Boundary Detection Benchmarking: Beyond F-Measures
Computer Vision and Pattern Recognition(2013)
摘要
For an ill-posed problem like boundary detection, human labeled datasets play a critical role. Compared with the active research on finding a better boundary detector to refresh the performance record, there is surprisingly little discussion on the boundary detection benchmark itself. The goal of this paper is to identify the potential pitfalls of today's most popular boundary benchmark, BSDS 300. In the paper, we first introduce a psychophysical experiment to show that many of the "weak" boundary labels are unreliable and may contaminate the benchmark. Then we analyze the computation of f-measure and point out that the current benchmarking protocol encourages an algorithm to bias towards those problematic "weak" boundary labels. With this evidence, we focus on a new problem of detecting strong boundaries as one alternative. Finally, we assess the performances of 9 major algorithms on different ways of utilizing the dataset, suggesting new directions for improvements.
更多查看译文
关键词
boundary detection benchmarking,active research,strong boundary,ill-posed problem,new problem,beyond f-measures,better boundary detector,popular boundary benchmark,boundary detection benchmark,boundary label,new direction,boundary detection,computer vision,benchmarking,image segmentation,algorithm design and analysis,classification algorithms,reliability,benchmark testing,edge detection,f measure,detectors
AI 理解论文
溯源树
样例
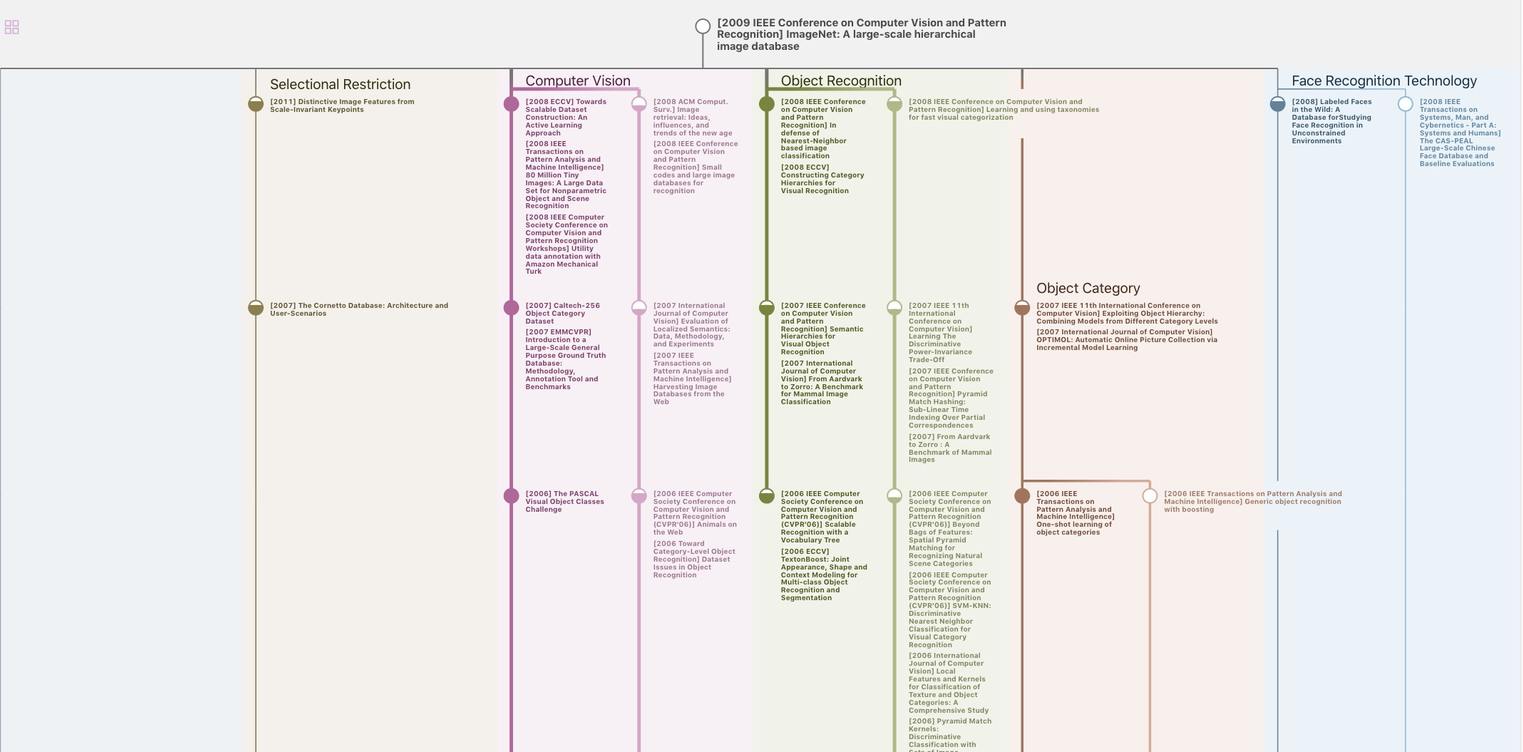
生成溯源树,研究论文发展脉络
Chat Paper
正在生成论文摘要