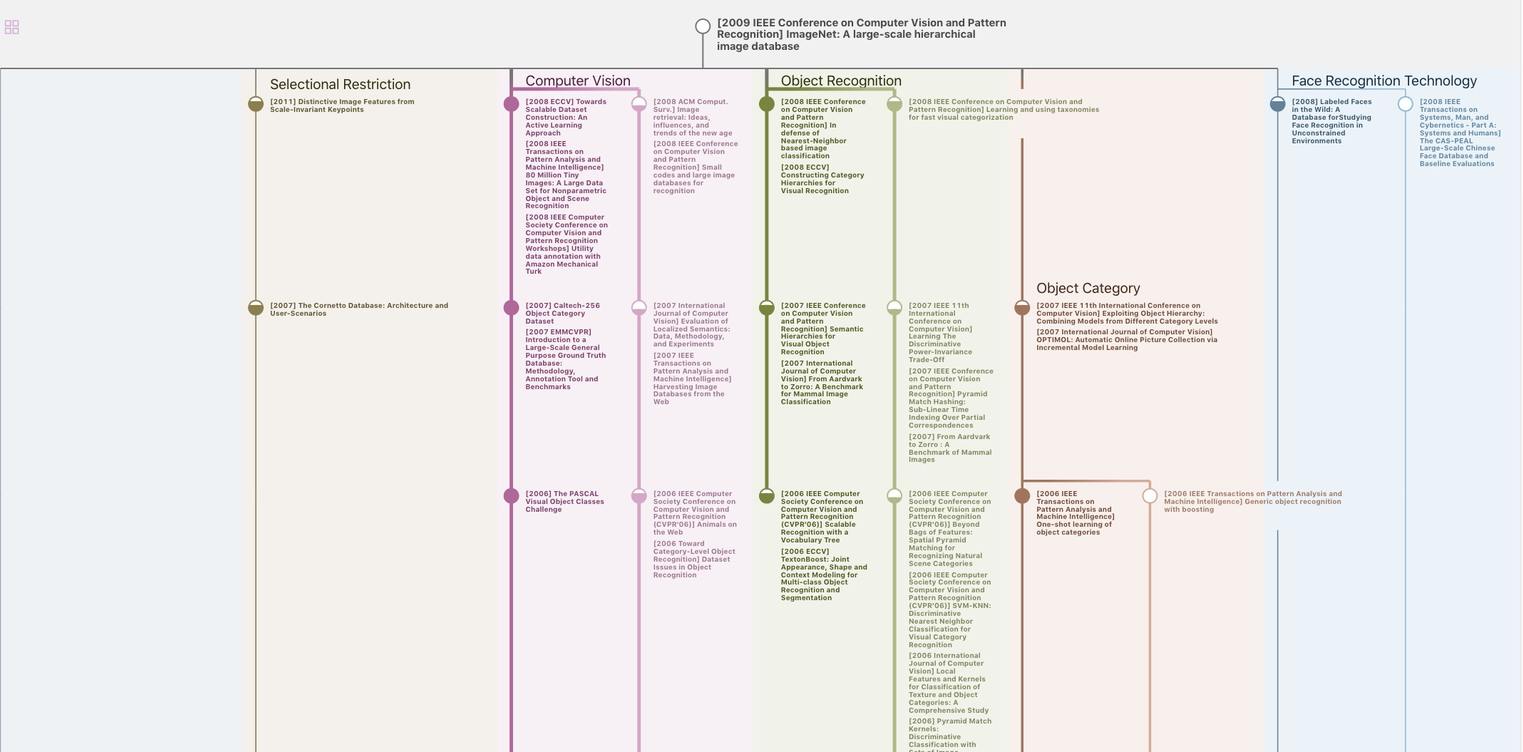
生成溯源树,研究论文发展脉络
Chat Paper
正在生成论文摘要