Dynamic Scene Classification: Learning Motion Descriptors with Slow Features Analysis
Computer Vision and Pattern Recognition(2013)
摘要
In this paper, we address the challenging problem of categorizing video sequences composed of dynamic natural scenes. Contrarily to previous methods that rely on handcrafted descriptors, we propose here to represent videos using unsupervised learning of motion features. Our method encompasses three main contributions: 1) Based on the Slow Feature Analysis principle, we introduce a learned local motion descriptor which represents the principal and more stable motion components of training videos. 2) We integrate our local motion feature into a global coding/pooling architecture in order to provide an effective signature for each video sequence. 3) We report state of the art classification performances on two challenging natural scenes data sets. In particular, an outstanding improvement of 11\% in classification score is reached on a data set introduced in 2012.
更多查看译文
关键词
feature extraction,image classification,image motion analysis,image representation,image sequences,natural scenes,unsupervised learning,video signal processing,dynamic natural scene classification,global coding-pooling architecture,handcrafted descriptor,learning motion descriptor,motion component,slow feature analysis principle,training video,unsupervised learning,video representation,video sequence categorization,Motion descriptors,Slow Feature Analysis,Unsupervised learning,Video classification
AI 理解论文
溯源树
样例
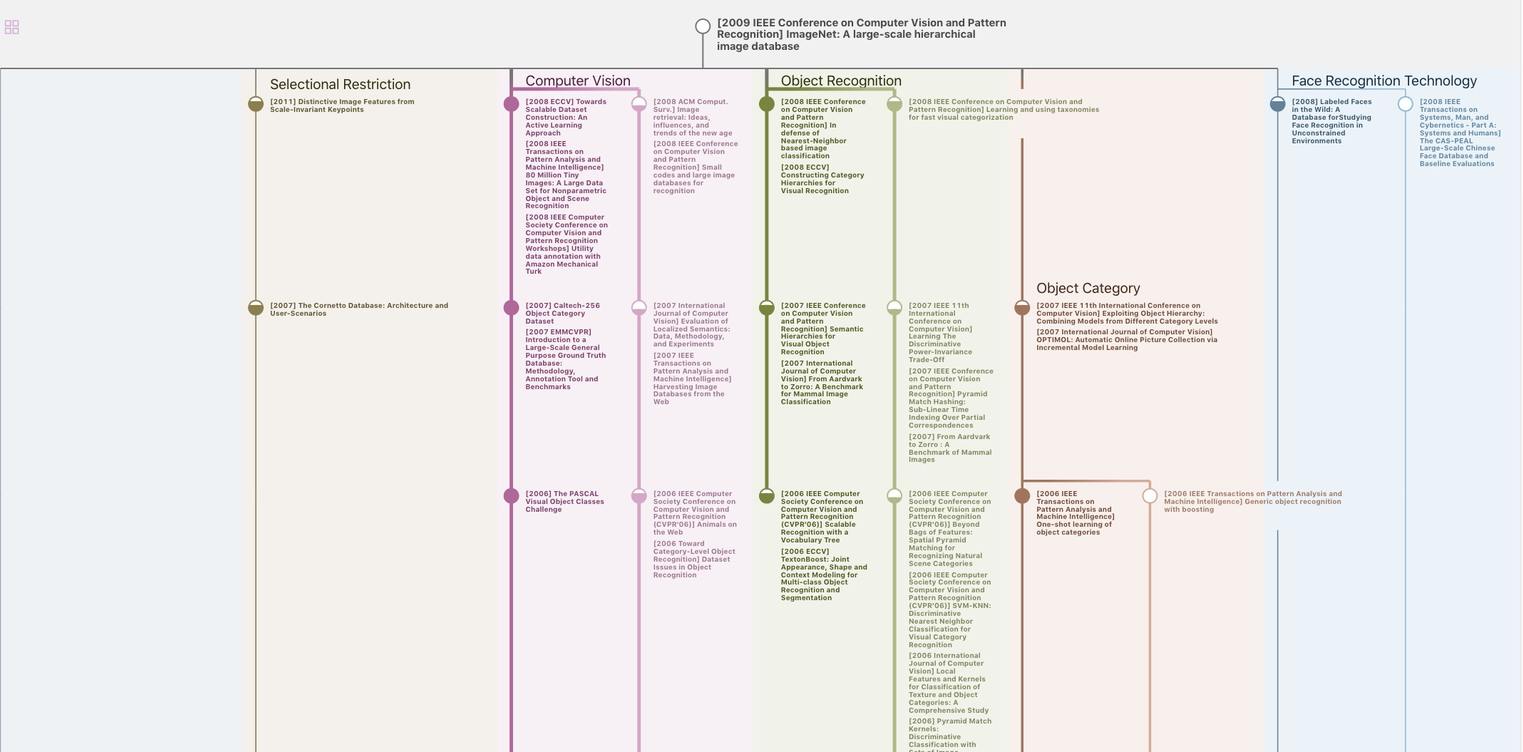
生成溯源树,研究论文发展脉络
Chat Paper
正在生成论文摘要