A PSO Accelerated Immune Particle Filter for Dynamic State Estimation
Computer and Robot Vision(2011)
摘要
Particle Filter (PF) is a flexible and powerful Sequential Monte Carlo (SMC) technique to solve the nonlinear state/parameter estimation problems. The generic PF suffers due to degeneracy or sample impoverishment, which adversely affects its performance. In order to overcome this issue of the generic PF, a Particle Swarm Optimization accelerated Immune Particle Filter (PSO-acc-IPF) is proposed in this work. It combines the robustness and the diversified search capability of the Immune Algorithm (IA) and the speed and the computational efficiency of the Particle Swarm Optimization (PSO) in pursuing the global optimal solution. Mutation plays the key role in the proposed algorithm to help avoid the local optima and search for a global best solution. A two stage mutation operation is proposed. The first stage, with a high mutation rate, helps in exploring a larger solution space and the second stage, with a smaller mutation rate, helps in local optimal search. Later on, PSO is employed to accelerate the convergence speed. To validate the effectiveness of the proposed algorithm, its performance is compared with the generic PF and PSO Particle Filter (PSO-PF). The simulation results have demonstrated better robustness in state estimation for switching dynamic systems.
更多查看译文
关键词
dynamic state estimation,diversified search capability,immune particle filter,particle swarm optimization,pso particle filter,pso accelerated immune particle,stage mutation operation,particle filter,generic pf,smaller mutation rate,high mutation rate,proposed algorithm,artificial immune system,immune system,dynamic system,monte carlo methods,parameter estimation,sequential monte carlo,global optimization,artificial immune systems,soft computing,mutation rate,cloning,mathematical model,particle filters
AI 理解论文
溯源树
样例
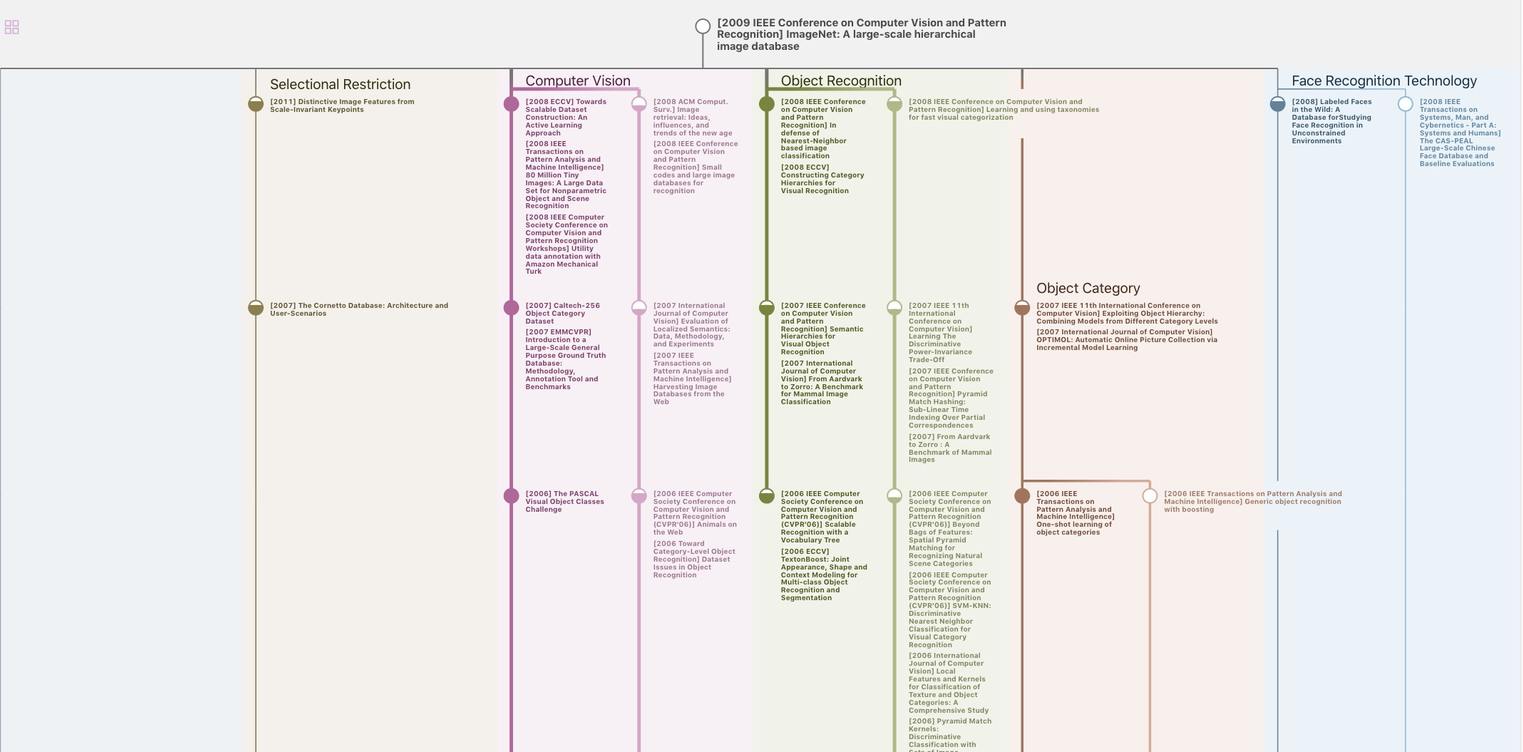
生成溯源树,研究论文发展脉络
Chat Paper
正在生成论文摘要