Using file relationships in malware classification
DIMVA(2012)
摘要
Typical malware classification methods analyze unknown files in isolation. However, this ignores valuable relationships between malware files, such as containment in a zip archive, dropping, or downloading. We present a new malware classification system based on a graph induced by file relationships, and, as a proof of concept, analyze containment relationships, for which we have much available data. However our methodology is general, relying only on an initial estimate for some of the files in our data and on propagating information along the edges of the graph. It can thus be applied to other types of file relationships. We show that since malicious files are often included in multiple malware containers, the system's detection accuracy can be significantly improved, particularly at low false positive rates which are the main operating points for automated malware classifiers. For example at a false positive rate of 0.2%, the false negative rate decreases from 42.1% to 15.2%. Finally, the new system is highly scalable; our basic implementation can learn good classifiers from a large, bipartite graph including over 719 thousand containers and 3.4 million files in a total of 16 minutes.
更多查看译文
关键词
low false positive rate,typical malware classification method,multiple malware container,false negative rate decrease,bipartite graph,new malware classification system,malware file,file relationship,false positive rate,automated malware classifier,machine learning
AI 理解论文
溯源树
样例
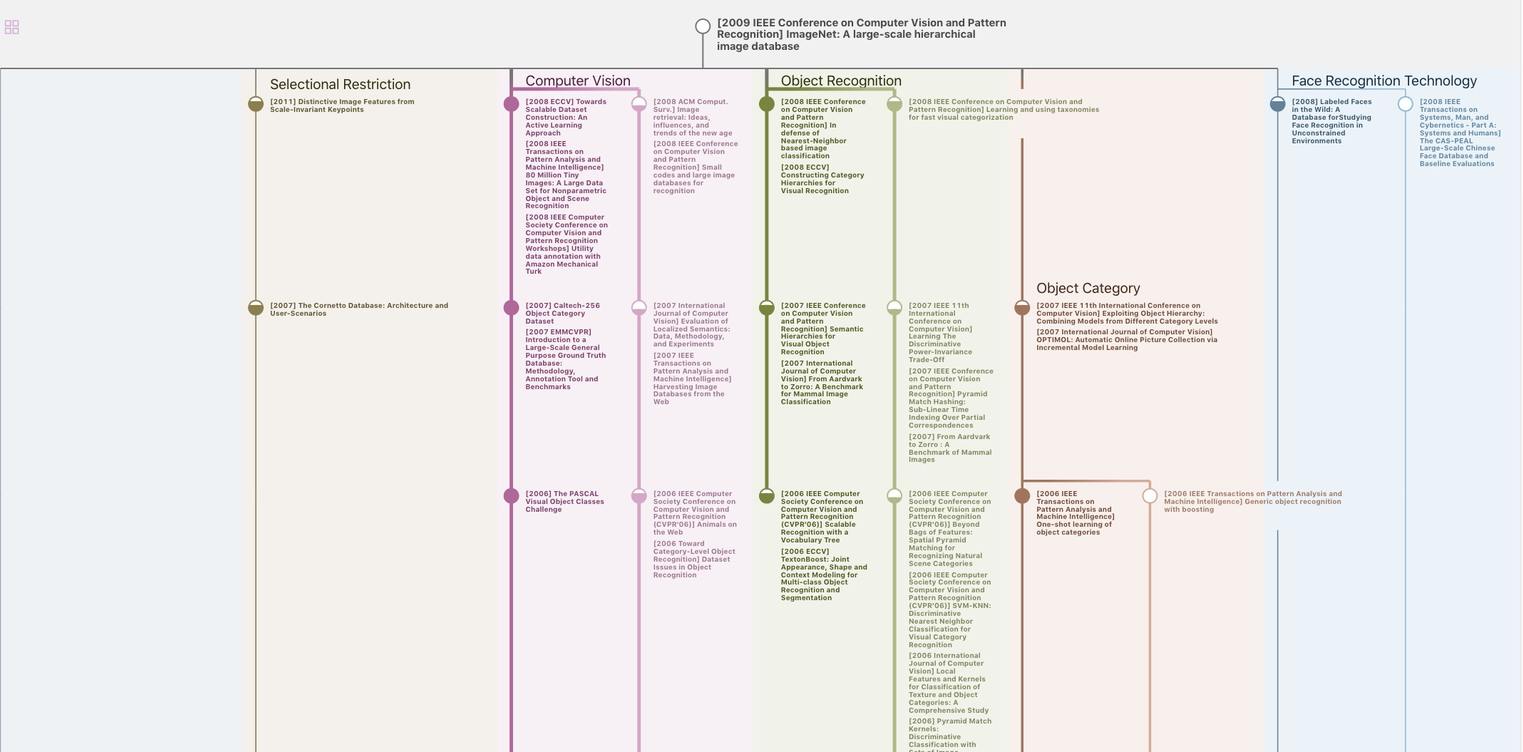
生成溯源树,研究论文发展脉络
Chat Paper
正在生成论文摘要