Discovering K web user groups with specific aspect interests
MLDM(2012)
摘要
Online review analysis becomes a hot research topic recently. Most of the existing works focus on the problems of review summarization, aspect identification or opinion mining from an item's point of view such as the quality and popularity of products. Considering the fact that authors of these review texts may pay different attentions to different domain-based product aspects with respect to their own interests, in this paper, we aim to learn K user groups with specific aspect interests indicated by their review writings. Such K user groups' identification can facilitate better understanding of customers' interests which are crucial for application like product improvement on customer-oriented design or diverse marketing strategies. Instead of using a traditional text clustering approach, we treat the clusterId as a hidden variable and use a permutation-based structural topic model called KMM. Through this model, we infer K groups' distribution by discovering not only the frequency of reviewers' product aspects, but also the occurrence priority of respective aspects. Our experiment on several real-world review datasets demonstrates a competitive solution.
更多查看译文
关键词
product improvement,review summarization,different domain-based product aspect,discovering k web user,k group,product aspect,k user group,review writing,review text,online review analysis,specific aspect interest,real-world review datasets
AI 理解论文
溯源树
样例
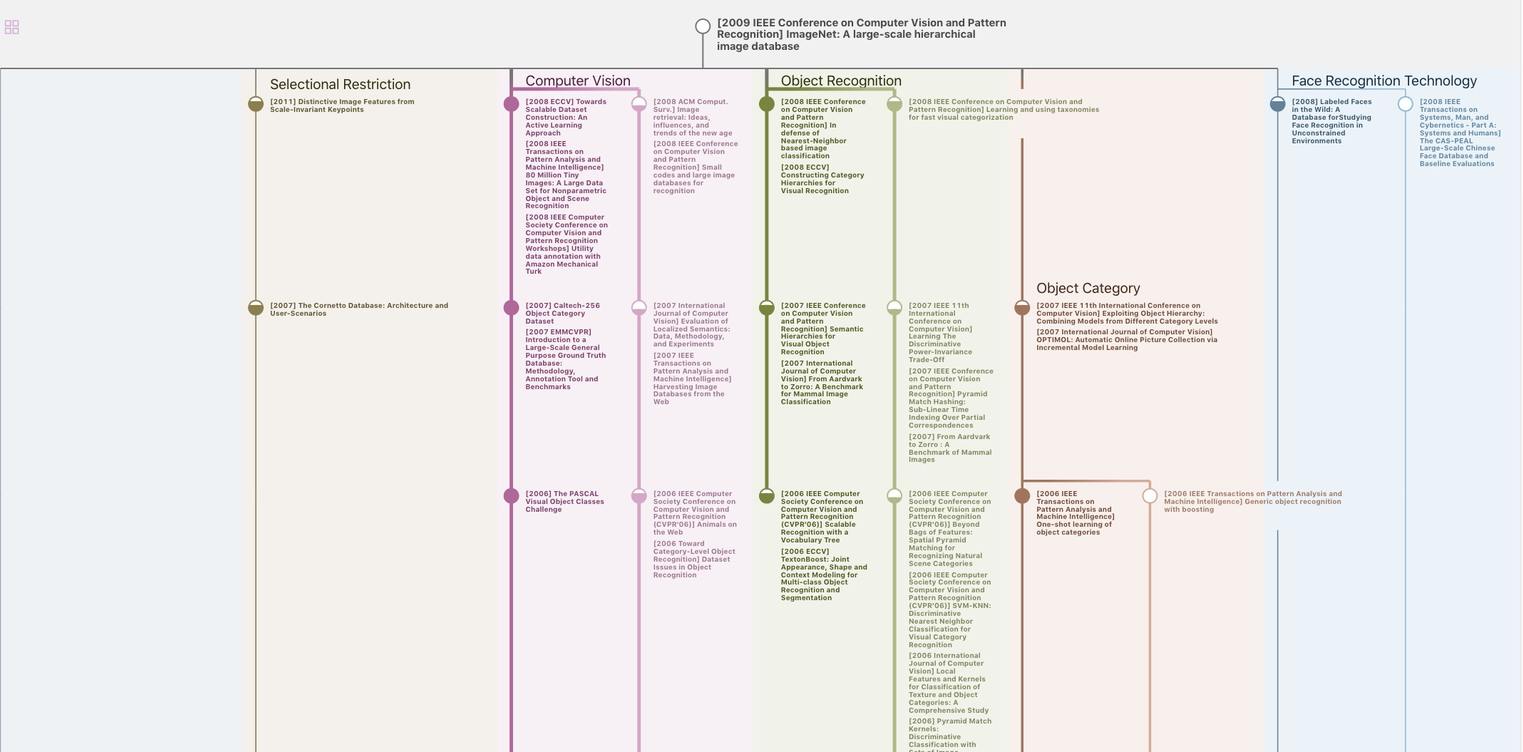
生成溯源树,研究论文发展脉络
Chat Paper
正在生成论文摘要