Physical activity recognition in free-living from body-worn sensors
SenseCam '13: Proceedings of the 4th International SenseCam & Pervasive Imaging Conference(2013)
摘要
Machine learning techniques are used to improve accelerometer-based measures of physical activity. Most studies have used laboratory-collected data to develop algorithms to classify behaviors, but studies of free-living activity are needed to improve the ecological validity of these methods. With this aim, we collected a novel free-living dataset that uses SenseCams to obtain ground-truth annotations of physical activities. We trained a classifier on free-living data and compare it to a classifier trained on prescribed activities. The classifier predicts five activity classes: bicycling, riding in a vehicle, sitting, standing, and walking/running. When testing on free-living data, classifiers trained on free-living data significantly outperform those trained on a controlled dataset (89.2% vs. 70.9% accuracy).
更多查看译文
关键词
physical activity recognition,body-worn sensor,gps,accelerometer,activity recognition
AI 理解论文
溯源树
样例
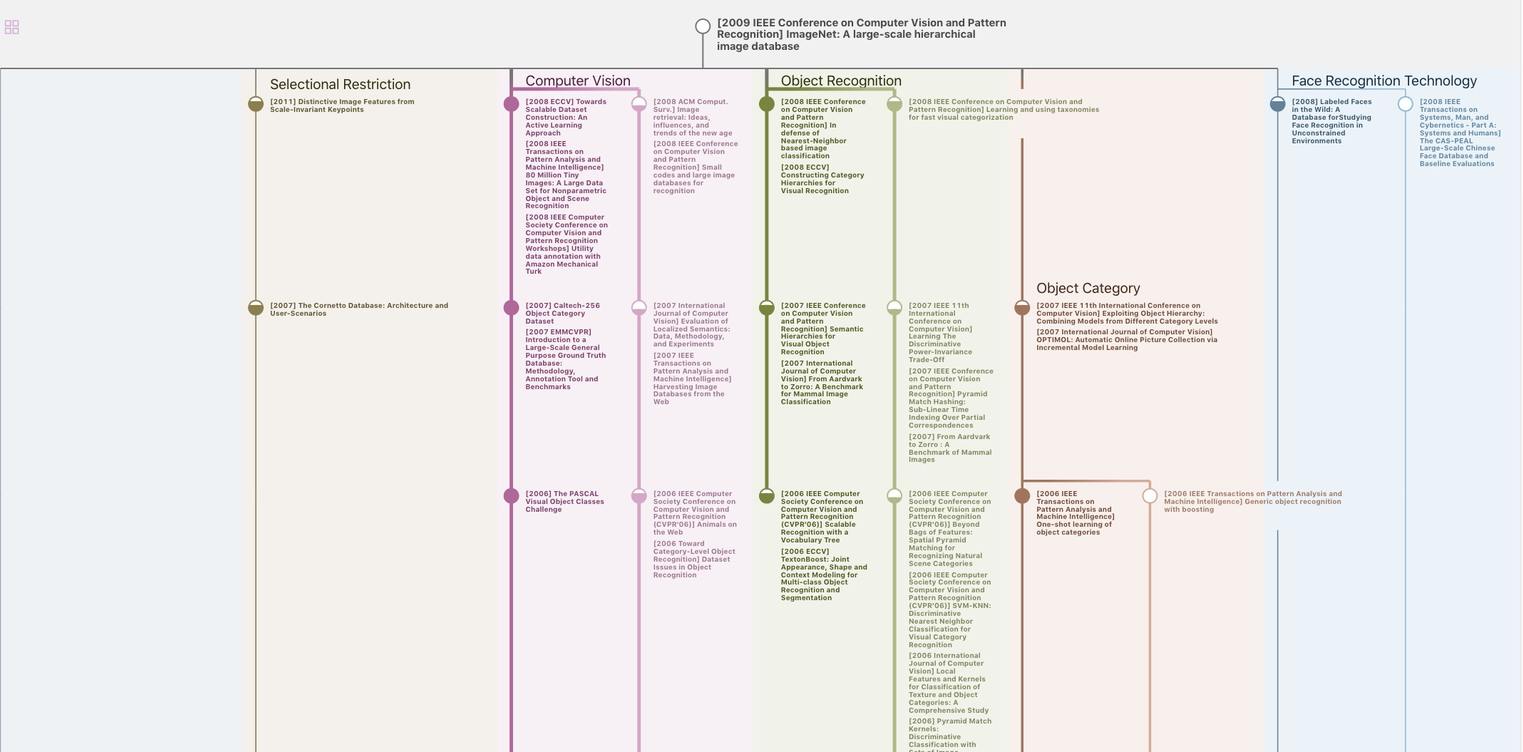
生成溯源树,研究论文发展脉络
Chat Paper
正在生成论文摘要