Feature Extraction for Simple Classification
Pattern Recognition(2010)
摘要
Constructing a recognition system based on raw measurements for different objects usually requires expert knowledge of domain specific data preprocessing, feature extraction, and classifier design. We seek to simplify this process in a way that can be applied without any knowledge about the data domain and the specific properties of different classification algorithms. That is, a recognition system should be simple to construct and simple to operate in practical applications. For this, we have developed a nonlinear feature extractor for high-dimensional complex patterns, using Deep Neural Networks (DNN). Trained partly supervised and unsupervised, the DNN effectively implements a nonlinear discriminant analysis based on a Fisher criterion in a feature space of very low dimensions. Our experiments show that the automatically extracted features work very well with simple linear discriminants, while the recognition rates improve only minimally if more sophisticated classification algorithms like Support Vector Machines (SVM) are used instead.
更多查看译文
关键词
nonlinear feature extractor,simple classification,simple linear discriminants,feature extraction,different classification algorithm,recognition system,data domain,recognition rate,domain specific data,different object,feature space,artificial neural networks,discriminant analysis,markov processes,neural network,restricted boltzmann machines,support vector machines,data preprocessing,support vector machine,object recognition,databases,boltzmann machine,pattern recognition,neural nets
AI 理解论文
溯源树
样例
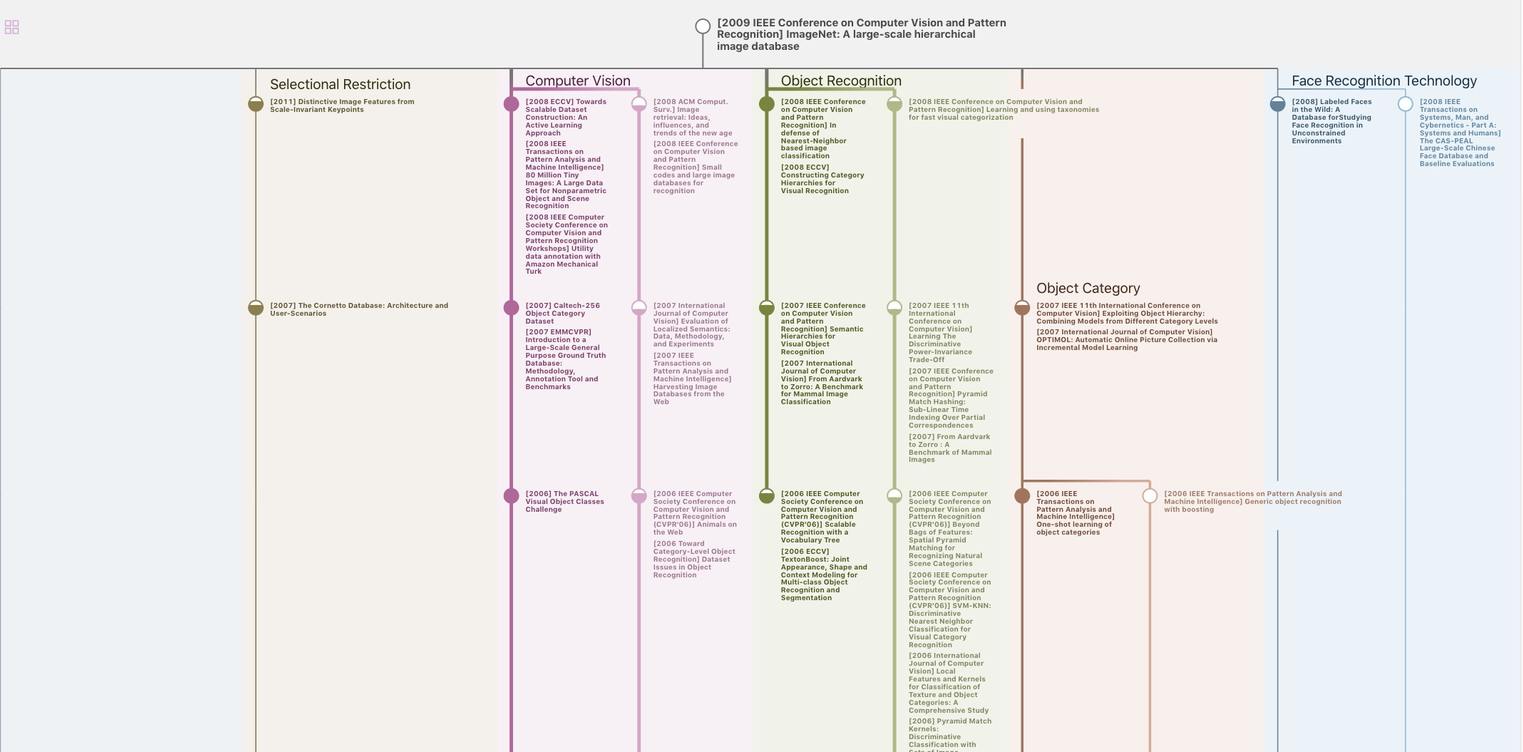
生成溯源树,研究论文发展脉络
Chat Paper
正在生成论文摘要