g-MARS: Protein Classification Using Gapped Markov Chains and Support Vector Machines
PATTERN RECOGNITION IN BIOINFORMATICS, PROCEEDINGS(2008)
摘要
Classifying protein sequences has important applications in areas such as disease diagnosis, treatment development and drug design. In this paper we present a highly accurate classifier called the g-MARS (gapped Markov Chain with Support Vector Machine) protein classifier. It models the structure of a protein sequence by measuring the transition probabilities between pairs of amino acids. This results in a Markov chain style model for each protein sequence. Then, to capture the similarity among non-exactly matching protein sequences, we show that this model can be generalized to incorporate gaps in the Markov chain. We perform a thorough experimental study and compare g-MARS to several other state-of-the-art protein classifiers. Overall, we demonstrate that g-MARS has superior accuracy and operates efficiently on a diverse range of protein families.
更多查看译文
关键词
support vector machines,gapped markov chains,markov chain style model,non-exactly matching protein sequence,protein classification,state-of-the-art protein classifier,accurate classifier,protein family,classifying protein sequence,gapped markov chain,protein classifier,protein sequence,markov chain,drug design,amino acid,transition probability,support vector machine
AI 理解论文
溯源树
样例
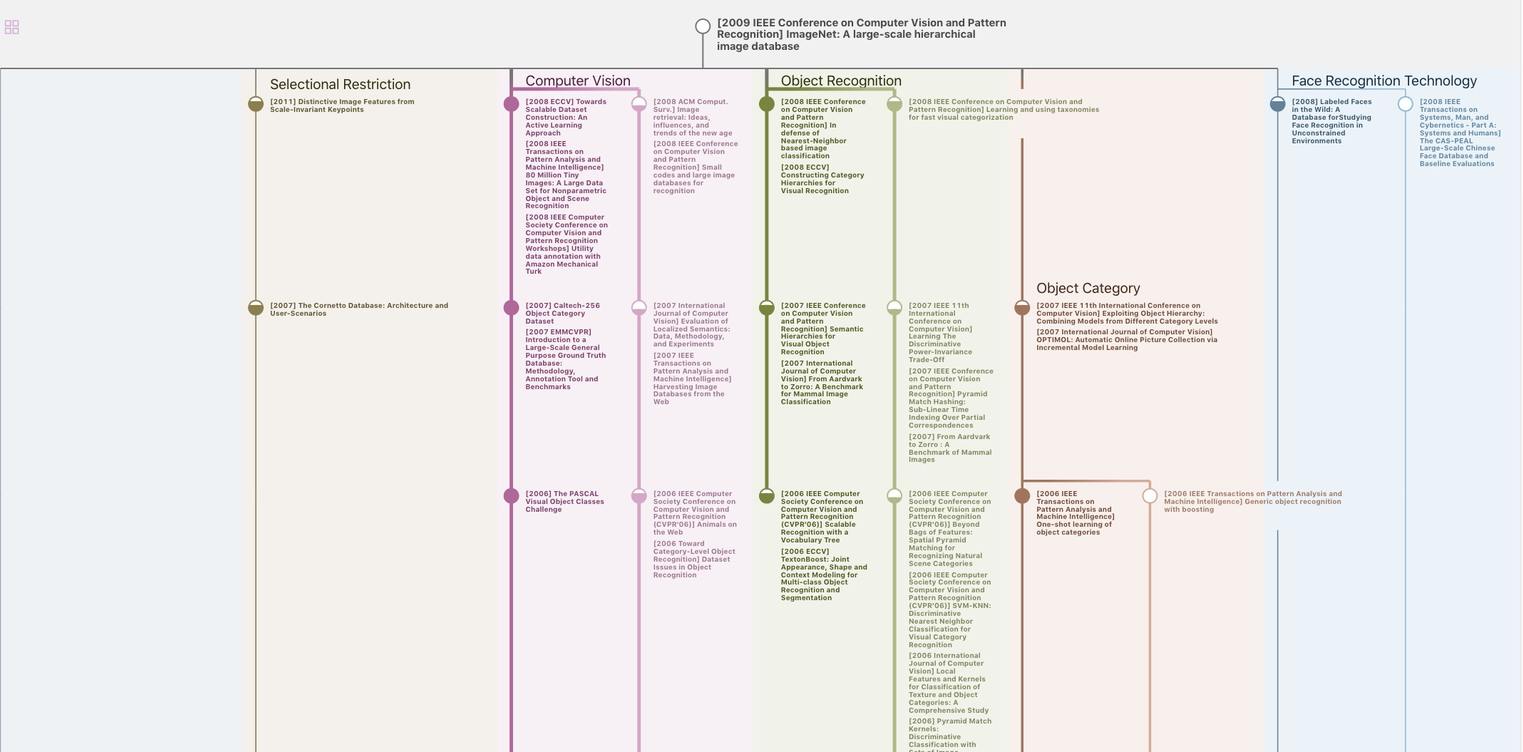
生成溯源树,研究论文发展脉络
Chat Paper
正在生成论文摘要