A novel connectionist-oriented feature normalization technique
ARTIFICIAL NEURAL NETWORKS - ICANN 2006, PT 2(2006)
摘要
Feature normalization is a topic of practical relevance in real-world applications of neural networks. Although the topic is sometimes overlooked, the success of connectionist models in difficult tasks may depend on a proper normalization of input features. As a matter of fact, the relevance of normalization is pointed out in classic pattern recognition literature. In addition, neural nets require input values that do not compromise numerical stability during the computation of partial derivatives of the nonlinearities. For instance, inputs to connectionist models should not exceed certain ranges, in order to avoid the phenomenon of “saturation” of sigmoids. This paper introduces a novel feature normalization technique that ensures values that are distributed over the (0,1) interval in a uniform manner. The normalization is obtained starting from an estimation of the probabilistic distribution of input features, followed by an evaluation (over the feature that has to be normalized) of a “mixture of Logistics” approximation of the cumulative distribution. The approach turns out to be compliant with the very nature of the neural network (it is realized via a mixture of sigmoids, that can be encapsulated within the network itself). Experiments on a real-world continuous speech recognition task show that the technique is effective, comparing favorably with some standard feature normalizations.
更多查看译文
关键词
novel connectionist-oriented feature normalization,input feature,classic pattern recognition literature,neural network,novel feature normalization technique,proper normalization,feature normalization,input value,neural net,standard feature normalization,connectionist model,pattern recognition,numerical stability
AI 理解论文
溯源树
样例
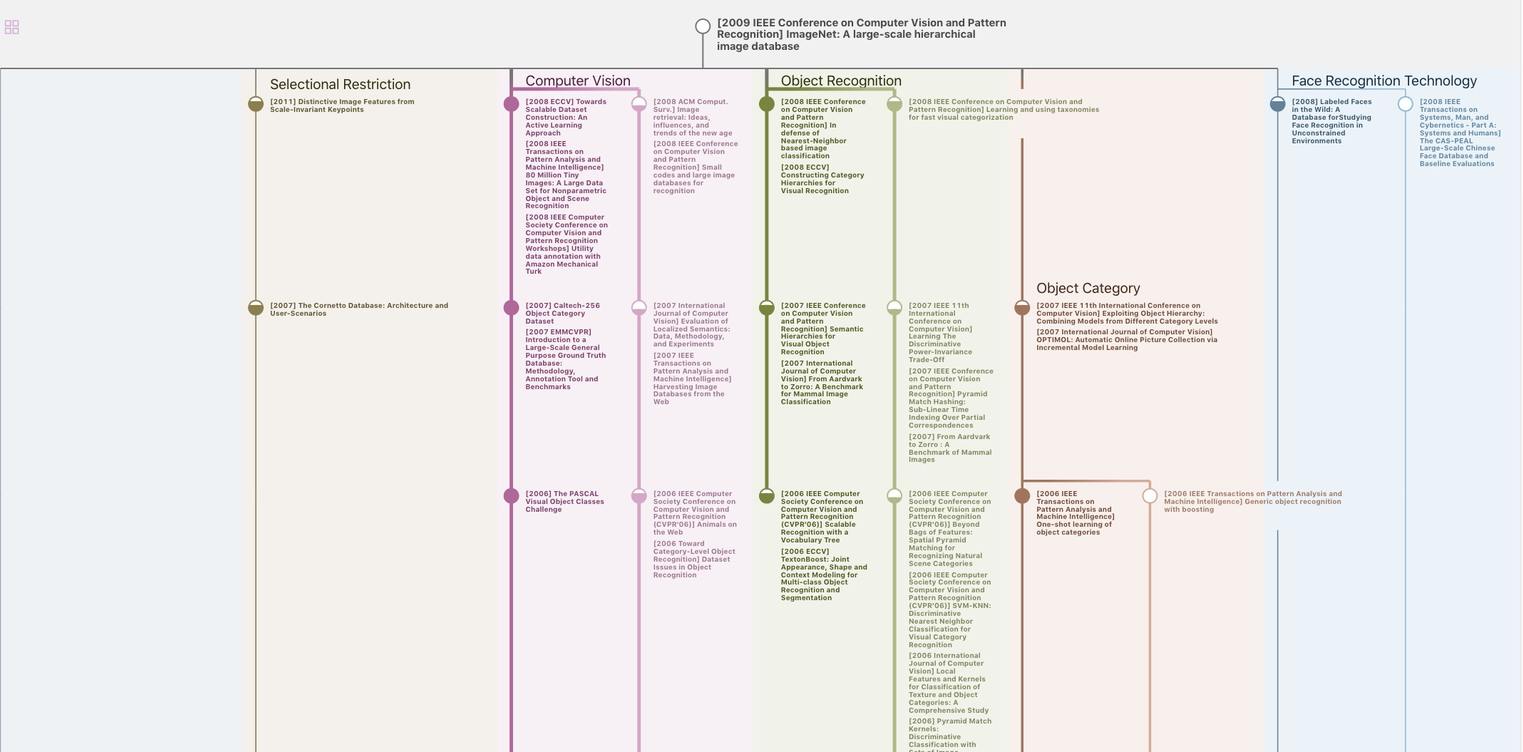
生成溯源树,研究论文发展脉络
Chat Paper
正在生成论文摘要