Improving Image Annotation In Imbalanced Classification Problems With Ranking Svm
CLEF'09 Proceedings of the 10th international conference on Cross-language evaluation forum: multimedia experiments(2010)
摘要
We try to overcome the imbalanced data set problem in image annotation by choosing a convenient loss function for learning the classifier. Instead of training a standard SVM, we use a Ranking SVM in which the chosen loss function is helpful in the case of imbalanced data. We compare the Ranking SVM to a classical SVM with different visual features. We observe that Ranking SVM always improves the prediction quality, and can perform up to 23% better than the classical SVM.
更多查看译文
关键词
Visual Feature, Image Annotation, Equal Error Rate, Ranking Algorithm, Imbalanced Data
AI 理解论文
溯源树
样例
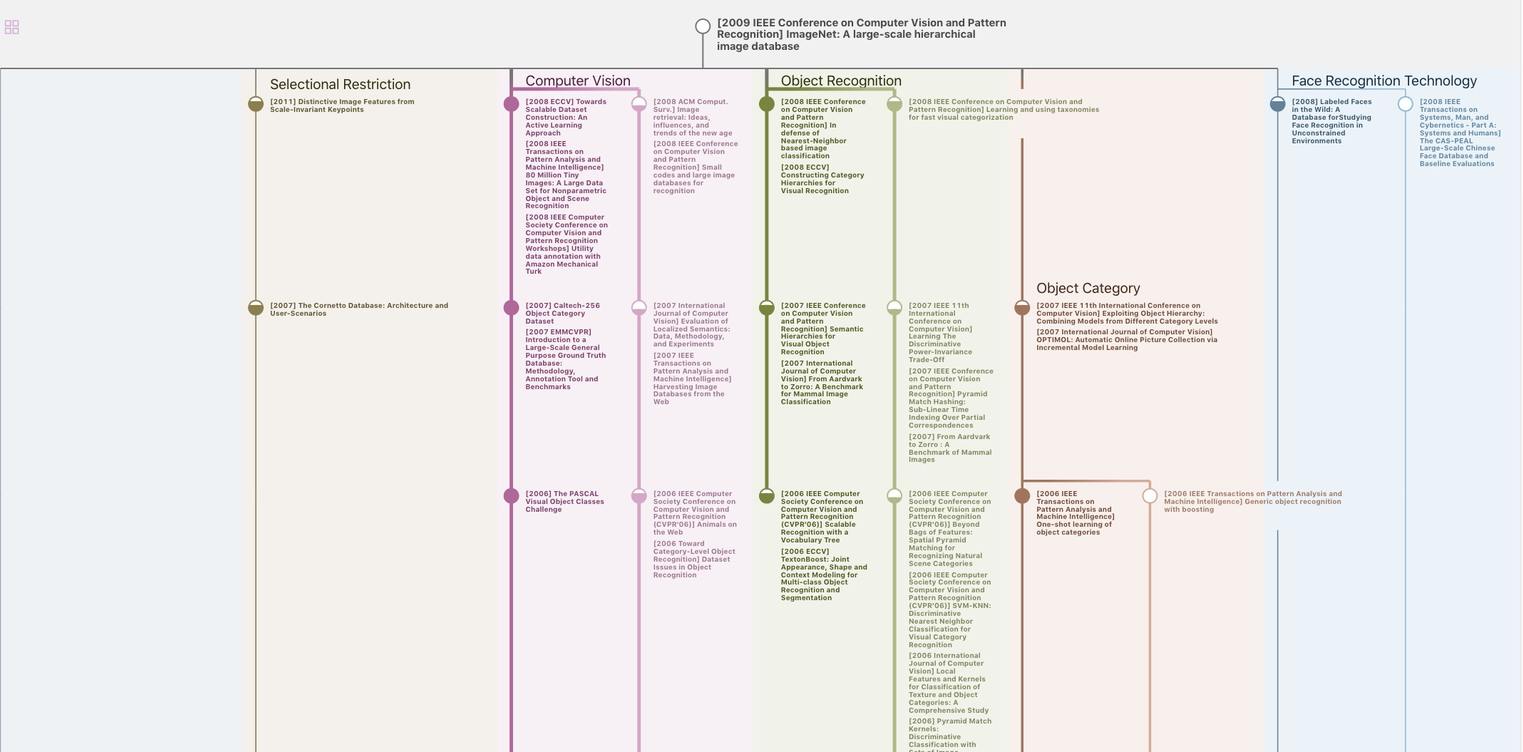
生成溯源树,研究论文发展脉络
Chat Paper
正在生成论文摘要