Protein-Protein interaction affinity prediction based on interface descriptors and machine learning
INTELLIGENT COMPUTING THEORIES AND APPLICATIONS, ICIC 2012(2012)
摘要
Knowing the protein-protein interaction affinity is important for accurately inferring the time dimensionality of the dynamic protein-protein interaction networks from a viewpoint of systems biology. The accumulation of the determined protein complex structures with high resolution facilitates to realize this ambitious goal. Previous methods on protein-protein interaction affinity (PPIA) prediction have achieved great success. However, there is still a great space to improve prediction accuracy. Here, we develop a support vector regression method to infer highly heterogeneous protein-protein interaction affinities based on interface properties. This method takes full advantage of the labels of the interaction pairs and greatly reduces the dimensionality of the input features. Results show that the supervised machine leaning methods are effective with R=0.80 and SD=1.41 and perform well when applied to the prediction of highly heterogeneous or generic PPIA. Comparison of different types of interface properties shows that the global interface properties have a more stable performance while the smoothed PMF obtains the best prediction accuracy.
更多查看译文
关键词
protein-protein interaction affinity,protein-protein interaction affinity prediction,prediction accuracy,dynamic protein-protein interaction network,best prediction accuracy,machine learning,interface descriptors,generic ppia,interaction pair,global interface property,interface property,great space,heterogeneous protein-protein interaction affinity,potential of mean force
AI 理解论文
溯源树
样例
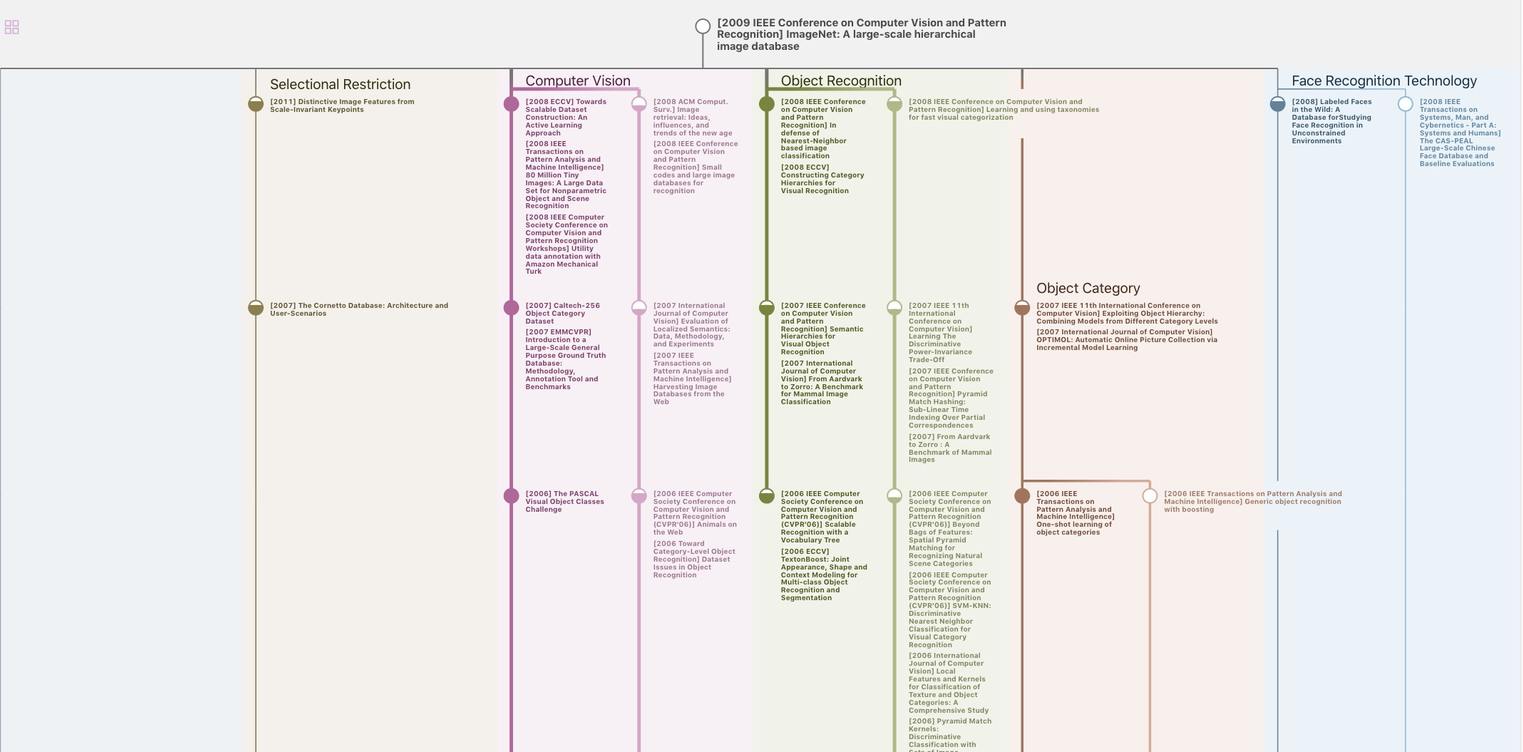
生成溯源树,研究论文发展脉络
Chat Paper
正在生成论文摘要