Comparing multiobjective swarm intelligence metaheuristics for DNA motif discovery
Engineering Applications of Artificial Intelligence(2013)
摘要
In recent years, a huge number of biological problems have been successfully addressed through computational techniques, among all these computational techniques we highlight metaheuristics. Also, most of these biological problems are directly related to genomic, studying the microorganisms, plants, and animals genomes. In this work, we solve a DNA sequence analysis problem called Motif Discovery Problem (MDP) by using two novel algorithms based on swarm intelligence: Artificial Bee Colony (ABC) and Gravitational Search Algorithm (GSA). To guide the pattern search to solutions that have a better biological relevance, we have redefined the problem formulation and incorporated several biological constraints that should be satisfied by each solution. One of the most important characteristics of the problem definition is the application of multiobjective optimization (MOO), maximizing three conflicting objectives: motif length, support, and similarity. So, we have adapted our algorithms to the multiobjective context. This paper presents an exhaustive comparison of both multiobjective proposals on instances of different nature: real instances, generic instances, and instances generated according to a Markov chain. To analyze their operations we have used several indicators and statistics, comparing their results with those obtained by standard algorithms in multiobjective computation, and by 14 well-known biological methods.
更多查看译文
关键词
multiobjective swarm intelligence metaheuristics,multiobjective context,dna sequence analysis problem,multiobjective computation,biological relevance,dna motif discovery,well-known biological method,multiobjective proposal,biological problem,multiobjective optimization,computational technique,biological constraint,swarm intelligence
AI 理解论文
溯源树
样例
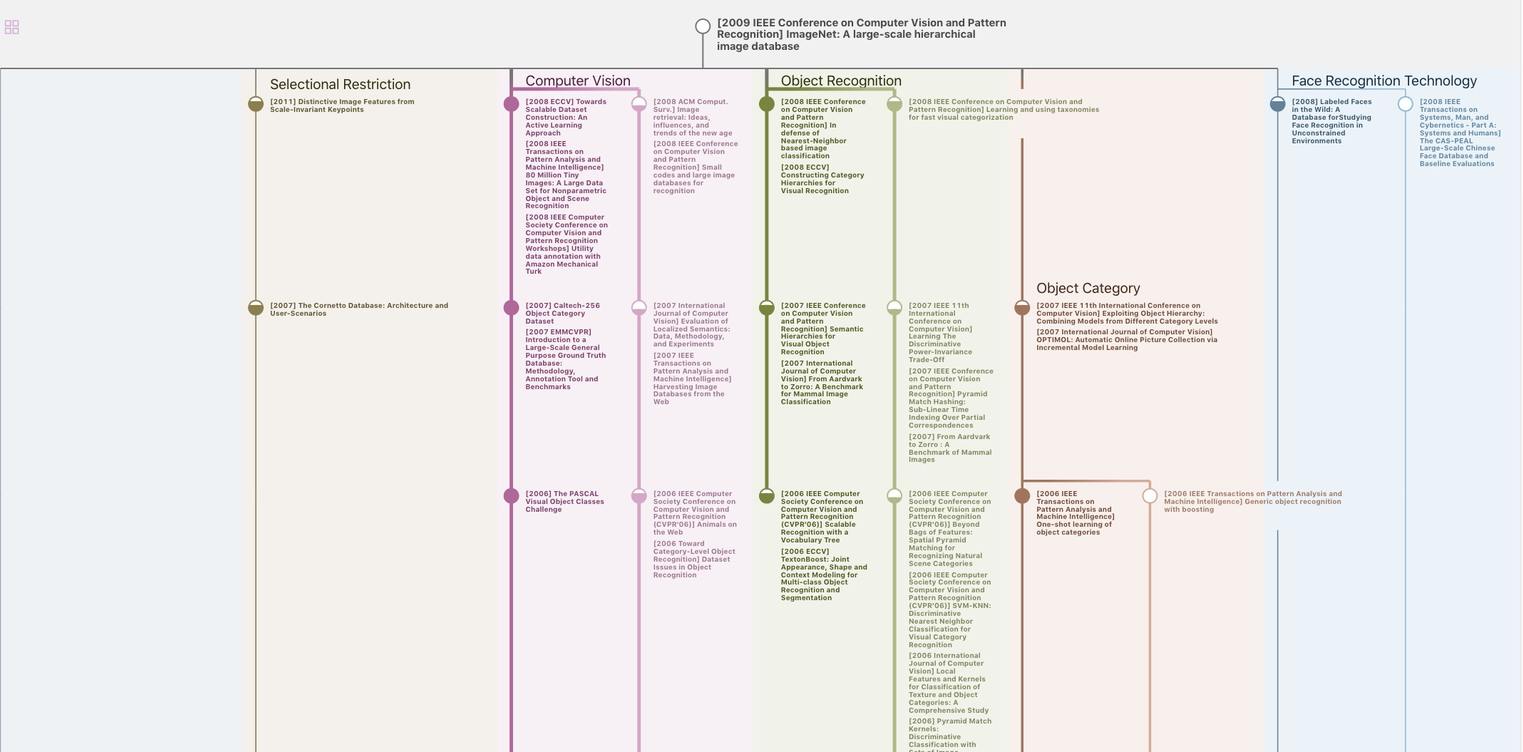
生成溯源树,研究论文发展脉络
Chat Paper
正在生成论文摘要