Semi-unsupervised weighted maximum-likelihood estimation of joint densities for the co-training of adaptive activation functions
PSL'11 Proceedings of the First IAPR TC3 conference on Partially Supervised Learning(2011)
摘要
The paper presents an explicit maximum-likelihood algorithm for the estimation of the probabilistic-weighting density functions that are associated with individual adaptive activation functions in neural networks. A partially unsupervised technique is devised which takes into account the joint distribution of input features and target outputs. Combined with the training algorithm introduced in the companion paper [2], the solution proposed herein realizes a well-defined, specific instance of the novel learning machine. The extension of the overall training method to more-than-one hidden layer architectures is pointed out, as well. A preliminary experimental demonstration is given, outlining how the algorithm works.
更多查看译文
关键词
input feature,joint density,companion paper,neural network,training algorithm,joint distribution,hidden layer architecture,overall training method,algorithm work,semi-unsupervised weighted maximum-likelihood estimation,individual adaptive activation function,explicit maximum-likelihood algorithm,expectation maximization
AI 理解论文
溯源树
样例
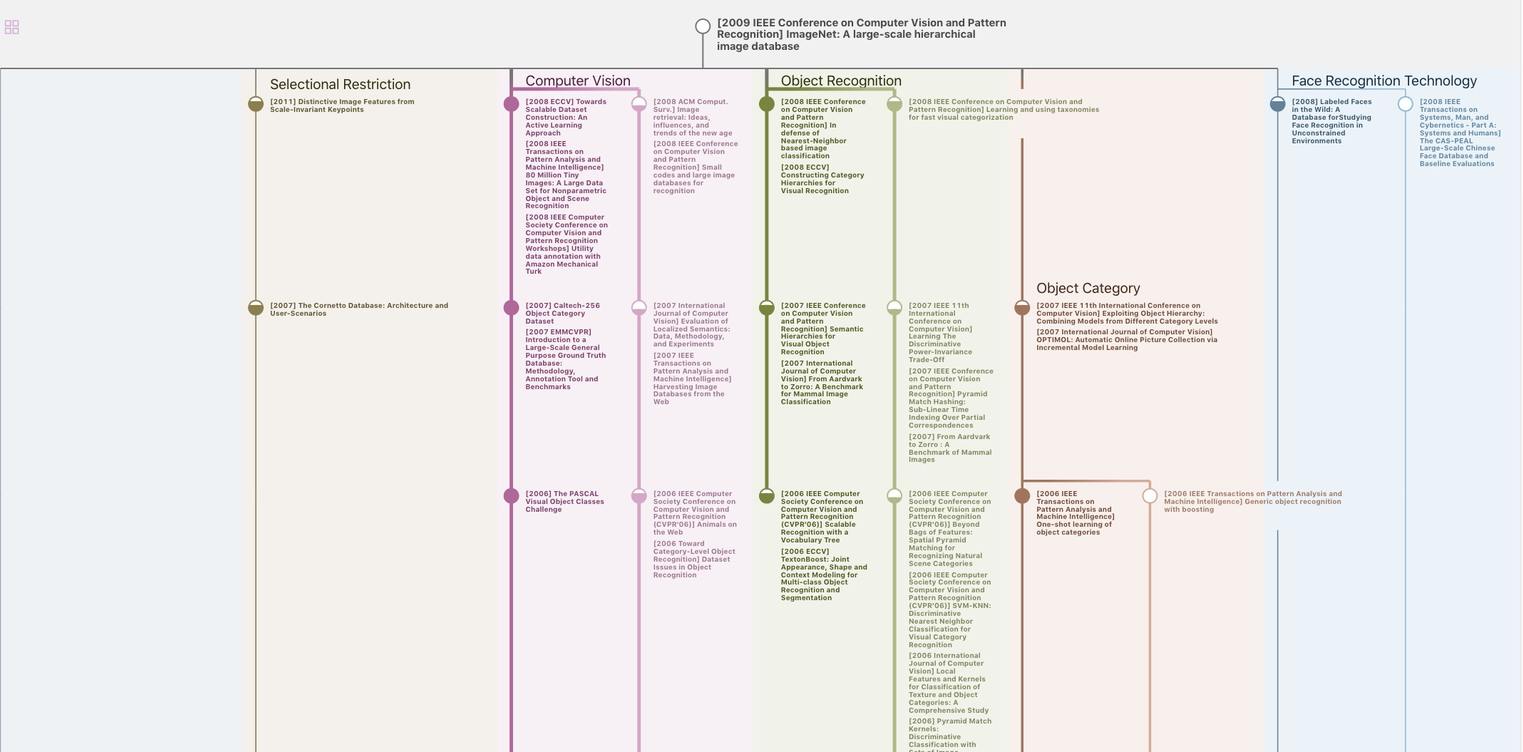
生成溯源树,研究论文发展脉络
Chat Paper
正在生成论文摘要