A heuristic for non-convex variance-based clustering criteria
SEA'12 Proceedings of the 11th international conference on Experimental Algorithms(2012)
摘要
We address the clustering problem in the context of exploratory data analysis, where data sets are investigated under different and desirably contrasting perspectives. In this scenario where, for flexibility, solutions are evaluated by criterion functions, we introduce and evaluate a generalized and efficient version of the incremental one-by-one clustering algorithm of MacQueen (1967). Unlike the widely adopted two-phase algorithm developed by Lloyd (1957), our approach does not rely on the gradient of the criterion function being optimized, offering the key advantage of being able to deal with non-convex criteria. After an extensive experimental analysis using real-world data sets with a more flexible, non-convex criterion function, we obtained results that are considerably better than those produced with the k-means criterion, making our algorithm an invaluable tool for exploratory clustering applications.
更多查看译文
关键词
exploratory data analysis,exploratory clustering application,k-means criterion,non-convex criterion,two-phase algorithm,real-world data set,non-convex variance-based clustering criterion,non-convex criterion function,clustering problem,criterion function
AI 理解论文
溯源树
样例
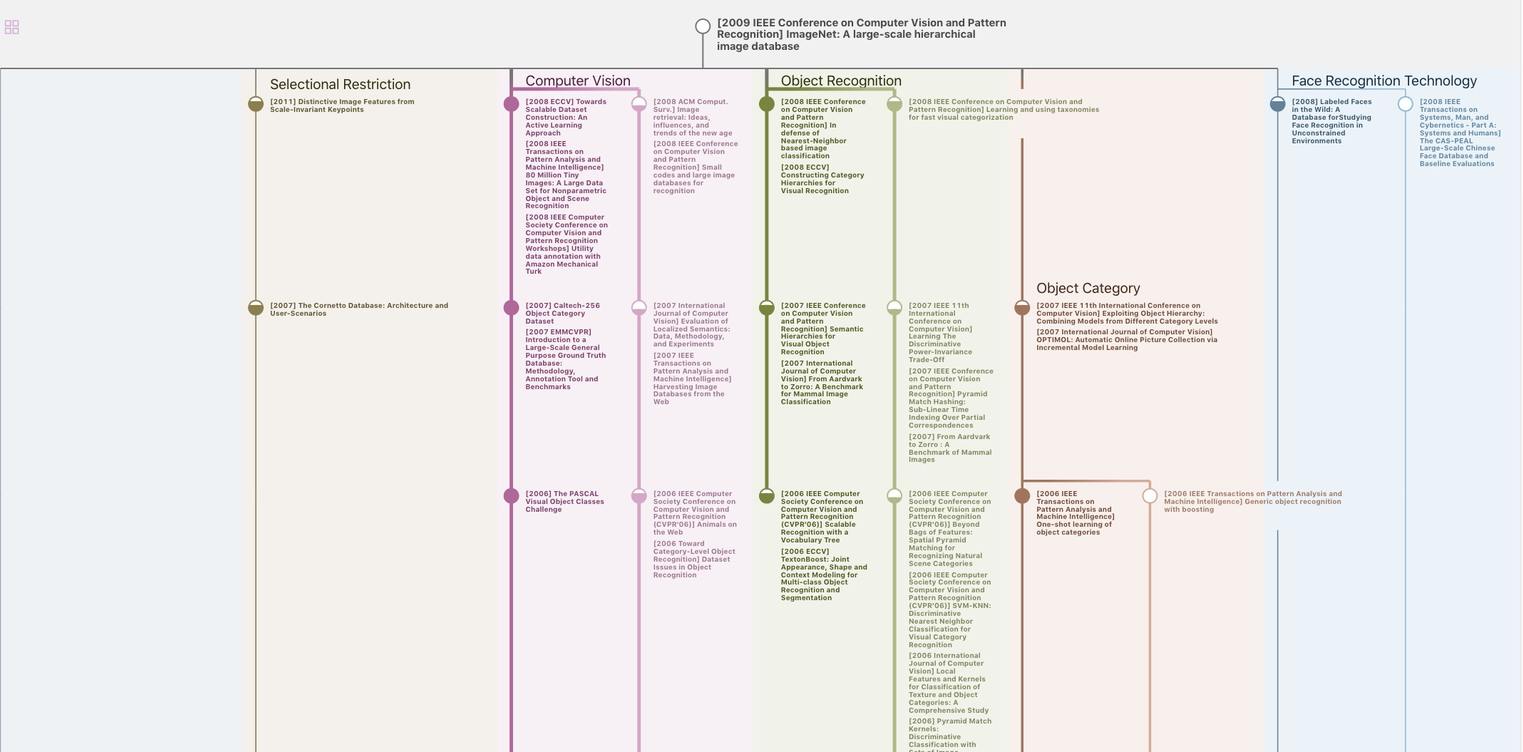
生成溯源树,研究论文发展脉络
Chat Paper
正在生成论文摘要