TransRank: A Novel Algorithm for Transfer of Rank Learning
Pisa(2008)
摘要
Recently, learning to rank technique has attracted much attention. However, the lack of labeled training data seriously limits its application in real-world tasks. In this paper, we propose to break this bottleneck by considering the cross-domain ldquotransfer of rank learningrdquo problem. Simultaneously, we propose a novel algorithm called TransRank, which can effectively utilize the labeled data from a source domain to enhance the learning of ranking function in the target domain. The proposed algorithm consists of three key steps. Firstly, we introduce a utility function to select the k-best queries from the source domain labeled data. Secondly, feature augmentation is performed on both source and target domain data, which can straightly adapt the ranking information from source domain to target domain. Finally, we utilize the classical ranking SVM to learn the enhanced ranking function on the augmented features. Experimental results on benchmark datasets well validate our proposed TransRank algorithm.
更多查看译文
关键词
novel algorithm,utility theory,labeled training data,source domain labeled data,learning (artificial intelligence),utility function,ranking function,transrank algorithm,source domain,ranking svm,target domain,target domain data,rank learning,proposed algorithm,feature augmentation,query selection,transfer rank learning technique,cross-domain transfer,proposed transrank algorithm,support vector machines,enhanced ranking function,query processing,target domain rank learning,training data,feature extraction,learning artificial intelligence,machine learning,classification algorithms,learning to rank
AI 理解论文
溯源树
样例
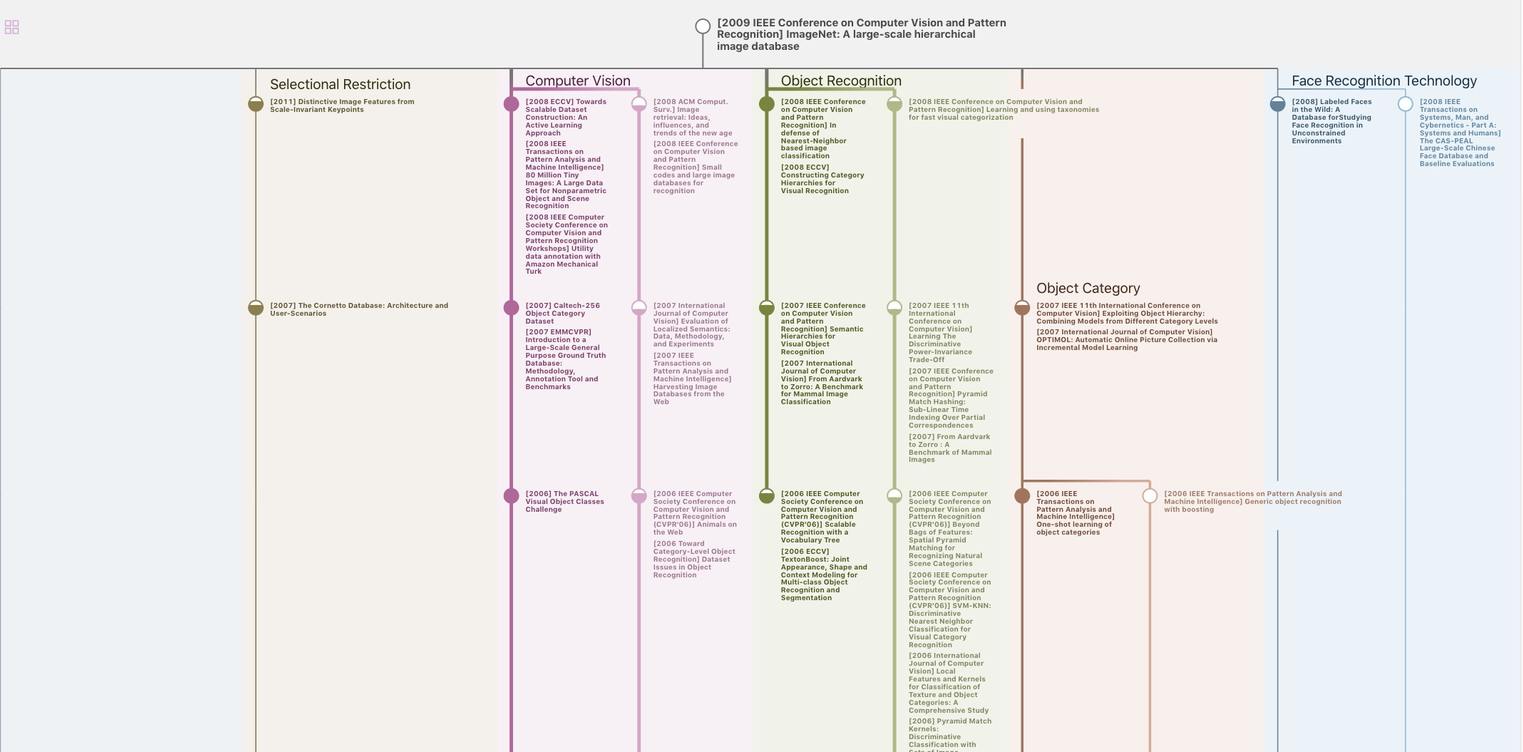
生成溯源树,研究论文发展脉络
Chat Paper
正在生成论文摘要