Review-Based Ranking of Wikipedia Articles
Fontainbleu(2009)
摘要
Wikipedia, the largest encyclopedia on the Web, is often seen as the most successful example of crowdsourcing. The encyclopedic knowledge it accumulated over the years is so large that one often uses search engines, to find information in it. In contrast to regular Web pages, Wikipedia is fairly structured, and articles are usually accompanied with history pages, categories and talk pages. The meta-data available in these pages can be analyzed to gain a better understanding of the content and quality of the articles. We discuss how the rich meta-data available in wiki pages can be used to provide better search results in Wikipedia. Built on the studies on "Wisdom of Crowds" and the effectiveness of the knowledge collected by a large number of people, we investigate the effect of incorporating the extent of review of an article in the quality of rankings of the search results. The extent of review is measured by the number of distinct editors contributed to the articles and is extracted by processing Wikipedia's history pages. We compare different ranking algorithms that explore combinations of text-relevancy, PageRank, and extent of review. The results show that the review-based ranking algorithm which combines the extent of review and text-relevancy outperforms the rest; it is more accurate and less computationally expensive compared to PageRank-based rankings.
更多查看译文
关键词
wikipedia articles,encyclopedic knowledge,review-based ranking,better search result,better understanding,different ranking algorithm,large number,history page,pagerank-based ranking,search engine,regular web page,search result,meta data,electronic publishing,search engines,encyclopedias,informatics,internet,ranking,data mining,feature extraction,java,wikipedia,computer networks,history,computer science,web pages
AI 理解论文
溯源树
样例
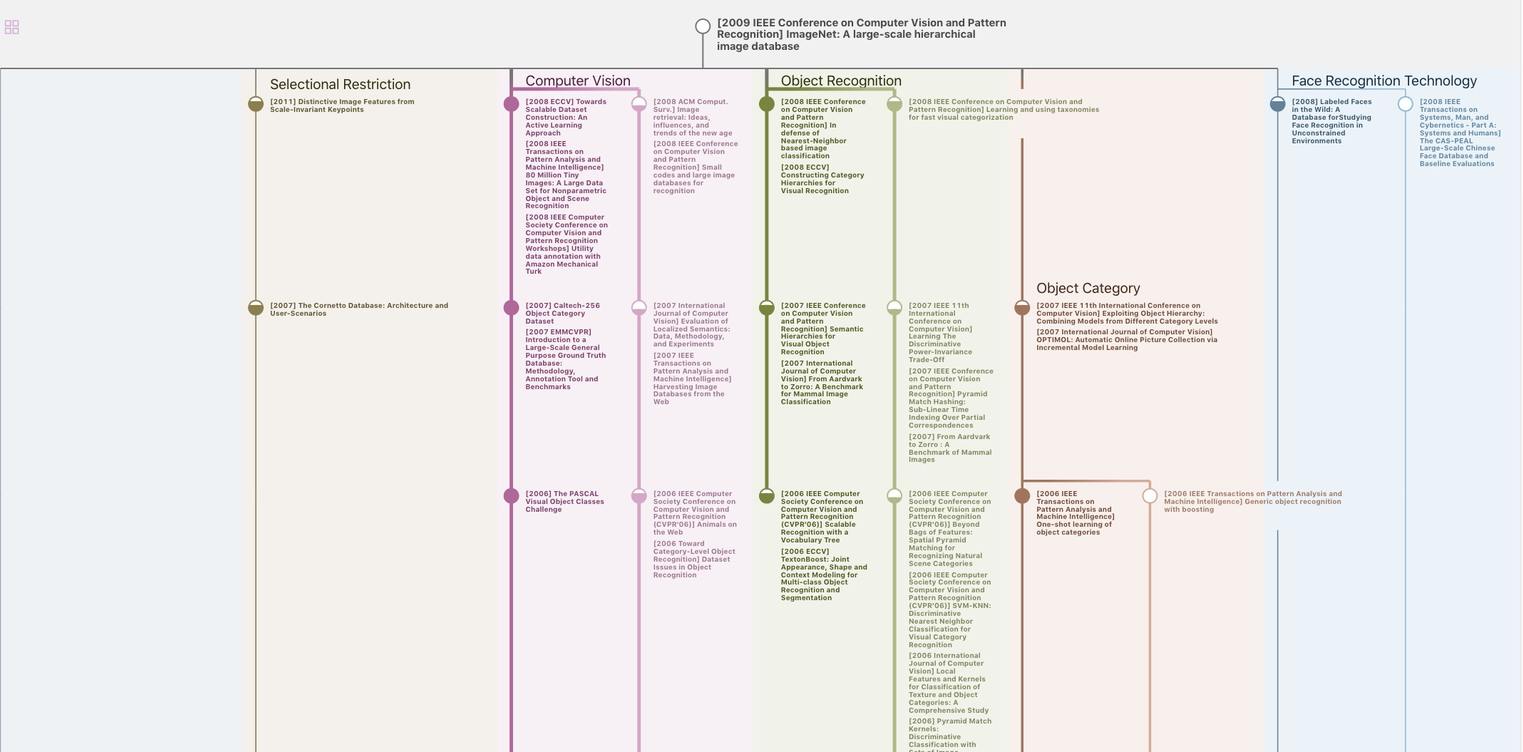
生成溯源树,研究论文发展脉络
Chat Paper
正在生成论文摘要